Get amazing AI audio voiceovers made for long-form content such as podcasts, presentations and social media. (Get started for free)
Troubleshooting Voice Cloning 7 Common Issues and Their Solutions
Troubleshooting Voice Cloning 7 Common Issues and Their Solutions - Accent Inconsistencies in Synthesized Speech
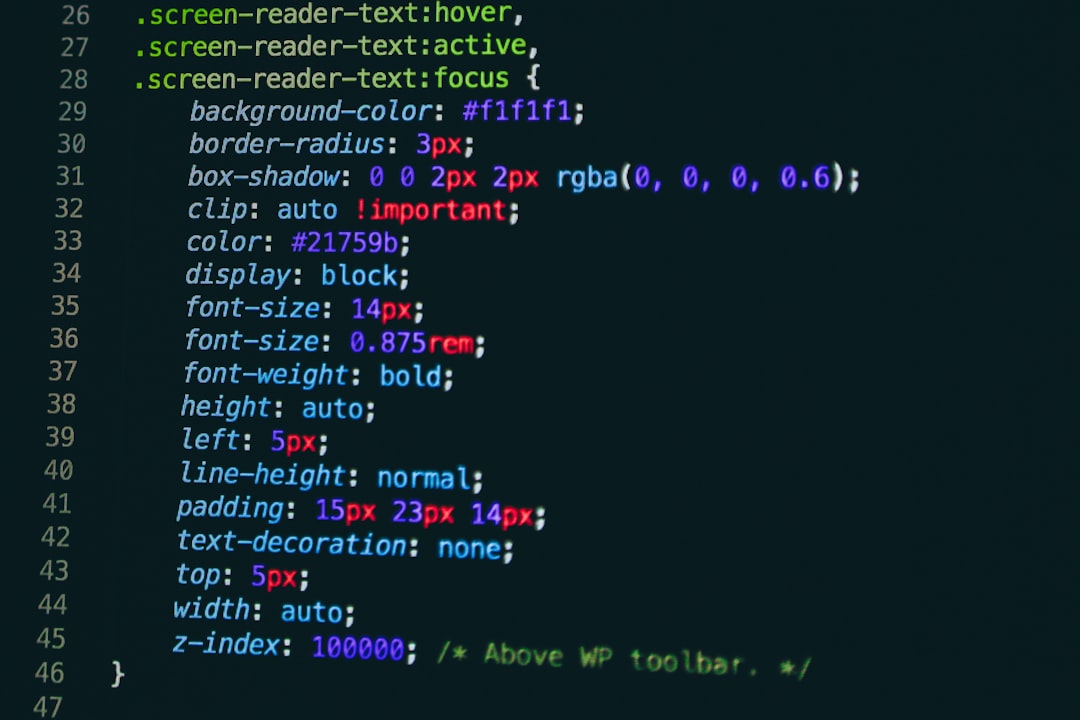
Achieving consistent accents in synthetic speech remains a hurdle in voice cloning and text-to-speech applications. The process of accurately replicating the intricate nuances and patterns of a specific accent can be quite challenging. This can result in noticeable deviations from the desired accent, making the generated voice sound unnatural or even jarring.
The quest for authentic synthetic speech hinges on effectively capturing the nuances of diverse accents. This requires careful training of the voice cloning models with varied and representative audio data. When the training data doesn't sufficiently reflect the desired accent, the resulting synthetic speech will likely struggle to maintain accuracy and consistency. The impact of this can be particularly detrimental in situations where realism is paramount, such as audiobooks and podcasts, where listeners might find the inconsistencies disruptive.
The field of artificial intelligence and natural language processing is continually evolving, and a strong focus on understanding and refining accent replication within synthetic speech is needed. This will help ensure that the voices we create through these technologies increasingly reflect a greater level of authenticity, leading to a more satisfying user experience.
Synthesized speech can sometimes exhibit inconsistencies in accent reproduction, often stemming from the limitations of the training data. If a model primarily learns from a single accent, it might struggle to accurately replicate or smoothly transition between different accents, leading to noticeable shifts in pronunciation or intonation.
The intricacies of accent variations, including subtle changes in pitch, rhythm, and intonation, pose a challenge for synthetic voice generation. These differences are often difficult for voice cloning models to fully capture, resulting in a robotic or unnatural quality when attempting to emulate non-standard dialects.
Voice cloning algorithms may misinterpret regional pronunciation nuances, making it difficult to maintain a consistent accent throughout longer audio outputs. This inconsistency is particularly noticeable during dynamic changes in context or emotional expression where the model's ability to adapt its accent appropriately may falter.
Many voice cloning methods rely on unit selection synthesis, which involves stitching together pre-recorded speech fragments. If these fragments do not represent the full range of an accent's phonetic features, the generated speech might sound inconsistent, even within a single sentence.
A phenomenon called interpolation in speech synthesis can also contribute to accent inconsistencies. During interpolation, the system attempts to blend sounds from different accents, sometimes resulting in an unintended oscillation between multiple accents within the output speech.
The prosodic features of language, such as rhythm and intonation, vary considerably between different accents. Most current voice cloning technologies struggle to replicate these subtle nuances, leading to a relatively flat or inconsistent delivery when attempting to clone a voice from a specific region.
Accents influence not just pronunciation but also the subtle emotional undertones of speech, which are frequently lost in synthetic voices. This loss of emotional nuance can lead to a less authentic, more mechanical sound compared to natural speech, where emotional cues and regional identity are often intertwined.
In multilingual models, accent inconsistencies can arise from the mixing of linguistic features. If not carefully calibrated during training, the synthesis process across different languages might distort the intended accent representation.
Research suggests that human listeners are very sensitive to accent inconsistencies, often perceiving them as a lack of authenticity or professionalism, which can detract from the overall quality of applications like audiobooks and podcasts.
While neural speech synthesis models are making progress in mitigating accent inconsistencies, many systems still require manual adjustments to parameters like tone, speed, and emotional cues. This highlights the significant gap that remains between human-like speech generation and the current capabilities of machine-based voice cloning.
Troubleshooting Voice Cloning 7 Common Issues and Their Solutions - Unnatural Pauses and Rhythm in Audio Output
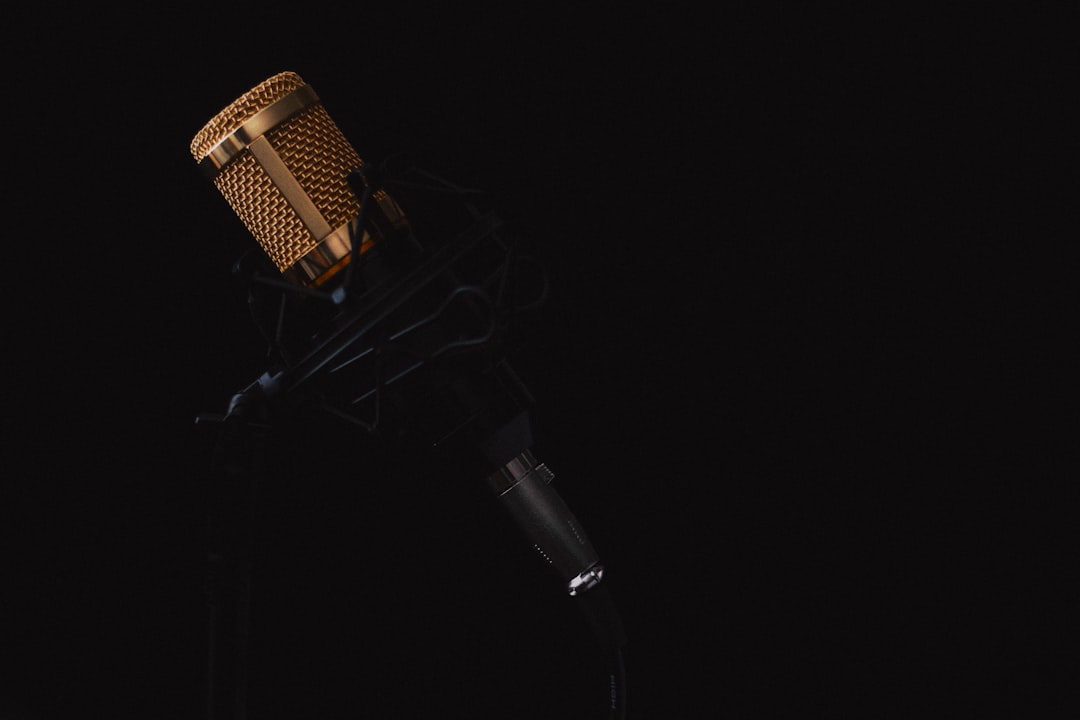
Unnatural Pauses and Rhythm in Audio Output
A frequent issue in voice cloning and text-to-speech technologies is the creation of unnatural pauses and rhythms within the audio output. This can significantly impact the listener's experience, making the synthesized speech sound robotic or disjointed. The problem often stems from a difficulty in replicating the natural flow and intonation of human speech, a facet of language known as prosody. The resulting audio can feel jarring due to awkward pauses or a lack of dynamic rhythm, ultimately diminishing the overall quality and engagement of the generated voice.
One approach to improving this aspect of synthetic speech is the use of voice activation detection (VAD). This method aims to fine-tune the audio output by minimizing unnatural silences and smoothing the transitions between words or phrases. Furthermore, some newer techniques that analyze audio in its raw waveform format (instead of relying on traditional methods of interpreting features in the audio) show promise in refining the naturalness of the voice, potentially eliminating the need for many of the typical workarounds used to achieve acceptable results. The development of truly lifelike synthetic voices hinges on overcoming these challenges, especially when considering applications such as audiobooks or podcasts, where an immersive and natural listening experience is essential.
### Unnatural Pauses and Rhythm in Audio Output
Even subtle, unnatural pauses in audio output can significantly impact the listening experience. Research indicates that a pause as short as 200 milliseconds can feel jarring, making it harder for listeners to follow along. Proper rhythm and timing are crucial for natural-sounding speech. In audio production, anything longer than about 500 milliseconds of silence can be interpreted as a stop or a break rather than a smooth transition, creating a disjointed feeling.
Human speech features a natural variation in pace and rhythm that contributes to emphasis and engagement. However, voice cloning systems often stick to a fixed speech rate, resulting in a monotonous delivery and noticeable inconsistencies. This artificiality stands in stark contrast to how humans naturally vary their speech rhythm.
Accurate representation of speech features like pitch contour and emphasis, collectively known as prosody, is a major challenge for voice cloning. The failure to capture this dynamism in synthetic speech often leaves it sounding robotic and unnatural, lacking the expressiveness of human communication.
The presence of background noise can affect how humans perceive pauses and rhythm. We naturally adapt our speech to accommodate surrounding sounds. However, synthetic voices often lack this adaptability, making them sound out of sync in noisy settings. This can be a major issue for scenarios like podcasts, which are often recorded in environments with some ambient sounds.
Effective communication relies heavily on the use of context-specific pauses. Speakers naturally adjust their pacing based on the thematic flow of conversation. Voice cloning technology struggles with this nuanced application of pauses, resulting in output that feels rhythmically disjointed from the intended message.
Another area of concern is how the clustering of phoneme sounds, the basic units of speech, is handled in synthesis. Inconsistent phoneme clustering can cause abrupt shifts in rhythm, leading to a stilted or fragmented audio experience.
Different languages and dialects exhibit unique syllable timing patterns, adding another layer of complexity to natural speech. Many voice cloning methods struggle to accurately replicate these intricacies, which creates a dissonance for native speakers.
Human speech uses timing and pauses to reflect emotional states, creating powerful effects like emphasizing tension with dramatic pauses. Most voice cloning tools cannot convincingly convey emotional context through pausing, leading to a rather mechanical-sounding output, even when the topic is emotionally charged.
While some advanced voice synthesis systems are incorporating adaptive learning methods to try to improve pause and rhythm placement, they face considerable hurdles. Effectively training these models requires massive datasets and the technology still struggles to generalize rhythm across diverse speaking styles and contexts. These are areas that still require more research.
In the pursuit of more natural-sounding synthetic speech, ongoing research and development are focused on tackling these issues. We need more sophisticated methods that can more accurately replicate the rhythmic complexities found in natural speech.
Troubleshooting Voice Cloning 7 Common Issues and Their Solutions - Emotional Tone Mismatches in Cloned Voices
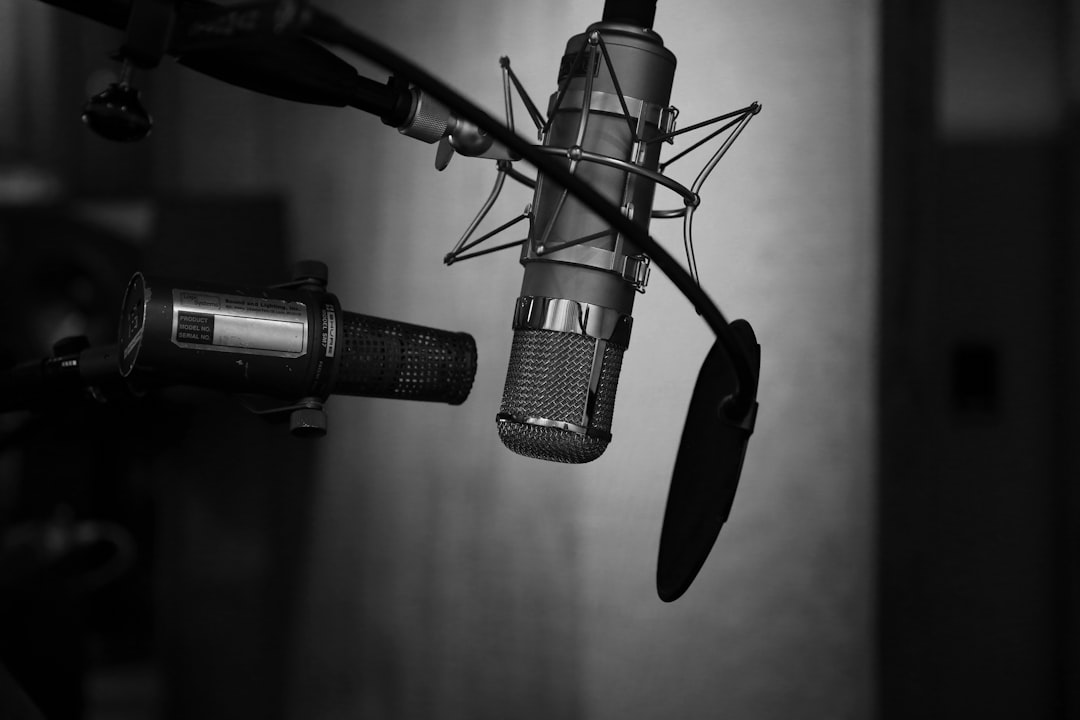
When attempting to replicate a person's voice, one of the most difficult aspects to capture accurately is the emotional tone conveyed through speech. While voice cloning technology can mimic a variety of emotional states, it often falls short of producing the subtle and nuanced delivery we find in human voices. This can result in a synthesized voice that sounds flat or even robotic, detracting from the desired impact.
The quality of the original audio used for cloning significantly impacts the emotional tone of the resulting voice. If the input audio is of poor quality or doesn't capture the desired emotional range, the synthesized voice may lack emotional depth or exhibit unnatural variations in tone. Furthermore, the ability to adjust the emotional characteristics of a cloned voice after its creation is very limited. This means that careful selection of the original audio sample(s) is essential for producing a voice with the desired emotional attributes.
The field of voice cloning is constantly evolving, and researchers are actively working to develop more sophisticated techniques for accurately reproducing emotional tones in synthetic speech. However, bridging the gap between the natural expressiveness of human voices and the current capabilities of voice cloning technology is still a major challenge. This is especially important in applications such as audiobook creation and podcasting where a voice that can connect with the listener on an emotional level is essential. The future of truly engaging synthetic voices likely relies on overcoming this limitation and developing methods that can authentically and accurately capture the emotional nuances of human speech.
Replicating the emotional tone of a voice during cloning remains a challenge. While voice cloning technology can mimic different vocal patterns, accurately conveying emotion often falls short, leading to outputs that sound monotonous. This is because emotional expression is deeply intertwined with subtle changes in pitch, rhythm, and the overall tonal quality of speech—characteristics that are not always effectively captured by current voice cloning algorithms.
The mismatch between intended and synthesized emotion can negatively impact listeners. It can create a disconnect, causing confusion and reducing engagement. This is particularly important for media like audiobooks or podcasts where a natural and expressive voice is crucial for the listener's experience.
Even subtle variations in how phonemes are produced can reflect different emotions. Joy might increase pitch, while sadness can lower it, making it difficult for voice clones to accurately capture the desired emotional nuance. Furthermore, the dynamics of speech delivery—known as prosody—which involves variations in pitch, speed, and emphasis, are often not effectively replicated. Excitement might be conveyed by faster speech and pitch variations, while sadness often leads to a slower, flatter delivery. If these dynamic aspects aren't properly considered, the cloned voice often sounds flat and unnatural.
We as listeners also have expectations about the emotional tone of a voice based on the content or context. When a synthesized voice doesn't meet these expectations, the overall quality and authenticity of the audio can be undermined, leading to a potentially negative impression on the listener.
The training data used to build a voice clone has a substantial impact on its ability to replicate emotional tone. Models trained on limited data, or data that lacks diverse emotional expressions, may struggle to generate a convincing emotional response. This can be especially noticeable in more nuanced narratives, where a wide range of emotions is necessary to create a compelling experience.
Many voice cloning algorithms rely on statistical methods that prioritize clear articulation over emotional expressiveness. This can lead to synthetic voices that sound clear but lack the richness of human emotion that is so important for impactful communication.
When trying to represent complex emotional contexts, like a funny anecdote with a serious undertone, many cloning models struggle. The output might either oversimplify the emotional content, resulting in a flat or even caricatured expression that fails to resonate with listeners.
Additionally, feedback loops in automated systems can inadvertently reinforce flaws. For instance, if a voice clone frequently generates an emotion incorrectly, users might provide feedback that encourages the model to further emphasize that specific error, leading to a persistent issue within the cloned voice.
Finally, cultural differences play a significant role in emotional expression in speech. Speech patterns, intonation, and rhythm vary greatly across cultures, and voice cloning technology may not adequately account for these variations, potentially leading to outputs that are unfamiliar or even jarring to international audiences.
These challenges highlight the need for continued development in voice cloning technology, focusing specifically on improving the ability of these systems to replicate the nuances of human emotion in speech. The ability to produce truly lifelike and emotionally expressive synthetic voices is still a significant goal for researchers and engineers in the field.
Troubleshooting Voice Cloning 7 Common Issues and Their Solutions - Background Noise Interference in Voice Samples
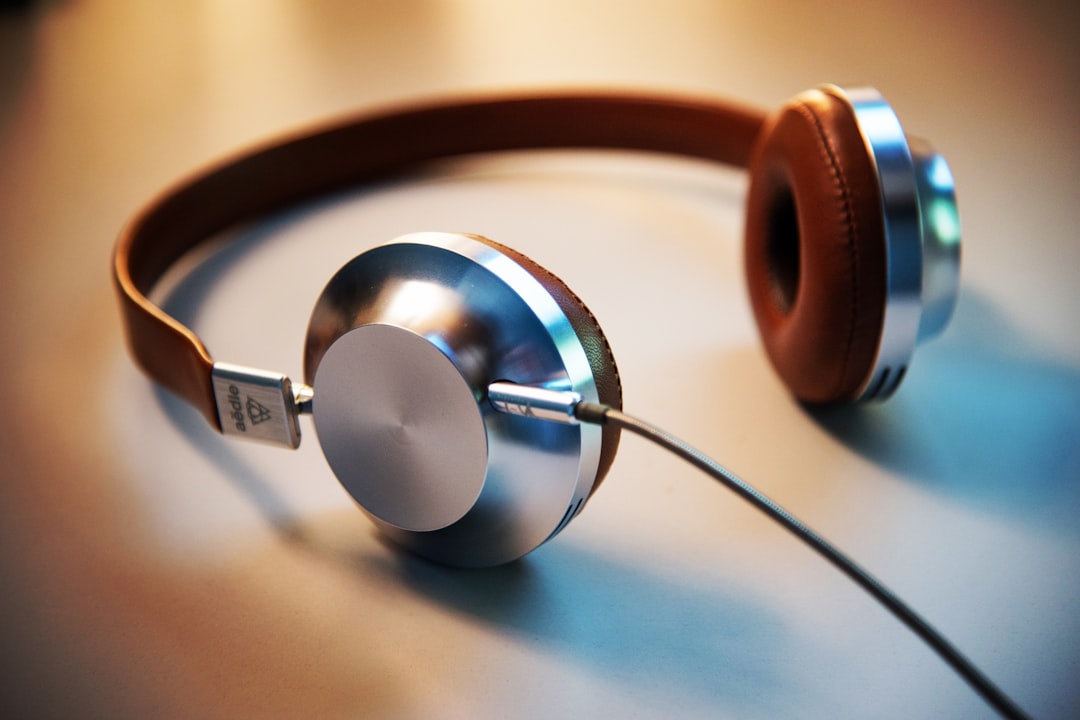
Background noise can significantly impact the quality of voice samples used in voice cloning. Unwanted sounds, whether it's traffic, conversations, or equipment hum, can interfere with the clarity of the speaker's voice, making it difficult for the cloning process to accurately capture the desired vocal characteristics. This can lead to a synthesized voice that sounds muddled, unnatural, or even distorted.
Creating a controlled recording environment is crucial to minimize this issue. The ideal recording space should be relatively quiet and free from distractions that could interfere with the audio. This allows for a clearer capture of the speaker's voice, which is especially important when the goal is to replicate a specific voice with accuracy.
Furthermore, the selection of audio samples for the cloning process is essential. Opting for samples that feature a single speaker and are free from background noise contributes significantly to the success of the cloning process. This minimizes the chances of the algorithm incorporating unwanted sounds into the synthesized voice, resulting in a more authentic and high-quality clone.
While current voice cloning technology is steadily improving, noise reduction and filtration remain important areas of development. As technology advances, more sophisticated noise-cancellation techniques will likely play a greater role in refining voice clones. This is especially important for applications where achieving high-fidelity audio is critical, such as audiobooks and podcast production. The goal is to deliver a smoother and more natural listening experience, eliminating distractions caused by background noise and ultimately improving the overall quality of voice cloning output.
### Background Noise Interference in Voice Samples: A Closer Look
Background noise poses a significant challenge for achieving high-quality voice cloning. Even subtle noise can impact the clarity of audio samples, leading to issues with the final synthesized voice. It's fascinating how sensitive these voice recognition algorithms are; even a 3-decibel change in ambient noise can make a noticeable difference in how well the system understands the spoken words.
One reason for this sensitivity is the overlap between common noise frequencies and the frequencies of human speech. Think about a typical café—it tends to have a lot of noise in the mid-range, the same area where much of human speech falls. This makes isolating the voice signal from the noise a difficult computational task.
Another interesting phenomenon is called temporal masking, where the presence of noise right before or after a sound event affects how the voice is perceived. Voice cloning systems attempt to understand the timing of speech components, but noise can disrupt this process, making the generated voice sound unnatural or off-sync.
While many noise reduction techniques exist, they can sometimes create more problems than they solve. Some algorithms use aggressive filtering that ends up altering the voice itself, introducing artifacts that can sound robotic. Furthermore, while advanced cloning systems use adaptive learning to deal with noise, they often struggle with highly variable or unexpected sounds. This leads to inconsistencies in voice quality.
It's worth mentioning the impact of how audio is stored and compressed. Compressing audio into formats like MP3 often leads to a loss of fine details in the sound, and this can negatively affect the cloning process, particularly in the presence of noise.
Noise even interferes with how emotion is detected in the speech. Things like laughter or hesitation can become less discernible, which makes it difficult to accurately convey emotion in the cloned voice. And if a person has to listen to synthesized voice in a noisy place for a long period of time, they may end up feeling tired from having to focus too hard to hear it. This highlights the importance of the quality of audio recording when cloning voices for uses like audiobooks or podcasts.
To combat this issue, some researchers use speech enhancement techniques like beamforming. This approach tries to focus on particular sound sources in a noisy environment to isolate the voice more effectively. However, it's a delicate balancing act because poorly implemented beamforming can introduce distortions.
Interestingly, different cultures have different levels of tolerance and reaction to background noise. This has to be kept in mind when developing voice cloning systems to make sure that the generated voices are well-received by listeners worldwide. The challenge of producing a seamless cloning experience across all cultures and environments remains a fascinating area of research.
Troubleshooting Voice Cloning 7 Common Issues and Their Solutions - Limited Vocabulary in Trained Voice Models
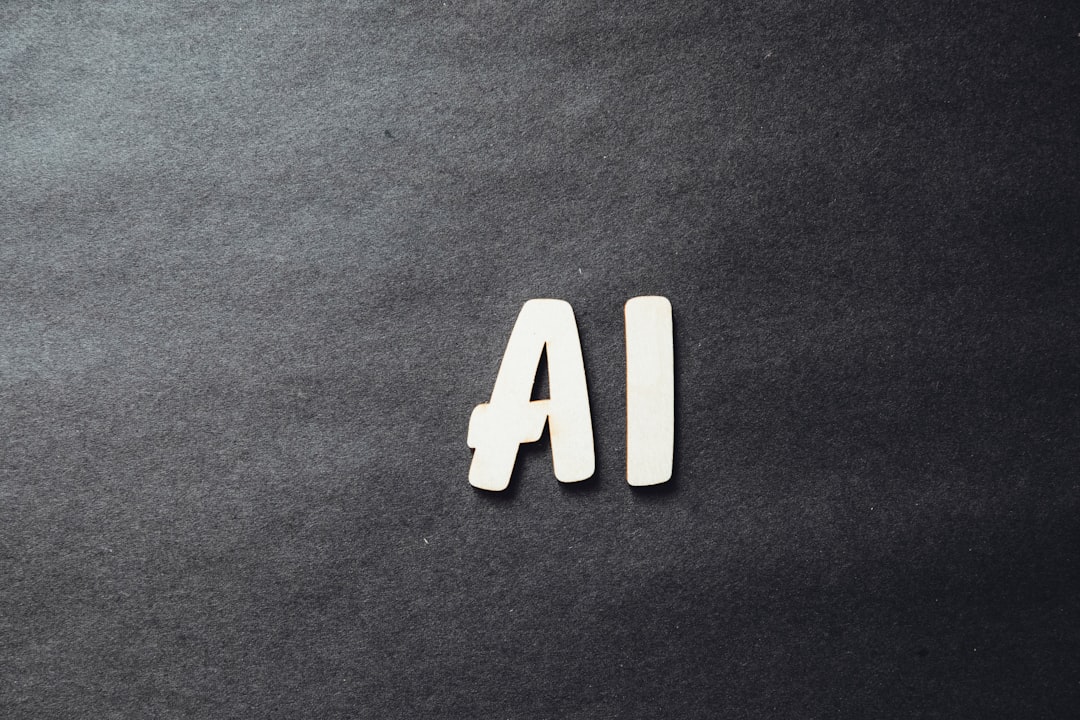
When training voice models, a limited vocabulary can result in synthesized speech that, while potentially sounding like the original speaker, may be difficult to understand. Voice cloning models often face challenges accurately pronouncing words, particularly when the training data is small or lacks diversity. While newer voice cloning methods, like those using generative models, show promise in producing high-quality cloned voices, they rely heavily on the quality and breadth of the training data. Ensuring that the data used to train the models covers a wide range of vocabulary is vital for achieving natural-sounding speech that can handle diverse linguistic structures. Preparing and refining the training datasets is an important step in improving the overall performance of voice cloning. Ultimately, ongoing research is essential to overcome the limitations of current models and develop synthetic voices that can handle a wider range of language expressions with fluency and clarity. This is particularly important for applications like audiobooks and podcasts where the ability to generate natural-sounding speech with a diverse vocabulary is essential.
### The Challenge of Limited Vocabulary in Voice Models
The accuracy of synthesized speech, even when the voice itself sounds remarkably like the original speaker, can be surprisingly hampered by a limited vocabulary within the trained voice model. This issue arises because the model's ability to understand and produce speech is fundamentally tied to the words and phrases it encountered during its training phase.
One notable consequence of this limitation is the model's struggles with accurately pronouncing words, particularly those that are uncommon or outside the range of its training dataset. This can be especially evident when models are trained on smaller or less diverse audio collections. We're seeing a trend towards newer voice cloning methods relying on generative models of speech, often using minimal audio samples to derive a speaker's unique vocal characteristics. While these methods are showing promise for cloning voices with fewer samples compared to traditional methods that required vast datasets, they can still struggle when faced with unfamiliar vocabulary.
For example, the popular VITS (Variational Inference Text-to-Speech) model can generate remarkably realistic voice clones. However, if the training process hasn't adequately covered a particular word or phrase, the resulting output can become unintelligible. This underscores the crucial role of dataset preparation in voice cloning, highlighting the importance of generating training data specifically tailored for the vocoders to enhance speech quality.
Despite the rapid advancement of voice cloning technologies, adapting them to new speakers with limited data remains a challenge. For instance, successfully cloning an accent that's not well-represented in the model's training data can be difficult, leading to inconsistencies in the synthesized voice.
Many modern voice synthesis models utilize powerful techniques like deep neural networks and sequence-to-sequence modeling to bridge the gap between input text and synthesized speech. However, these advancements still face the fundamental constraint of the model's vocabulary. Developers frequently leverage APIs and platforms like Replicate to expedite the voice cloning process, but ultimately, the quality of the training data, including its vocabulary, remains the core determinant of the model's capabilities.
While ongoing research is actively addressing the limitations of current voice models, there's still much to learn. Researchers are exploring innovative approaches like semantic tokens and advanced audio codebooks to further enhance the quality of the synthesized speech. These innovations aim to address the core issue of vocabulary constraint by giving models a deeper understanding of the meaning behind the words they are tasked with synthesizing, which in turn could lead to more natural-sounding voice outputs. But until we fully crack the code for imbuing models with a comprehensive understanding of language and its diverse facets, the limitations of vocabulary will likely continue to be a key factor influencing the quality of synthesized speech.
Troubleshooting Voice Cloning 7 Common Issues and Their Solutions - Pitch Fluctuations in Extended Audio Segments
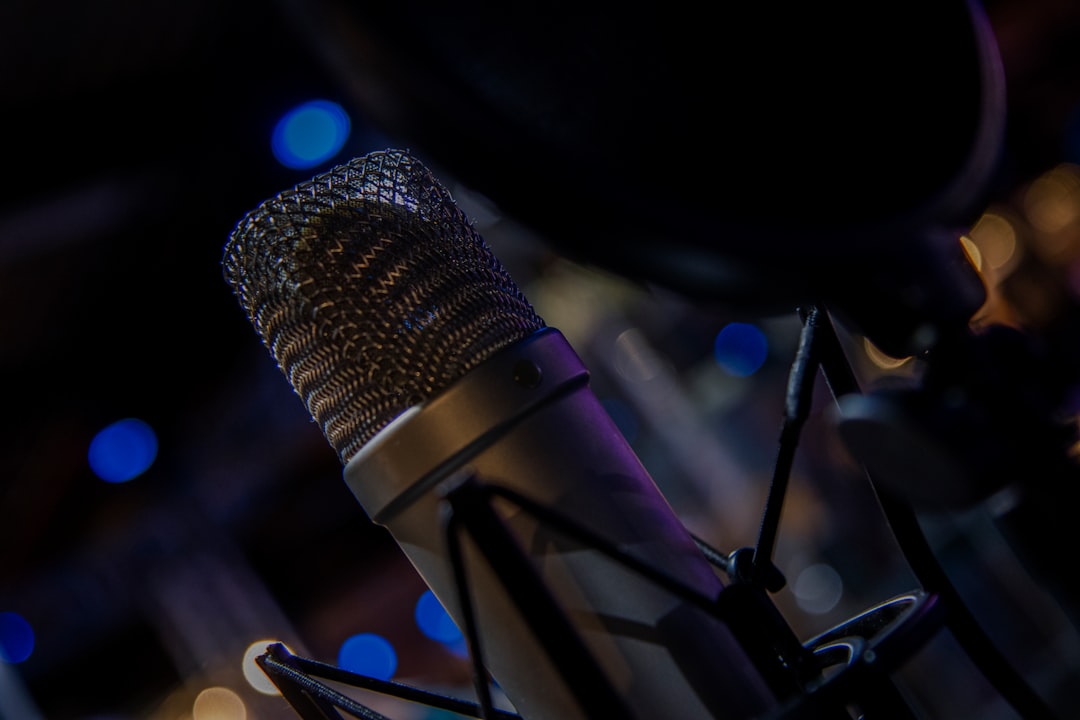
When creating extended audio segments using voice cloning, inconsistencies in pitch can emerge. These fluctuations can stem from the inherent characteristics of the cloned voice, like its natural range of tones or the stability of the model being used. This can manifest as noticeable changes in tone, sometimes even accent-like shifts, which detract from a smooth and consistent delivery. If a voice sounds like it's constantly changing its pitch or overall tone, it breaks the illusion of a natural speaker and can be off-putting. This is particularly apparent in applications where maintaining a consistent vocal presence is crucial, such as in audiobooks or podcasts, where maintaining a constant listening experience is vital.
Improving the ability to maintain consistent pitch in extended audio segments is crucial for advancing voice cloning technology. The goal is to generate voices that not only closely mirror a target speaker's unique vocal qualities but also do so in a way that feels natural. This involves developing methods that can better control the pitch, timbre, and overall tonal characteristics of the generated voice, ensuring consistency throughout even long stretches of synthesized audio. Ultimately, achieving more believable and engaging synthetic voices requires overcoming the challenges of maintaining consistent pitch, allowing the technology to deliver a truly immersive and natural listening experience.
Pitch fluctuations in extended audio segments can be a significant issue in voice cloning, often leading to a less natural and engaging listening experience. While we've made strides in voice cloning, replicating the subtle nuances of human speech, like pitch, remains a challenge.
Humans are surprisingly sensitive to pitch variations. Even a small shift of just 1-2% can be detected, emphasizing the importance of maintaining consistency in cloned voices. This sensitivity becomes even more critical in longer audio pieces, where even minor pitch fluctuations can lead to listener fatigue or a feeling that something is "off."
Furthermore, pitch plays a crucial role in conveying emotion. Higher pitches often signify excitement or happiness, while lower pitches might communicate sadness or calmness. This presents a challenge for voice cloning systems attempting to accurately replicate emotional nuances. If the pitch isn't managed correctly, the emotional tone of the cloned voice can feel artificial or inconsistent.
In longer recordings, harmonic interactions can further complicate pitch variations, introducing phenomena like beating that create an uneven sound. This is especially true when dealing with multiple audio sources, posing an additional challenge for models that need to ensure clarity and naturalness.
Our perception of pitch can even be influenced by the listening environment. Noisy surroundings can affect our interpretation of pitch, making it vital for voice cloning systems to maintain a consistent pitch in challenging acoustic conditions.
The interplay between pitch and formant frequencies (resonant frequencies of the vocal tract) also plays a key role. If a model doesn't accurately replicate formants, it can lead to pronounced pitch fluctuations, resulting in a distorted or unnatural voice.
The subtle pitch changes that contribute to prosody – conveying emphasis and questions – often get lost in translation during voice cloning. When these subtle cues aren't captured correctly, the generated speech can sound robotic and lack the natural rhythm that makes human speech so engaging.
Maintaining a consistent dynamic range in voice cloning, particularly during extended audio segments with more dynamic pitch changes, is difficult for most current systems. This can lead to noticeable drops in voice quality, especially over time.
Real-time pitch modification, crucial for applications like live podcasts, is also challenging. Delays or latency can create a mismatch between the audio input and output, potentially disrupting the natural flow of the conversation.
Many voice cloning models rely on datasets that might not fully capture the natural complexities of pitch fluctuation in human speech. This limits their ability to adapt to atypical or unexpected pitch changes, resulting in outputs that don't sound natural.
Lastly, cultural differences in speech patterns introduce another layer of complexity. Different languages and dialects exhibit unique patterns of pitch fluctuation, making it difficult for voice cloning systems to accurately capture the nuances of intonation across cultures.
These limitations highlight the ongoing need for research and development in voice cloning to ensure we can produce truly lifelike and natural-sounding synthesized voices. We're not quite there yet, but continued work in this space will bring us closer to achieving seamless and emotionally resonant voice cloning for various applications, including podcasts and audiobooks.
Troubleshooting Voice Cloning 7 Common Issues and Their Solutions - Voice Age Discrepancies in Cloning Results
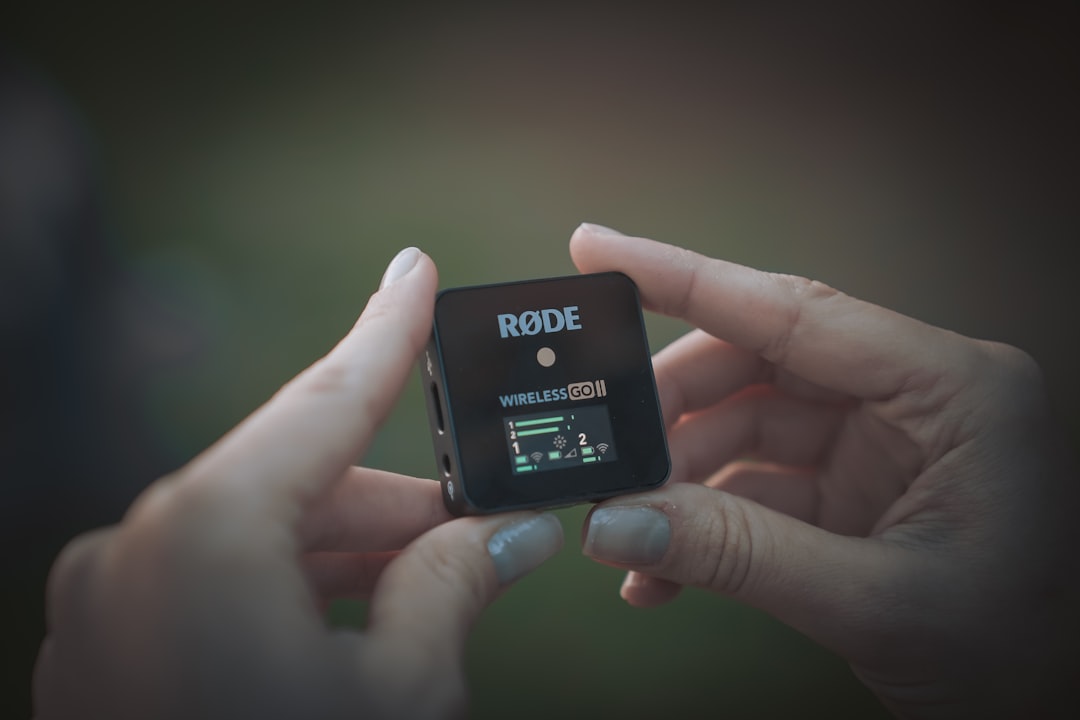
When using voice cloning to replicate a person's voice, accurately capturing their perceived age can be surprisingly challenging. While progress has been made, creating convincing voice clones across different age groups, particularly transitioning from a child's voice to an adult's, often falls short. The reason lies in the complexities of vocal production—the interplay of pitch, resonance, and emotional expression that varies significantly with age. Cloned voices may end up sounding unnatural when attempting these types of conversions. This is a problem, especially in areas where authenticity matters most, such as audio books and podcasts, where listeners are sensitive to any discrepancies that hinder immersion. If we want to move towards truly believable and relatable synthetic voices, it's clear that we must improve how cloning systems handle these age-related differences in sound.
### Voice Age Discrepancies in Cloning Results: A Look Under the Hood
Voice cloning technology, while impressive, can sometimes struggle to accurately represent the age of the speaker it's mimicking. This is particularly true when trying to clone the voice of an older individual and have it sound natural. There's a complex interplay of factors influencing this phenomenon. For example, the human vocal cords change with age, and these changes manifest in the voice's frequency characteristics. Older voices often have a lower fundamental frequency compared to younger voices. If a voice cloning model isn't trained on a sufficiently diverse set of audio examples across various age ranges, it may struggle to generate a synthesized voice that accurately reflects the age of the target speaker.
Furthermore, the spectrum of sounds in a voice also changes with age. Formant frequencies, which are basically the resonant frequencies of the vocal tract, shift over time. These shifts can lead to a synthetic voice sounding surprisingly youthful or unnatural if the training data doesn't properly capture them. Similarly, the overall quality of a voice—its timbre—changes as we age. The voice might become breathier or less resonant. Many cloning algorithms aren't equipped to automatically pick up on these delicate changes, requiring a carefully curated training set that spans age groups to capture this nuance.
Beyond the physical aspects of voice production, the way we speak—intonation and rhythm—also shifts as we get older. Individuals in later life might use a broader range of pitch variations when speaking compared to their younger selves. Cloning models may not effectively recreate these variations, causing the synthetic voice to sound strangely monotonous or flat.
We also need to remember that humans are remarkably sensitive to how someone's voice reflects their age. This means that a slight inconsistency in age perception can be easily picked up on by a listener. If an audiobook is meant for adults, but the voice sounds decidedly youthful, it can shatter the immersion.
It’s not just about the listener's perception. There are also very real biological changes that happen in the larynx and vocal tract as a person ages. Currently, voice cloning models typically don't factor in these biological alterations. This can result in major inconsistencies between the perceived and intended age of the synthetic voice.
Even how quickly someone speaks changes with age. Older individuals frequently adjust their speaking rate, often slowing down over time. However, voice cloning systems that utilize a consistent speech rate across different individuals may not reflect these natural pacing differences. This can lead to a noticeably unnatural sounding output that doesn't match the typical speed we expect from a speaker in a certain age range.
Relatedly, vocal fatigue also increases with age. It introduces subtle modifications in voice quality, like slight pitch drops and less clarity. If a training set isn’t specifically engineered to include examples of this gentle decline, the results of cloning may portray the speaker as surprisingly energetic and younger.
Furthermore, age can significantly impact emotional expression in speech. Older individuals may exhibit a more complex and nuanced range of emotions. Unfortunately, the current state of voice synthesis tools has trouble capturing this correlation. This can create synthetic voices that lack the rich emotional depth typical of older speakers, a key element in genres like audiobooks or podcasts aimed at mature audiences.
Finally, we should consider contextual cues in speech that indicate a speaker’s age, like the way they hesitate or modify their inflection. Voice cloning models that aren’t adequately trained to recognize these contextual indicators can produce voices that aren’t a convincing representation of the speaker’s age.
It's evident that there's a need for more research in this area. While voice cloning has made substantial progress, there's still work to be done to develop more sophisticated models that can accurately synthesize voices representing a wider range of ages. It's a complex challenge, but solving it is vital for ensuring voice cloning delivers a truly natural and believable experience in a wider range of applications.
Get amazing AI audio voiceovers made for long-form content such as podcasts, presentations and social media. (Get started for free)
More Posts from clonemyvoice.io: