Using Voice Biometrics to Enhance N-of-1 Trials A 7-Step Guide for Recording Vocal Health Data
Using Voice Biometrics to Enhance N-of-1 Trials A 7-Step Guide for Recording Vocal Health Data - Voice Sample Collection Methods Using Modal and Head Voice Registers
When gathering voice samples for applications like voice cloning or podcast production, capturing a diverse range of vocal production is paramount. This includes using both the modal and head voice registers. The modal voice, our everyday speaking voice, provides a baseline, while the head voice, often used in singing, reveals different vocal qualities. By encompassing these registers, we get a more detailed picture of a person's vocal capabilities.
This comprehensive approach becomes even more valuable when combined with modern analysis techniques. Deep learning, for example, shows great promise in automatically analyzing the finer details of vocal recordings, potentially detecting subtle vocal changes associated with health or vocal strain. Traditional acoustic analysis also remains an important tool, especially when focused on stable sections of voice recordings. Properly choosing these stable sections is key to accurate vocal fold function assessment.
Ultimately, the combination of diverse recording techniques like modal and head voice captures, coupled with sophisticated analysis methods, offers improved insights into the nuances of human voice. These methods can provide a more precise personalized assessment in applications like vocal health monitoring within N-of-1 trials, or even in capturing unique aspects of someone's voice for cloning. However, there's an ongoing need to carefully balance the collection methods with the inherent complexity of voice analysis.
When collecting voice samples for applications like voice cloning or podcast production, understanding the differences between modal and head voice registers is crucial. Modal voice, our typical speaking and singing voice, uses a lower frequency range and a full closure of the vocal cords. Head voice, on the other hand, involves a blend of modal and falsetto qualities, resulting in higher frequencies and a thinner, more vibratile vocal fold edge.
This difference in frequency range – modal voice often falls between 85 Hz to 300 Hz while head voice can extend up to 2,000 Hz – can pose challenges for voice biometrics. The higher frequencies in head voice might not contain the same unique characteristics that are ideal for reliable voice identification.
Vocal training, particularly in head voice, can physically alter the vocal folds over time, potentially influencing the tone used for biometrics. This raises the question of how adaptable voice biometrics must be to accommodate long-term vocal changes. Meanwhile, the clarity and resonance emphasized in head voice can prove beneficial for applications demanding high intelligibility, such as audiobook production. This clarity makes voice recognition simpler in certain scenarios.
On the other hand, modal voice's richer sound can be susceptible to environmental noise, a factor that impacts the quality of recordings and could undermine the accuracy of biometric systems. Using either voice register extensively can lead to fatigue, affecting the consistency of pitch and sound quality in our recordings. This fatigue can introduce unexpected variation that we must be aware of while gathering voice samples, especially when doing long-term studies.
Moreover, the way different cultures emphasize vocal techniques, potentially favoring one register over the other, necessitates a flexible approach in voice biometrics. Algorithms need to adapt to varied vocal styles to accurately capture diverse populations.
When recording, especially head voice, the microphone's positioning is critical to avoid distortions. This is because the higher frequencies from head voice can easily distort with improper recording techniques. This is particularly important when seeking a good voice clone. The future of advanced AI-driven voice cloning depends on the careful representation of both modal and head voice to produce convincing synthetic voices. The subtle differences in pitch, tone, and resonance need to be perfectly balanced during training.
It's important to remember that biological variation plays a major role in how individuals produce sound. This inherent uniqueness emphasizes the importance of tailoring algorithms to personalize voice biometrics. This will ultimately improve the accuracy of systems designed to recognize and replicate the individual characteristics of a person's voice.
Using Voice Biometrics to Enhance N-of-1 Trials A 7-Step Guide for Recording Vocal Health Data - Creating a Controlled Recording Environment in Your Home Studio
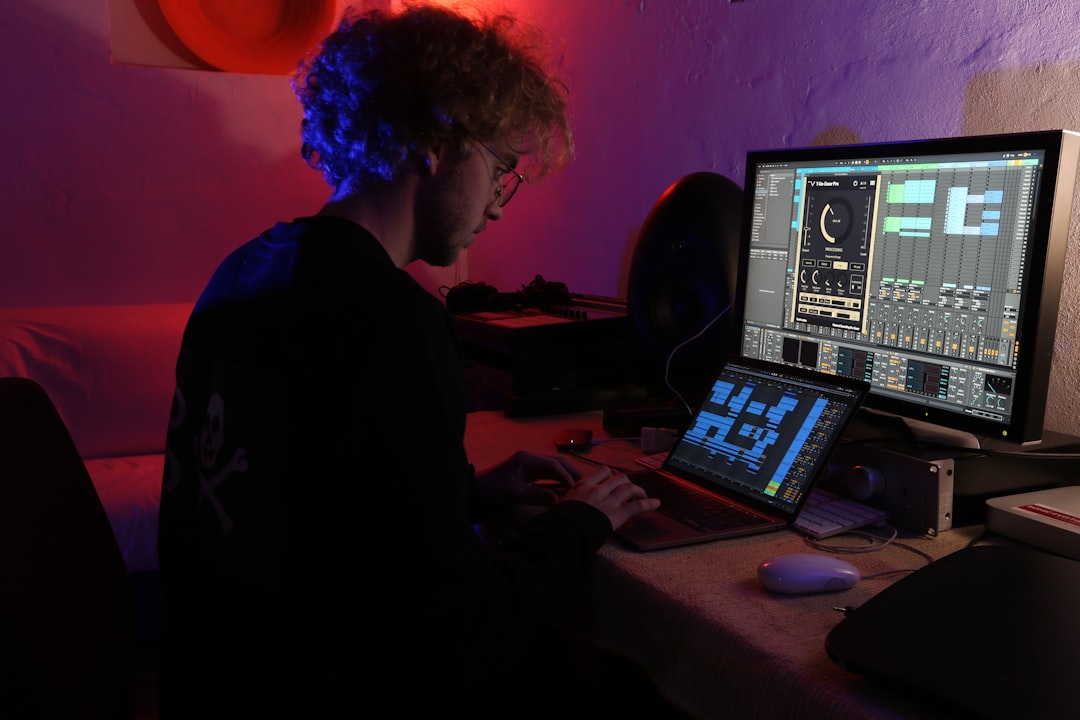
Creating a controlled recording environment within your home studio is crucial for achieving high-quality audio, especially when dealing with projects involving voice, such as podcasts, audiobook production, or even voice cloning. The foundation of a successful home studio involves thoughtful planning, including the layout of furniture and recording equipment. This layout will influence sound quality. You will need basic equipment, which includes a microphone, audio interface, and a digital audio workstation (DAW), but also consider a pop filter to minimize plosive sounds.
Beyond the gear, you must also treat your recording space acoustically to manage reflections and reduce undesirable echoes. This is important to achieve a controlled recording environment. Good monitoring tools, such as headphones or speakers, are essential. They let you hear what your recordings actually sound like. Also important are microphone selection and proper cable management. If you want high fidelity, the physical characteristics of your recording environment must be tailored for optimal audio capture. Aim to get the best results directly from the initial recording, minimizing heavy reliance on post-production sound editing.
The challenge is to create an acoustic environment that balances clarity with the ability to capture the nuances and features of the human voice. This is important whether you are trying to produce a consistent tone for a voice clone, or capture a range of vocals for speech biometrics. The recording environment can be a major factor in whether you are able to pick up all of the nuances of a speaker's voice. By focusing on the elements listed above, you'll be able to improve your recordings while gaining a better understanding of how to enhance voice-related applications such as cloning or biometrics.
To establish a controlled recording environment within a home studio, it's crucial to carefully consider the room's layout and the placement of recording equipment and furniture. This involves more than simply arranging things; it's about understanding how a room's physical attributes can impact the quality of a recording.
A basic setup typically includes a microphone, a pop filter to minimize plosive sounds, an audio interface to connect the microphone to a computer, a digital audio workstation (DAW) for recording and editing, and, of course, a quiet room dedicated to recording.
However, just having equipment isn't enough. Acoustic treatment plays a critical role in enhancing the audio quality. We can use various materials to manage unwanted reflections and echoes within the recording space. Different types of material are needed to optimize both higher and lower frequency sounds. Simply slapping up some foam panels might not be sufficient.
Essential tools for capturing high-quality recordings include headphones or speakers for audio monitoring. The quality of your microphone, its placement, and any preamps you are using also have a major impact on the recording's final sound.
Understanding how factors like the recording environment, microphone positioning, and preamp usage influence the overall sound quality is important. In particular, when we're dealing with human voice, it's important to minimize the influence of ambient sound.
Voice biometrics is finding increasing use in areas like N-of-1 trials because of the detailed analysis it provides of voice patterns. With a controlled environment, researchers can gather and analyze a wide array of data related to vocal health.
This involves understanding room acoustics, choosing the right software, and mastering effective microphone techniques. Tools such as Pro Tools or Adobe Audition can be helpful in enhancing the final result through the editing and mixing processes.
While post-production tools like those found in DAWs are helpful, it's beneficial to optimize the recording space to get the best sound quality at the source. This means working to mitigate room deficiencies to reduce the need for excessive processing.
Maintaining a recording space that minimizes extraneous noises and disturbances is vital. Such noises can interfere with the quality and clarity of the recording.
Minimizing the need to spend an excessive amount of time post-processing means a better understanding of acoustics and optimizing the recording environment. When recording voice, it's important to ensure the room temperature and humidity levels are stable as those aspects can influence how sound behaves. Additionally, flutter echoes—created by sound reflecting back and forth between parallel walls—can create a confusing aural experience and should be avoided.
The recording sample rate can impact the fidelity of recordings. For voice cloning applications, higher sample rates can provide a finer level of detail. Every room has specific frequencies at which it resonates and these can be manipulated to produce certain sonic characteristics. It's important to learn more about this and how it can influence a recording session.
Using Voice Biometrics to Enhance N-of-1 Trials A 7-Step Guide for Recording Vocal Health Data - Processing Raw Audio Files Through Voice Recognition Filters
Processing raw audio files, a necessary step in many voice-related applications like voice cloning and voice biometrics, involves using filters to improve the overall quality and reliability of the audio data. Essentially, this means cleaning up the recording by removing unwanted noise and other distortions, making the voice clearer and easier to process. This is important when trying to achieve accurate results in areas like assessing vocal health or when trying to clone someone's voice. Techniques such as noise reduction, filtering out unwanted frequencies, and standardizing the volume (normalization) are commonly used in audio preprocessing. These steps are critical for creating data that is suitable for machine learning algorithms used in tasks like analyzing voice features.
Recent advancements like the wav2vec 2.0 method have significantly enhanced the efficiency of these audio processing steps. The technology is making it faster and more effective to extract features that provide insights into vocal characteristics and possible changes in vocal health. Additionally, any preprocessing techniques must accommodate the diverse nature of human voice production, including differences between the modal and head voice registers, as discussed earlier in this guide. Voice biometrics relies on capturing distinct characteristics of the voice for accurate recognition, and the ability to correctly interpret both the modal and head voice registers will affect accuracy. As voice technology advances, increasingly complex preprocessing techniques are needed, as good initial audio quality is crucial for the ultimate success of a voice-related project. The link between recording quality and subsequent data analysis is only going to become more complex as voice cloning and voice health applications evolve.
When working with audio files for voice-related tasks like voice cloning or podcasting, we need to pay close attention to how we process the raw audio data. This processing can significantly affect the quality of the data used in applications that rely on voice characteristics, whether it be voice biometrics or even creating a convincing voice clone. One aspect is how the vocal folds—the structures in the larynx that produce sound—affect the voice. The elasticity and tension of these folds influence pitch and tone, creating a unique fingerprint for each individual. If we want to improve the accuracy of voice recognition, we need to learn more about how this elasticity varies from person to person.
Another challenge is latency in real-time voice recognition systems. These systems can introduce delays that can disrupt the flow of conversation, which can be problematic in applications that require quick and natural responses, like voice-controlled assistants or live podcasting. Reducing this lag is vital for a smooth user experience. Different microphones have different strengths and weaknesses, which is a factor we must consider. Their frequency response ranges impact how they capture different aspects of a person's voice, especially the contrast between the modal voice (our typical speaking voice) and head voice (a higher, often softer singing voice). Some mics might excel in capturing lower frequencies, while others perform better when capturing the higher frequencies common in head voice.
Breathy voices, characterized by a lot of air being emitted alongside the sound, also pose a challenge. Because of their inherent lower signal-to-noise ratio and the variability in how they are produced, they can lead to inaccuracies in voice recognition. This is especially true in noisy environments where a clear signal is crucial for proper analysis. Each individual’s vocal tract—the passage from the vocal folds to the lips—has a unique shape and size. This difference can significantly influence how sound waves resonate within the vocal tract and how they are ultimately perceived by a listener. Voice biometrics needs to account for these differences to function reliably.
Furthermore, our recording environment—especially a home studio—can have a significant impact on how a voice sounds. Different rooms will resonate at particular frequencies based on their dimensions and materials. Learning how to recognize and manipulate these resonant frequencies can improve the sound quality, which is especially important for things like voice cloning where we aim to produce realistic synthetic voices.
There are also aspects of human vocal development that are important to consider. Gender and age both influence vocal characteristics, leading to differences that can create challenges for voice biometrics systems. Systems need to account for these shifts and adapt over time to maintain their accuracy in voice identification. When capturing sound, we need to be mindful of plosive sounds, which are the sounds that arise from bursts of air when producing certain consonants like "p" or "b". These sounds can cause distortions in recordings, so employing techniques like pop filters and careful microphone positioning is vital to produce clear audio.
Beyond the purely technical challenges, how listeners perceive sound quality is also critical. Perception of a person's voice is not solely based on acoustics. Factors like familiarity and emotional context can also influence how a voice is perceived. This complexity can make developing consistently reliable voice biometric systems a difficult endeavor.
Finally, while post-processing of audio can enhance sound quality, overdoing it can create problems. Excessive modification can obscure the original vocal features essential for accurate voice recognition or biometrics. Achieving a good balance between enhancing the recording and preserving the underlying voice characteristics is important for obtaining reliable and accurate outcomes in applications like voice cloning, where we want a realistic copy of a person's voice.
The field of voice processing is constantly evolving, and researchers are continually looking for ways to improve the accuracy and robustness of voice recognition and voice biometrics. As we better understand the intricacies of human voice production, we can create more effective technologies that can adapt to these complexities.
Using Voice Biometrics to Enhance N-of-1 Trials A 7-Step Guide for Recording Vocal Health Data - Running Daily Voice Analysis Tests Using Pitch Detection Software
Regularly analyzing voice samples with pitch detection software is crucial for tracking vocal health, particularly within the context of voice biometrics and cloning projects. This method empowers the extraction of valuable vocal indicators, revealing both emotional states and potential physical changes. Sophisticated algorithms, often powered by Deep Neural Networks, significantly refine the accuracy of voice analysis, allowing for the identification of slight shifts in pitch and tone that might signal health concerns or vocal strain. The daily collection of data through these tests also provides a comprehensive record of an individual's vocal characteristics over time. This long-term data is invaluable in implementing personalized approaches, especially within the realm of N-of-1 trials. The continuous use of pitch detection software contributes to both the advancement of voice-related technologies and a more nuanced understanding of the multifaceted nature of human vocal production. However, keep in mind that solely relying on pitch as a measure for assessment might overlook other crucial features within a voice recording, requiring a more comprehensive approach that considers other factors. Moreover, the effectiveness of the analysis can be tied to the quality of the recording and may be affected by the type of voice (e.g., modal versus head voice) and environmental variables. The reliability of these automated systems will also vary, and it is still advisable to review any results using an experienced listener's ear.
Vocal fold characteristics play a key role in shaping the uniqueness of an individual's voice. The way these folds vibrate and interact with airflow determines the sound's fundamental aspects, creating a sort of 'vocal fingerprint.' This becomes increasingly important when trying to accurately clone a voice or perform voice recognition.
However, relying on these nuances for voice identification and cloning poses challenges. For example, pitch detection software, which is the cornerstone of many voice analysis systems, may struggle with the unique vocal qualities found in the head voice register compared to the more common modal voice. Head voice, often used in singing, produces higher frequencies and has a different vocal quality compared to the lower frequency modal voice. This can cause difficulties for algorithms originally designed to primarily handle lower frequencies.
Cultural aspects can also introduce challenges to standard voice biometric systems. Depending on a culture’s traditions and language, people tend to favor either the modal or head voice registers. These differences in vocal techniques, especially for speaking and singing, can pose an obstacle to universal applicability of biometric algorithms.
The pre-processing phase is important for enhancing the quality and reliability of voice recordings, particularly crucial in voice cloning and biometric assessments. Employing sophisticated noise reduction filters, like spectral subtraction, helps to improve signal clarity, so that the voice features are not masked by ambient noise.
Breathy voices, which have a relatively low signal-to-noise ratio due to the large amount of air emitted during vocalization, present a major problem for voice recognition systems, especially in environments that are noisy. This presents a challenge for applications that require fast and reliable voice processing, such as voice assistants.
The acoustics of a recording space influence how sound is captured and, consequently, affects the overall quality of recordings. Depending on the room size, dimensions, and materials, certain frequencies resonate more strongly than others, and this needs to be considered when building an optimal recording environment.
The fidelity of a recording is directly influenced by the recording's sample rate. Higher sampling rates provide greater detail within the audio data, which becomes especially useful when attempting to accurately replicate a voice for cloning or during vocal health monitoring when minute changes in voice need to be captured.
The human voice constantly changes. Vocal characteristics shift over time, influenced by age, health, and even hormonal changes. These shifts can cause issues for voice biometric systems, as they are not designed to account for changes over time, which can result in inaccurate results.
When dealing with real-time voice recognition, latency becomes a major concern. Any delays in a voice recognition system can hinder the natural flow of communication, which can be a nuisance in applications like live podcasting or voice assistants, where timely interaction is expected.
We also need to consider the impact of plosive sounds on the quality of a recording. Sounds like the "p" or "b" can cause distortion and degrade recordings, which makes the use of proper microphone placement and the use of accessories like pop filters crucial when trying to create high quality recordings for cloning or biometric analysis.
The field of voice processing is still evolving, and as researchers develop a better understanding of the complex interplay between acoustics, physiology, and human perception, voice recognition and voice biometrics will continue to improve and adapt to the ever-changing challenges.
Using Voice Biometrics to Enhance N-of-1 Trials A 7-Step Guide for Recording Vocal Health Data - Monitoring Voice Health Changes Through Spectral Analysis
Analyzing the spectrum of voice sounds has become increasingly important for understanding how our vocal characteristics connect to our overall health. This technique allows us to look at specific features in our voices, called vocal biomarkers, that can hint at various health conditions, from issues with the voice box to psychological problems. This approach helps with diagnosis and tracking patient progress, but also gives us a better way to tailor health assessments to each person, especially when considering N-of-1 trials where the focus is on individual health data. The technology behind voice analysis is constantly improving, with machine learning and artificial intelligence helping to increase the accuracy of health checks by picking up on even small changes in the way we speak. It's important to remember, however, that there are complexities involved in getting and understanding voice data. The way we speak varies widely between people and depends a lot on the situation. So, even as the field progresses, it's important to consider the limitations of voice analysis and how this influences the results we get.
1. Each person's voice is unique due to the way their vocal folds vibrate, creating a kind of vocal fingerprint. Voice biometrics and cloning rely on this uniqueness, but these features can change slightly because of things like vocal training or health issues. It's important to consider these variations when developing these systems.
2. Even when someone tries to produce the same sound repeatedly, their voice will naturally vary in pitch. This makes analyzing a voice using pitch detection software more complicated, as even small changes can signal a change in emotional state or even health. We need algorithms that are sensitive enough to identify these differences.
3. Many of the existing pitch detection algorithms used in voice analysis don't work well with higher-pitched or "head" voices (often used in singing) when compared to the more common "modal" or speaking voice. This difference makes it challenging to reliably use biometrics with all types of voices, especially if the system was built primarily for lower-frequency speech.
4. The room you record in can have a significant impact on how the sound is captured. Certain rooms will reflect some sound frequencies more than others, depending on their size and shape. This can lead to some sound frequencies becoming more prominent or muted, potentially interfering with our efforts to use voice analysis in applications like cloning or biometrics.
5. Environmental conditions like humidity and temperature can alter the way our vocal folds function, causing changes in our vocal tone. These variations in vocal quality can potentially impact the trustworthiness of any data collected for voice biometrics, especially if those conditions aren't carefully considered during recording.
6. The elasticity of our vocal folds is a key factor in determining the pitch and tone of our voice. When these vocal folds stretch or become less elastic, there are noticeable shifts in pitch, which need to be tracked accurately for monitoring and analysis using voice biometrics.
7. Different cultures have different ways of using their voices, particularly when it comes to singing and speaking. Some may rely more on head voice, while others use modal voice more often. This means it's a challenge to build a biometric system that works equally well for all people, as the systems need to adapt to these diverse ways of communicating.
8. In real-time voice recognition systems, delays (or latency) can create a disruptive experience, especially during interactive applications like live podcasting or conversations with voice assistants. Keeping the latency as low as possible is important for a smooth experience.
9. Sounds like "p" and "b" (plosive sounds) can create distortion in a recording. To reduce this, we need to be mindful of how we use microphones and consider using things like pop filters, which can make a significant difference in the quality of voice recordings for cloning and biometric analysis.
10. Voices that are described as breathy have a lower ratio of signal to noise, mainly because there's a large amount of air escaping when they speak. This can create issues with voice recognition in noisy environments, making it harder to isolate the voice for analysis.
Using Voice Biometrics to Enhance N-of-1 Trials A 7-Step Guide for Recording Vocal Health Data - Building a Personal Voice Pattern Database
Building a personal voice pattern database involves systematically collecting and organizing a person's unique vocal characteristics. This database becomes a valuable resource for applications like voice cloning or tracking changes in vocal health over time, which is increasingly important in personalized medicine. To construct a truly representative database, you need to record and analyze a diverse range of vocal productions, covering both the typical speaking voice (modal voice) and the higher-pitched, often softer singing voice (head voice). This variety helps to capture the full spectrum of an individual's vocal abilities and creates a more accurate representation of their voice.
However, the creation and use of these databases also present challenges. Human voices are incredibly complex, and they are susceptible to changes based on factors like health, training, and even the recording environment. This means we need algorithms that can flexibly adapt to these variances to avoid inaccurate analysis. Similarly, there can be differences in how different cultures emphasize vocal qualities, so we need to be sensitive to this in the design of voice biometric systems.
The ongoing development of voice technologies, particularly in areas like voice cloning and personalized healthcare, demands the creation of well-structured voice pattern databases. These databases serve as the foundation for building systems that can accurately identify and analyze voice characteristics, recognizing the range of sounds a person can produce. As these technologies become more sophisticated, ensuring the voice databases are constantly updated and able to adapt to the subtle differences in human voices will be critical for ensuring the technology delivers on its promise.
Building a robust personal voice pattern database is a fascinating challenge, especially in the context of voice biometrics and its applications in voice cloning and podcasting. There are several factors that contribute to this complexity and require careful consideration for achieving accurate and reliable results.
First, the dynamics of our vocal folds, the structures that produce sound in our larynx, are constantly in flux due to factors such as health, hydration, and even our daily routines. This inherent variability in vocal fold elasticity and tension can shift pitch and tone throughout the day, making it crucial for voice biometrics systems to be adaptable and adjust to these changes over time.
Second, human voices are influenced by both gender and age. As we grow and age, our vocal characteristics change significantly, due to hormonal changes and the natural aging process. This presents a challenge for systems meant to recognize voices long-term, highlighting the need for continuous model updates to ensure accurate voice recognition.
Third, the way we use our voices is highly dependent on cultural norms and traditions. Different cultures may emphasize different vocal registers, such as modal voice (our normal speaking voice) and head voice (a higher-pitched, often softer, singing voice). This variance in vocal technique can make it challenging to design universally applicable biometric algorithms, potentially requiring customization for certain vocal patterns and styles.
Fourth, the quality of a voice recording is strongly affected by the specific characteristics of the microphone in use. Certain microphones might be better suited for recording lower frequency sounds, while others might perform better at recording higher frequencies. This means that if you are trying to produce a reliable voice clone, for example, you might have to consider these factors during the recording process.
Fifth, the acoustics of a recording space have a large influence on the frequencies of sound captured. Every room has different dimensions and is made of different materials, which means that certain frequencies will resonate more than others within that particular room. This can create challenges for biometric systems that rely on consistent vocal characteristics.
Sixth, breathy voices, a vocal quality where a large amount of air escapes during sound production, pose a specific problem for voice recognition algorithms. Breathy voices generally have a low signal-to-noise ratio, meaning that there is a lot of background noise compared to the actual voice. This can be exacerbated when recording in noisy environments and can lead to inaccurate recognition.
Seventh, environmental conditions, such as humidity and temperature, play a significant role in vocal fold function and the quality of the recording. Small shifts in the environment can lead to subtle changes in vocal tone that might impact the accuracy of voice biometric systems that rely on stable, unchanging characteristics of an individual's voice.
Eighth, latency, which is essentially the delay time between producing a sound and a system recognizing it, can be a major issue for voice-based applications that require fast response times, such as voice-activated assistants or live podcasting. Minimizing latency is crucial for a smooth and natural interactive experience.
Ninth, vocal training, especially in singing, can result in significant physical changes to the vocal folds. This alteration of vocal production, particularly when emphasizing the head voice register, can affect the accuracy of systems that rely on a stable, consistent voice signature for identification.
Tenth, while post-processing can enhance the quality of audio recordings, excessive editing can mask crucial vocal characteristics needed for precise voice recognition or cloning. This calls for a careful balance between improving the recording and retaining the original voice features to ensure reliable results.
The human voice is truly a complex and intricate instrument, influenced by a wide range of factors. As voice biometric technologies continue to develop, researchers are working to refine algorithms and techniques to account for the ever-present nuances of human vocal production, striving for greater accuracy and reliability in applications such as voice cloning and vocal health monitoring.
Using Voice Biometrics to Enhance N-of-1 Trials A 7-Step Guide for Recording Vocal Health Data - Weekly Voice Data Reports Using Frequency Response Charts
Weekly voice data reports incorporating frequency response charts offer a new way to analyze voice data, particularly when it comes to vocal health and performance. These charts visually depict how various frequencies are represented during recording sessions, which can be very insightful when evaluating vocal abilities. Using these charts, individuals can potentially detect vocal strain, track changes in health, or even gain a better understanding of emotional states through their voice. This detailed data is especially relevant to personalized applications like voice cloning, where a precise representation of a person's voice is essential, and for audio projects, such as podcasting or audiobook production, where the quality of the recording is important.
However, the value of frequency response charts is not without its limitations. One area of concern arises from the differences between vocal registers—modal and head voice, for instance—and how these impact the frequency response. This can cause challenges when trying to use the charts to support specific applications like N-of-1 trials or projects focusing on a particular aspect of a person's voice. As a result, it is critical to thoughtfully interpret the information shown in the charts and understand the limitations of this approach, to avoid misinterpretations that could negatively affect the goal of a specific project.
Weekly voice data reports, visualized using frequency response charts, can offer a dynamic view of an individual's vocal landscape. These charts essentially map the various frequencies that make up someone's unique vocal signature, highlighting how they shift over time. This can be useful in detecting subtle changes that might indicate health issues, whether related to vocal strain, illness, or the natural aging process.
However, the relationship between speech and singing frequencies poses some interesting challenges. Our everyday speaking voice, often called the modal voice, typically sits within a frequency range of about 85 Hz to 300 Hz. On the other hand, singing, especially when exploring higher registers like head voice, can extend much higher, sometimes reaching frequencies beyond 2000 Hz. This stark difference has implications for how we design voice biometrics systems. Algorithms must be flexible enough to capture these different vocal registers and differentiate between them accurately.
Recording environments introduce another layer of complexity. The acoustics of a room, the way it reflects and absorbs sound, can significantly distort the frequency response of voice recordings. These distortions can essentially mask crucial vocal details that are needed for accurate biometric analyses. Optimizing recording spaces to minimize these issues is essential.
Furthermore, we must remember that human perception of voice is a multifaceted process. Our minds don't solely focus on the raw acoustic features of a voice. Background noise, emotional nuances in speech, and even our familiarity with the speaker can all influence how we interpret the sound. This can pose challenges for fully automated voice recognition systems, highlighting the need for approaches that combine algorithmic analysis with human evaluations.
The dynamics of our vocal folds, the structures that create sound in the larynx, introduce another variable to consider. These folds can shift in elasticity and tension due to various factors. A well-trained singer, for example, might exhibit a different range of vocal behavior compared to someone who's never sung before. This variability can make it difficult to establish consistent vocal baselines for voice biometric applications and underscores the need for systems with adaptability.
Voices with a breathy quality introduce a distinct set of difficulties for voice recognition systems. This kind of vocal production tends to be characterized by a relatively low signal-to-noise ratio. This means that the actual voice signal is masked by a larger amount of noise (think of the sound of air rushing during speech). Isolating the voice from this noise is a significant challenge, particularly when trying to extract specific vocal features for tasks like voice cloning.
Cultural variations in vocal production further complicate the picture. Different cultures may emphasize particular vocal registers more than others, favoring modal voice or head voice depending on communication styles. These diverse vocal techniques impact the adaptability of voice biometric algorithms, highlighting the need for flexible, potentially culturally sensitive, models.
Real-time processing of voice data, especially in interactive settings, can introduce another challenge: latency. In other words, the time lag between speaking and a system responding. Any delay, even a fraction of a second, can disrupt the natural flow of communication, which can be problematic in applications like voice assistants and live podcasting where immediacy and responsiveness are essential.
Vocal training, especially in singing, can produce significant physical changes in vocal folds over time. This raises questions about the adaptability of voice biometrics to recognize individuals who have undergone extensive vocal training. The system needs to recognize both trained and untrained individuals reliably.
Finally, the potential pitfalls of audio post-processing are worth noting. While we can enhance recordings through editing, excessive modifications can remove important subtle variations needed for reliable voice recognition or cloning. The key is to find a balance between refining a recording and preserving the intrinsic characteristics of the voice itself.
The journey of understanding the human voice, with all its intricacies, is an ongoing one. As we continue to develop voice technologies and applications, particularly in the domain of personalized voice cloning and health monitoring, we need to navigate these complexities carefully. This means constantly refining our approaches and developing more adaptable algorithms that can cope with the inherent variability and fascinating nature of human vocal production.
More Posts from clonemyvoice.io: