The Unique Cadence of Long Island Analyzing Voice Cloning Challenges for Regional Accents
The Unique Cadence of Long Island Analyzing Voice Cloning Challenges for Regional Accents - Capturing the Nuances of Long Island Pronunciation
Replicating the intricate sounds of the Long Island accent poses a substantial hurdle for voice cloning. The accent's particular way of combining sounds, along with its unique vocabulary, reflects a deep-rooted linguistic heritage that is hard to recreate accurately. Experts in language have raised concerns about the potential decline of this regional accent, which might add further difficulty to building authentic voice models. Furthermore, the perception of the accent as a symbol of local identity contrasts with broader trends toward a more standardized way of speaking, making one wonder about the fate of regional accents in voice-related projects like audiobooks and podcasts. Successfully tackling these complexities is essential for any initiative in voice cloning and related fields.
The Long Island accent exhibits a distinctive vowel shift, where sounds like "aw" in words like "coffee" can morph into something resembling "cawfee". This presents a challenge for voice cloning since these technologies rely on accurate identification of individual sounds (phonemes). Replicating these subtle shifts accurately is a hurdle.
The accent blends characteristics of both New York City and suburban speech patterns, making it intricate but also difficult for voice synthesis models to fully replicate. It's not a straightforward derivative of either, making a faithful artificial representation tricky.
Research has revealed that Long Island speech has unique intonation and rhythm, distinguishing it from neighboring accents. These rhythmic nuances are often challenging for voice cloning algorithms to capture correctly, potentially resulting in a synthetic voice that sounds unnatural or even robotic.
The tendency for "th-fronting", where "th" sounds are pronounced as "d" or "t" (as in "da" or "ta" instead of "the"), is a frequent feature of Long Island speech. This can notably alter the sound quality and clarity of audio content. Voice cloning systems need to model this properly to avoid impacting intelligibility.
Another characteristic is "final obstruent devoicing", where sounds at the end of words lose their voicing. This can affect the authenticity of cloned voices, making the synthetic output sound less rich. It requires careful consideration in the cloning process.
The frequent use of glottal stops in Long Island speech poses a particular challenge for audio book recordings and podcast creation, where clarity is paramount. These sudden stops in the vocal flow can result in a choppy, somewhat unnatural synthetic voice, making the listening experience less appealing.
Studies show that Long Island speakers emphasize different syllables in words compared to speakers from other regions. This can alter the meaning of phrases, posing a challenge for systems designed to interpret natural language. Voice cloning needs to consider these nuances.
The area's diverse population – with Eastern European, Hispanic, and Italian influences – contributes to a variety of pronunciation patterns. A truly accurate AI model for voice cloning needs to account for these variations, which is a considerable technical undertaking.
Evidence suggests that the way the Long Island accent is perceived can vary depending on social context. How someone speaks it can change from group to group or setting to setting. Voice cloning systems need to navigate these social dimensions carefully to avoid producing misrepresentations or biased outputs.
The accent's pronunciation patterns are dynamic and evolving. Younger generations tend to employ more neutral sounds compared to older speakers. Thus, the training data for voice cloning models must be flexible and updated frequently to accurately mirror the ongoing changes in speech. If not, the model will sound outdated or inaccurate.
The Unique Cadence of Long Island Analyzing Voice Cloning Challenges for Regional Accents - AI Challenges in Replicating Regional Speech Patterns
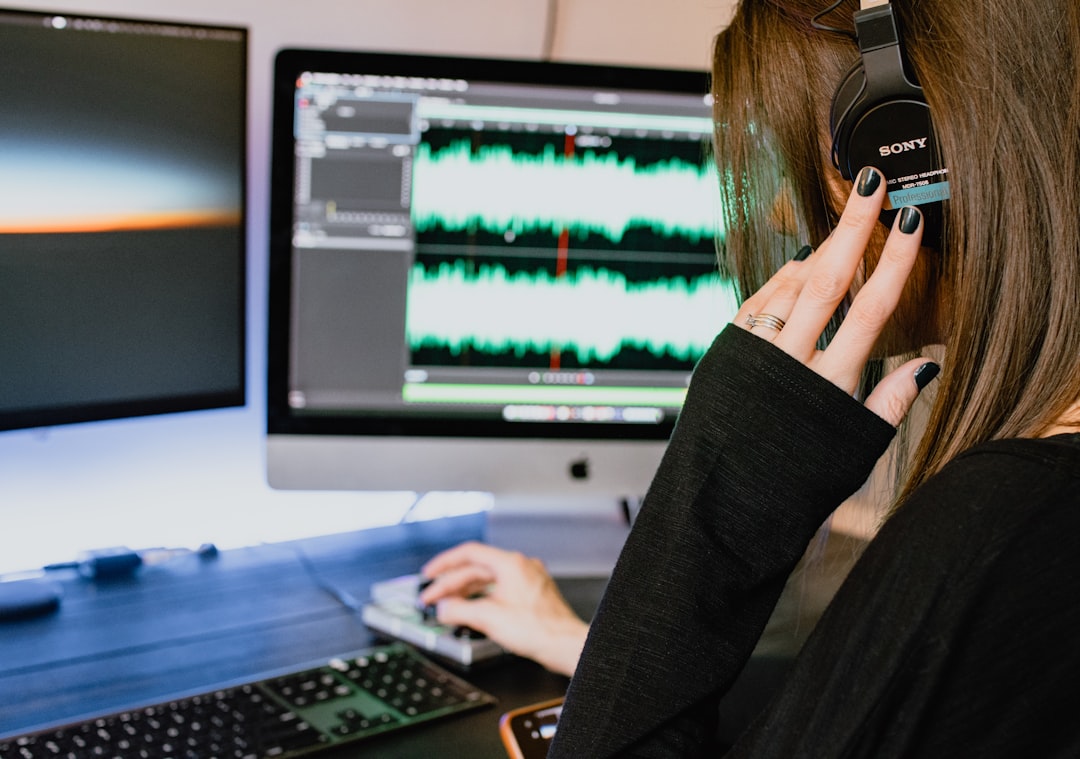
AI faces significant challenges in replicating regional speech patterns, particularly those with unique characteristics like the Long Island accent. Accurately capturing the subtle nuances of these accents, including distinct vowel shifts, rhythmic patterns, and social variations, is a complex endeavor. Voice cloning technologies rely heavily on precise sound production models, and features like glottal stops or specific vowel alterations can be difficult to replicate accurately. Failing to properly model these nuances often results in artificial voices that sound robotic or unnatural, potentially undermining the listener experience in applications like audiobooks or podcasts. Furthermore, the dynamic nature of regional accents—as they evolve due to social influences and generational shifts—demands that AI systems be adaptable and continuously refined. Maintaining the authenticity of cloned voices requires careful consideration of the ongoing changes in speech patterns. This continuous need for adjustment underlines the importance of responsible AI development and collaborative approaches to improve the fidelity of synthetic voices and enhance their overall quality.
AI's journey in accurately replicating regional speech patterns, particularly the intricacies of Long Island's accent, faces several challenges. One key obstacle is the complex interplay of sounds, such as the "intrusive r" where an "r" sound is added between vowels. Accurately recreating these phonetic quirks without sounding artificial is a major hurdle for voice cloning systems.
Further complicating matters is the subtle nature of vocal variations. Pitch and stress, for example, can drastically alter meaning, demanding finely tuned auditory models within the voice cloning process. Moreover, the accent's perception can vary significantly depending on the social setting, which makes building a stable model that doesn't reflect biases or stereotypes difficult.
Temporal phonology, or the timing of sounds, plays a vital role in meaning. Accurately capturing the rhythmic patterns of a Long Island speaker is crucial for natural-sounding synthetic speech and intelligibility, demanding a more sophisticated approach than just simple sound replication. Voices not only convey information but also emotions and social identities. Replicating these emotional nuances inherent in Long Island speech is crucial for a realistic listening experience, especially in audiobook and podcast contexts.
The frequent use of glottal stops, where sounds are abruptly cut off, contributes to a decrease in the richness of sound quality, which is especially problematic for audio production. Synthetic voices can come across as lacking in acoustic depth, highlighting the need for nuanced algorithms. The evolving nature of the accent across age groups further complicates things. Younger generations often exhibit speech patterns more aligned with broader American English, making it vital to incorporate a longitudinal model that adjusts to these linguistic shifts.
Furthermore, the unique blend of cultural backgrounds and personal experiences of individuals contributes to the diversity of the Long Island accent. Accurately reflecting this complex identity within a voice cloning model demands extensive and diverse training data. Phonological merging, where distinct vowel sounds become similar in certain contexts, also presents a significant hurdle, requiring sophisticated modeling techniques to properly distinguish them during the synthetic voice generation.
Finally, the variability in how listeners perceive the accent based on their familiarity with it highlights the importance of testing across various listener groups. The goal is to avoid alienating or misrepresenting specific audiences with synthetic outputs. Ensuring that voice cloning systems are rigorously validated across regions is critical for developing truly representative models.
The Unique Cadence of Long Island Analyzing Voice Cloning Challenges for Regional Accents - Voice Cloning Accuracy for Audiobook Narration
Voice cloning technology is transforming audiobook narration, aiming to create both authentic and engaging listening experiences. While this technology offers a promising path for capturing regional accents, it faces hurdles when attempting to reproduce the subtleties of dialects like the Long Island accent. Replicating the specific sound qualities and speech patterns of a region involves not just accurately generating individual sounds, but also replicating emotional inflections and rhythmic nuances that distinguish a particular voice. This is a difficult task. The ongoing evolution of speech patterns adds another layer of complexity, requiring voice cloning models to be flexible and adaptable to changes in how people speak across generations and social groups, with the goal of creating cloned voices that connect with a diverse range of listeners. However, a significant challenge persists: the risk of generating synthetic voices that sound artificial and robotic, which can detract from the intended effect. This highlights the ongoing need for improvement in the technology, alongside thoughtful consideration of the ethical implications of using this technology.
Voice cloning for audiobook narration presents interesting challenges, especially when dealing with regional accents like Long Island's. The accuracy of cloned voices hinges on how well systems can model the intricacies of intonation. Even subtle pitch variations can carry significant meaning in regional speech, and failing to accurately capture these nuances can lead to synthetic voices that sound unnatural or robotic.
Creating truly accurate voice clones also depends on a robust phonetic foundation. Long Island speech contains sounds not typical of general American English. Unique fricatives or diphthongs necessitate specialized phonetic libraries within the voice cloning process. Simply mapping common English sounds onto Long Island's unique sounds will not be enough for natural-sounding narration.
Beyond purely phonetic elements, cultural influences profoundly impact how people speak. The Long Island accent, for example, isn't uniform across the region. Its nuances can vary based on socioeconomic factors, adding another level of complexity to voice synthesis. To truly mirror the local sound, cloning systems need to account for these social dimensions.
The temporal aspect of speech, meaning the rhythm and timing of sounds, also plays a key role in how we perceive intelligibility. Long Island speakers often have faster speech patterns and a unique rhythm, and voice cloning needs to replicate this accurately. If the timing of sounds in a cloned voice isn't properly represented, it can lead to audio that sounds stilted or unnatural.
The diversity of the Long Island population – with its blend of cultural backgrounds and influences – further complicates the data requirements for training AI voice models. Models need extensive and varied datasets to accurately represent the range of the accent, otherwise the cloned voice may sound inaccurate or biased.
As the field progresses, voice cloning systems are increasingly integrating emotional cues into the synthetic output. This can enhance the listening experience but brings new challenges. Replicating the emotional range found in native Long Island speech remains challenging.
The language itself is in constant flux, with younger generations exhibiting broader American English influences. Voice cloning models must adapt to these shifting linguistic trends to avoid sounding dated or inaccurate. The cloning models need to be dynamic, and constantly updated with new data, in order to stay relevant.
Many systems also struggle with coarticulation, where sounds influence each other in natural speech. The complex sound blending of the Long Island accent requires cloning systems to have finely-tuned articulation models to create smoothly flowing audio.
Research reveals that listeners' experiences and familiarity with an accent can greatly influence their perception of synthetic voices. If a cloned voice doesn't align with listeners' expectations, it might come across as inauthentic. Extensive testing with a diverse range of listener groups is essential for achieving broader acceptance.
Finally, real-world audio conditions, like background noise, can impact how well cloned voices perform in audiobook applications. Voice cloning systems must be robust enough to mitigate these noise sources and maintain clarity and naturalness, mirroring how a native speaker might adjust their speech in various environments. The future of audio content hinges on our ability to overcome these challenges and achieve high-fidelity replication of regional accents.
The Unique Cadence of Long Island Analyzing Voice Cloning Challenges for Regional Accents - Preserving Vocal Identity in Synthesized Speech
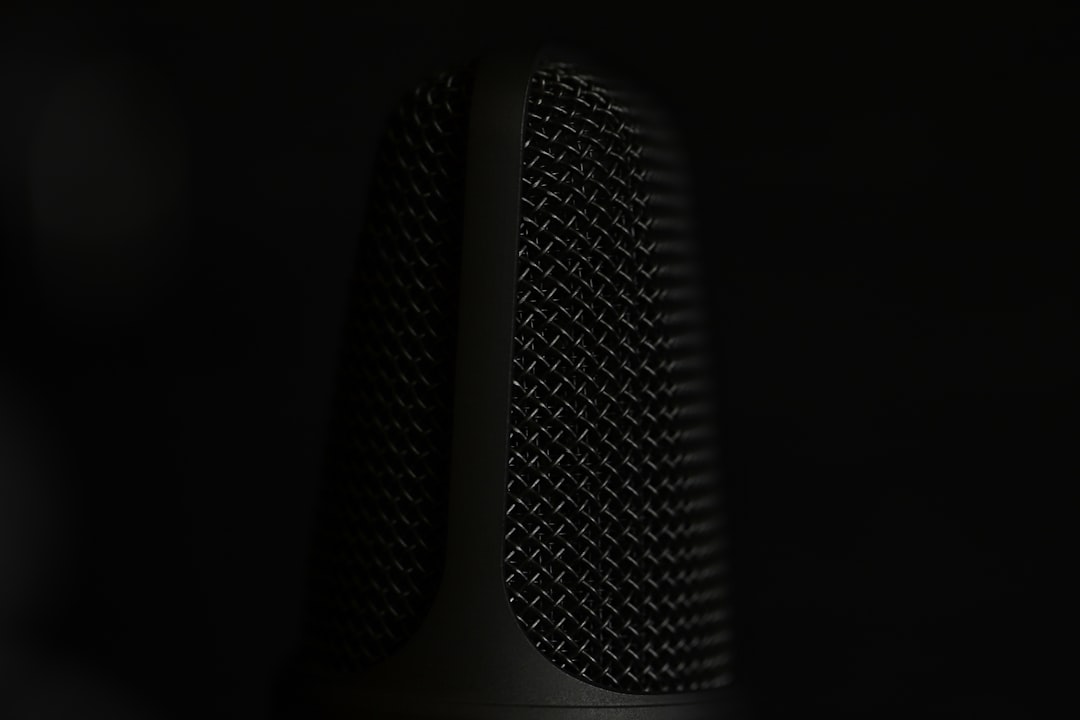
The pursuit of capturing and replicating the unique qualities of human voices in synthesized speech presents a significant challenge, especially when dealing with regional accents like the one found on Long Island. While advancements in voice cloning technologies have led to more natural-sounding and emotionally nuanced artificial voices, accurately replicating the subtleties of these accents remains a complex task. Factors like distinctive vowel shifts, the rhythmic structure of speech, and other specific acoustic characteristics—such as the frequent use of glottal stops—pose obstacles in achieving a truly authentic reproduction of regional speech. The synthetic voices created often struggle to match the nuanced sound production of the human voice, sometimes resulting in an artificial or robotic sound. Furthermore, the dynamic nature of language, with regional accents constantly evolving over time and across generations, demands that voice cloning techniques remain adaptable and use training data that is reflective of these changes. The goal is to create cloned voices that are not only understandable but that capture the essence of human vocal expression and identity, a feat crucial for applications like audiobooks and podcasts where listener engagement depends greatly on the perceived authenticity of the voice they are hearing.
Voice cloning technology hinges on capturing a wide spectrum of vocal characteristics, including pitch, intonation, and speaking rate. However, for regional accents like the Long Island accent, even subtle variations can significantly impact how natural the synthesized speech sounds.
The concept of "intonational phonology," which relates to how pitch variations convey meaning beyond words themselves, presents a major challenge. Voice cloning models need to accurately replicate these pitch changes in Long Island speech to maintain the intended emotional depth of a narrative.
The sophisticated neural network structures used in voice cloning are trained on vast datasets, but effectively capturing regional accents often demands a large amount of localized data. Without sufficient data samples, the synthesized speech might fail to incorporate distinctive features, resulting in unnatural or inaccurate pronunciations.
Research in human auditory perception indicates that listeners can identify discrepancies in synthetic voices with remarkable precision. Therefore, the extent to which voice cloning systems can mimic the subtleties of regional accents heavily influences the overall acceptance of synthetic voices in audio contexts like audiobooks and podcasts.
Some voice cloning algorithms utilize "spectrograms," visual representations of the sound frequency spectrum, to analyze vocal quality more effectively. However, the complex, nonlinear dynamics of human speech production mean that variations in accents or dialects can change the spectrogram, making model training more complex.
The fundamental frequency (F0), a key element in determining voice pitch, can vary significantly between speakers. In Long Island speech, these F0 variations contribute to the accent's uniqueness, and voice cloning systems must accurately reproduce these frequencies to maintain authenticity.
Phrases used to directly address someone (vocative phrases) often exhibit unique intonation patterns in regional dialects. If these patterns are not incorporated into voice cloning models, the resulting synthetic voices may sound less engaging or contextually appropriate.
Replicating the subtle cues of a speaker's age, gender, and social background adds another layer of difficulty. For example, younger Long Island speakers might use more neutral pronunciations, so voice models need to adapt dynamically to avoid sounding outdated or inaccurate.
Real-time audio processing is essential for applications like live podcasts, where voice cloning systems must adapt instantly to unexpected shifts in speech patterns. The challenge is developing algorithms that can maintain naturalness while responding to these unpredictable variations.
Finally, a listener's familiarity with a regional accent significantly influences how they perceive synthesized speech. Long Island residents might be more sensitive to nuances that others miss, emphasizing the need to test synthetic voices with the intended audience to ensure acceptance and a natural listening experience in audio productions.
The Unique Cadence of Long Island Analyzing Voice Cloning Challenges for Regional Accents - Ethical Considerations in Regional Accent Replication
The ethical landscape of replicating regional accents using voice cloning technology is complex, intertwined with questions of identity, authenticity, and the potential for misuse. As voice synthesis technologies continue to refine their ability to recreate human speech, anxieties arise concerning the accurate portrayal of the socio-cultural nuances embedded in unique accents like those found on Long Island. The risk of misrepresenting these accents not only jeopardizes the integrity of the regional identity but also necessitates a discussion around informed consent, especially when individuals' voices are cloned without their explicit awareness or agreement. We must also consider the societal impact of accent-based biases. If synthetic voices misrepresent or perpetuate stereotypes associated with regional accents, it risks reinforcing existing social prejudices. The core challenge lies in the development of voice cloning technology that respects the intricate tapestry of regional speech patterns while simultaneously avoiding the ethical pitfalls that such advancements can create.
Replicating the Long Island accent with voice cloning technology presents a complex challenge due to the high level of phonetic variety across small areas. For accurate voice cloning, it's essential to utilize localized speech data that captures these subtle differences. Otherwise, the resulting synthesized voice may miss crucial accent traits, hindering authenticity.
The Long Island accent is perceived through different cultural lenses, impacting how people interpret its sounds. Voice cloning models should consider the sociolinguistic context alongside phonetic features to avoid perpetuating biases or stereotypes. This is a vital aspect for ensuring responsible use of voice cloning.
Glottal stops, a common characteristic of Long Island speech, can create interruptions in the flow of sound. Accurately mimicking these abrupt vocal pauses is essential for a natural-sounding synthetic voice. Failure to properly replicate them might result in a choppy, jarring experience for listeners, diminishing the effectiveness of the voice cloning in audiobook and podcast settings.
Intonation—the way pitch changes to convey meaning—holds unique nuances in Long Island speech. Voice cloning projects need to meticulously replicate these intonational patterns to ensure that the cloned voice maintains the correct emotional context. This is vital for creating engaging and meaningful narratives in audiobooks.
The speed and rhythm of speech play a significant role in how easily we comprehend what's being said. Long Island speakers tend to have a faster speech rate and unique rhythmic patterns, and voice cloning technology must faithfully replicate these temporal aspects. If the timing of sounds in a synthesized voice isn't accurate, it can result in audio that sounds stilted and unnatural, hindering listener comprehension and immersion.
The Long Island accent isn't static; it undergoes continuous change across generations. Voice cloning systems need to integrate ongoing updates and adaptive training data to keep up with these linguistic shifts. Doing so ensures that synthesized voices remain relevant and continue to accurately reflect the accent's evolution.
Research reveals that people are quite good at detecting when a voice is artificial, and this ability is heightened when listeners are familiar with the accent in question. Long Island residents, for instance, might be more sensitive to subtle inaccuracies that non-local listeners miss. Thus, thorough testing with a representative audience is key when developing voice cloning models for this region.
Unique syllable stress patterns in Long Island speech can have significant effects on the meaning of spoken phrases. Voice cloning systems must accurately capture and reproduce these stress variations to maintain the intended meaning of the content. This is particularly crucial for audiobooks, where precise meaning is vital for conveying the story's intended message.
Voice cloning algorithms often utilize visual representations of sounds (spectrograms) to analyze vocal quality. However, the complex, irregular nature of human speech patterns, especially accent variations, can complicate this process. The changes in the spectrogram representation of Long Island speech present a particular challenge for training accurate models. Advanced machine learning techniques are needed to handle these accent-specific features effectively.
In live podcasting, for example, voice cloning systems need the ability to swiftly adapt to spontaneous changes in speech patterns. The ability to make modifications in real-time while still maintaining a natural-sounding output is critical for enhancing audience engagement in a dynamic conversational setting. This is an area where voice cloning technology faces exciting opportunities and development challenges.
The Unique Cadence of Long Island Analyzing Voice Cloning Challenges for Regional Accents - Advancements in Zero-Shot Voice Cloning Technology
Recent breakthroughs in zero-shot voice cloning are significantly impacting audio production, including audiobooks and podcasts. These advancements have led to remarkable improvements in speech synthesis, with some models achieving near-human quality. Techniques like Dynamic Convolution Attention have been instrumental in addressing the challenge of synthesizing longer audio segments while maintaining high intelligibility. Despite these gains, significant hurdles remain, especially in accurately capturing the distinct features of regional accents. The Long Island accent, for instance, presents a complex challenge due to its unique cadence, vowel shifts, and rhythmic patterns. Replicating these elements accurately is crucial for producing synthetic voices that sound natural and avoid a robotic quality. As the field moves forward, continuous refinement of voice cloning technology is necessary to ensure that diverse accents are represented authentically, contributing to more engaging and immersive listening experiences for a wider audience. While progress is being made, the full potential of seamlessly capturing regional nuances within voice cloning remains an active area of research and development.
Zero-shot voice cloning, a remarkable advancement in speech synthesis, enables systems to generate voices resembling a particular person's without needing prior training specifically on that voice. This capability relies on a model's ability to extrapolate from a few audio samples of a different speaker, adapting its understanding of voice characteristics to produce synthetic speech in a new, unseen speaker's style.
At the heart of zero-shot voice cloning lie sophisticated deep learning techniques like Generative Adversarial Networks (GANs). These networks are pivotal in synthesizing high-quality audio that mimics the natural pitch and tone of human voices. However, this approach faces the persistent challenge of coarticulation—accounting for how individual sounds blend and interact in natural speech. Successfully navigating this complexity demands intricate algorithms to ensure the output is smoothly flowing and coherent.
Recently, researchers have made strides by employing transformer models, which are adept at learning from extensive datasets. These models demonstrate a heightened ability to grasp the nuances of various accents, including the distinct features of regional speech like that found on Long Island. This allows for more precise speech synthesis compared to earlier methods.
While zero-shot voice cloning can produce synthetic voices capable of conveying emotional depth, accurately capturing the subtle shifts in emotional intonation characteristic of regional accents remains a challenge. Sometimes, this can lead to outputs that sound less authentic, needing further refinement.
The timing and rhythmic structure of speech (temporal phonology) are essential in achieving natural-sounding voice clones. Zero-shot systems need to effectively integrate these elements into their models to generate voices that adhere to regional speech patterns, avoiding a robotic or artificial quality.
A considerable body of research explores the ethical dimensions of voice cloning. Zero-shot techniques particularly raise concerns about the possibility of identity misrepresentation and the potential for malicious use. These concerns become more acute when a voice is cloned without proper awareness or consent.
Thankfully, incorporating mechanisms for user feedback into zero-shot voice cloning systems is an avenue for improvement. Through this feedback, models can adapt and enhance their performance over time, adjusting to listener preferences and perceptions.
The demographic composition of the data used to train zero-shot voice cloning models can significantly influence the authenticity of the resulting synthetic voices. Using datasets that include a diverse representation of speakers can help produce more genuine voices, allowing the technology to reflect the breadth of linguistic and cultural variations.
Importantly, maintaining high-quality audio in synthesized speech, especially in challenging audio environments, is vital for listener comprehension. Zero-shot models must be resilient enough to preserve clarity and accuracy, mirroring how humans adapt their speaking to overcome background noise or other environmental factors. The goal is to generate voices that feel as natural and clear as a live speaker in various situations.
More Posts from clonemyvoice.io: