Get amazing AI audio voiceovers made for long-form content such as podcasts, presentations and social media. (Get started for free)
The Science Behind Playlist Curation How Audio Algorithms Shape Modern Music Discovery
The Science Behind Playlist Curation How Audio Algorithms Shape Modern Music Discovery - Audio Fingerprinting Technology Behind Modern Playlist Recognition
Audio fingerprinting has become a core technology in modern music organization, particularly in the realm of playlist curation. It essentially translates sound into a unique, identifying fingerprint, much like a DNA sequence for audio. This fingerprint is derived from analyzing core sound elements, including things like sample rate and the way the audio data is stored. The ability to create these fingerprints makes it possible to instantly recognize and categorize any piece of audio, even when it's mixed with other sounds or modified.
The effectiveness of these audio fingerprints has been significantly improved with the advent of artificial intelligence and machine learning. These improvements are crucial in environments like listening to music through a speaker with other sounds around it. This means these algorithms can accurately identify audio in more complex, real-world scenarios. The core advantage of audio fingerprinting, however, is its independence from traditional methods. It bypasses the need for metadata tags, allowing for consistent identification across various audio formats. This technology is now used widely for sorting and identifying songs on streaming services, showing its importance in the music experience.
Audio fingerprinting delves into the core of sound, converting it into a unique signature or 'fingerprint'. This allows for lightning-fast matching of songs, even when the audio is distorted or low-fidelity, such as from a live recording. This method bypasses the limitations of traditional methods relying heavily on song metadata (like title and artist). Instead, it focuses on core sonic elements like melody, rhythm, and harmonic structures for rapid identification.
The fingerprint creation process relies on spectrograms—visual representations of audio over time, showcasing its frequency content. This allows for detailed analysis and identification of specific sounds and their unique characteristics. The incredible speed of some audio fingerprinting algorithms is truly impressive, allowing identification from a mere fraction of a second of audio input. This efficacy opens doors for diverse uses, extending beyond just streaming music to encompass broader audio tasks.
Beyond music identification, audio fingerprinting plays a pivotal role in voice recognition systems. By analyzing distinctive vocal traits, it enhances capabilities such as voice cloning and customer service automation. The improved precision of these systems translates to more responsive and accurate user experiences. This same technology can be applied to the audiobook production process, ensuring consistency of sound quality by detecting variations or anomalies in the recording, contributing to a more polished final product.
The blending of audio fingerprinting with machine learning has led to hybrid systems that can identify obscure music tracks and adapt to evolving audio trends. This opens up new frontiers for content identification in different audio formats and genres. Podcast production is another area benefiting from this innovation. Producers employ these techniques to maintain control over their content, ensuring proper attribution and compliance with copyrights when utilizing music or sound effects from external sources.
Noise resistance is a pivotal development in the field, as these systems now effectively filter out background noise and interference when recognizing songs. This is particularly crucial in busy public settings. The development of content-based retrieval systems is a remarkable application of this technology, enabling users to efficiently search for specific audio segments within extensive libraries with pinpoint accuracy. This capability is profoundly altering the way we experience and discover new music.
The Science Behind Playlist Curation How Audio Algorithms Shape Modern Music Discovery - Machine Learning Models in Voice Pattern Analysis for Music Recommendations
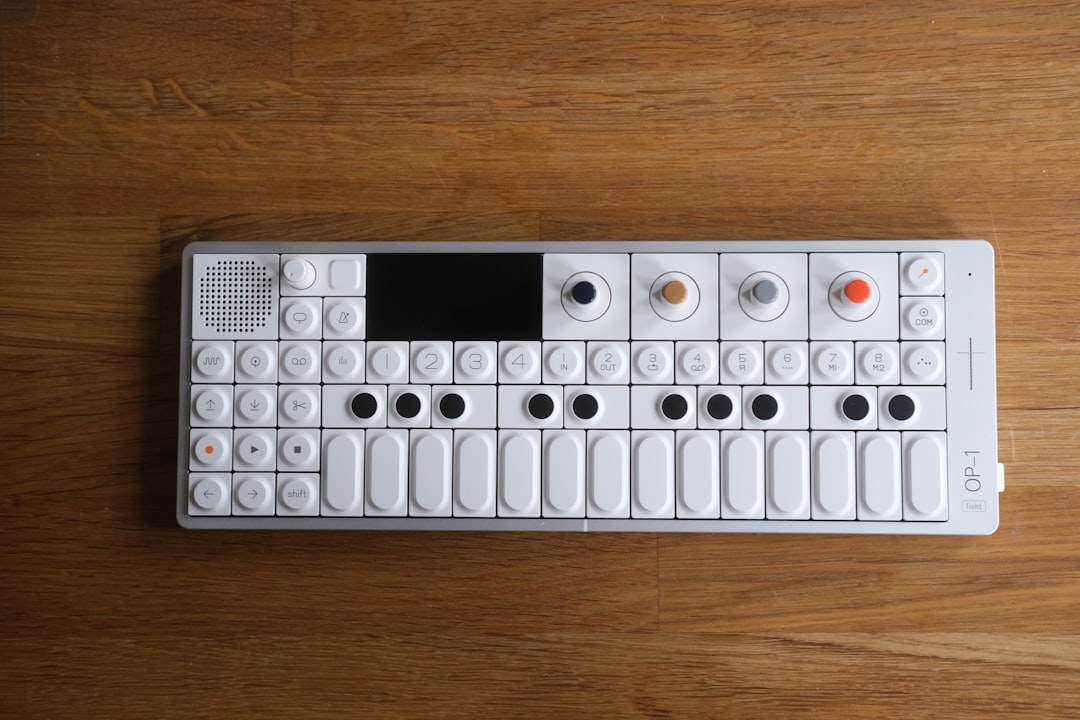
Machine learning models are increasingly important in analyzing voice patterns for music recommendations. These models can decipher intricate aspects of audio data, identifying unique traits like rhythm, melody, and vocal styles. By using speech-based emotion recognition, they can even translate the emotions expressed in a user's voice into specific musical suggestions, leading to a more personalized listening experience.
These systems are constantly evolving, offering a more profound understanding of user preferences. They incorporate a blend of sociological and musicological factors to improve the precision of recommendations. As AI progresses, the incorporation of emotional context and sophisticated pattern recognition transforms the way individuals discover music. It fosters a richer, more engaging audio landscape.
While these developments offer exciting possibilities, they also raise concerns. One area of concern is the potential for over-reliance on automated recommendations and the subsequent impact on the diversity of music discovery. It's important to consider the potential for this technology to shape, or even limit, the variety of musical experiences people have. The future of music discovery will likely be shaped by ongoing research and improvements in these models, which continually strive to achieve a more precise and intuitive match between listener and music.
Machine learning models are increasingly being used to analyze the intricacies of human voice, revealing patterns and trends that can inform music recommendations in surprisingly nuanced ways. For instance, algorithms can analyze the unique sonic characteristics of a person's voice, called timbre, to identify subtle emotional qualities and recommend music with a similar emotional vibe. This is like finding a soundtrack that truly speaks to your inner state. Further, these models are adept at deciphering emotional cues within our voices, a powerful tool in building recommendation systems attuned to our current emotional landscapes. Such techniques have shown promising results in therapeutic audio applications, where music selections are curated to support a specific emotional need.
These systems are becoming increasingly sophisticated in their ability to adapt the music experience to the listener’s environment. If a listener finds themselves in a noisy setting, the recommendation system might offer up music that is more impactful and able to cut through the ambient sound. It's like having a personal DJ that knows when to crank up the volume to match the setting. The same kind of clever adaptations are being explored for podcast recommendations. The AI algorithms can evaluate the vocal style and content of podcasts, suggesting future content based on an individual listener's history. This means you can discover a broader universe of podcasts that might just resonate with you, opening up new avenues for learning and entertainment.
Furthermore, the synergy between voice cloning technologies and music recommendations is quite fascinating. Voice cloning involves recreating a voice digitally, often with incredible accuracy, while music recommendations suggest tracks based on a variety of user preferences and listening habits. By melding these technologies, the idea of having a music curator that actually sounds like you, becoming the virtual host of your musical experiences, becomes possible. This type of customization isn't just about choosing songs based on genre, but tapping into the very soundscape you're drawn to. At a fundamental level, algorithms can delve deeper into musical elements like rhythm and pitch within the music a user enjoys, making suggestions that aren't just thematically similar but also capture a nuanced appreciation of musical structure and composition. This allows us to explore a wider spectrum of music outside our usual boundaries, suggesting cross-genre possibilities based on a shared vocal style or the emotional underpinnings of a musical piece.
Interestingly, the way people interact with their devices can play a significant role in tailoring their listening experience. Machine learning algorithms can learn from the way a listener uses voice commands and adjust future recommendations accordingly. This interaction provides a valuable feedback loop that helps refine the AI's understanding of a listener’s preferences. The possibilities don't end with personal devices. It's intriguing to consider how these models could be used at live music events, possibly even sampling the audience's vocal reactions to influence the song selection of the performing artist, generating real-time feedback that impacts the artistic flow of a concert. Beyond music, applications such as audiobook production heavily depend on the ability to detect subtle variations and irregularities in audio recordings. These discrepancies in voice volume and clarity, if left unchecked, can dramatically impact the listener’s overall experience. Machine learning models provide a powerful tool for editing and ensuring a high-quality audio product for consumers. It's a testament to how versatile these technologies are.
The Science Behind Playlist Curation How Audio Algorithms Shape Modern Music Discovery - The Role of Natural Language Processing in Song Metadata Organization
Natural Language Processing (NLP) is increasingly vital for organizing the information associated with songs, a crucial element in improving how users interact with music platforms. NLP tools like text mining and sentiment analysis allow systems to better categorize songs by considering both the words in the lyrics and the audio characteristics, leading to more insightful music discovery. Datasets like the WASABI Song Corpus showcase this change, offering a massive collection of songs with detailed metadata extracted from lyric analysis, which then informs the kinds of music recommendations that are delivered to users. Unfortunately, older methods tended to focus on just the sound of music, missing the subtle meanings within song lyrics, which points to the need for a more comprehensive approach to music that respects both aspects of a song. As NLP technology continues to mature, it holds the promise of fostering a more detailed understanding of the nature of music, which can then be used to improve playlist creation and amplify the ways we experience audio content. While this presents some exciting possibilities for the future, it's important to be mindful of the potential for bias in algorithms, and to always be aware that algorithms are not people and should not be treated as such.
Natural language processing (NLP) is emerging as a crucial tool in the intricate world of music organization, particularly in the realm of song metadata. It's not just about genre or artist anymore; NLP delves into the meaning behind the lyrics, uncovering the themes, emotions, and narratives embedded within songs. This opens up exciting possibilities for playlist creation, allowing for contextually richer and more insightful selections that go beyond simple categorization.
NLP-powered recommendation systems are becoming increasingly adept at tailoring musical experiences to individual tastes. By analyzing the lyrical content of songs, these systems can suggest music aligned with a listener's current mood or activity. This leads to more intuitive and personalized playlists, making the whole music discovery process much more insightful.
The ability to automatically extract and enrich metadata from lyrics and user-generated content offers a significant advantage. NLP algorithms can glean valuable information about a song's cultural significance or current trends, bypassing the need for manual oversight and accelerating the organization process.
Traditionally, genre classification has relied on manual tagging, a process that's inherently subjective and often inaccurate. NLP offers a more objective approach. By analyzing linguistic patterns and stylistic features in song lyrics, it can automate the genre classification process with enhanced accuracy. This allows for better organization and, consequently, a more efficient music discovery experience.
The integration of NLP with voice command interfaces is revolutionizing how people interact with audio platforms like podcast and streaming services. Users can now voice specific topics or themes they're interested in, allowing for a more natural and fluid way to discover and consume audio content, bypassing the need to wade through endless lists.
User-generated content like reviews or social media posts can be valuable in understanding how people feel about music. NLP can parse this unstructured text and transform it into actionable data, which allows the refinement of playlists and the creation of more contextually relevant recommendations based on social sentiment.
One of the more intriguing applications of NLP is its potential for providing real-time feedback on song lyrics or themes. Music services could gather listener responses and dynamically adjust playlists in real-time, making them evolve based on active user feedback, creating a truly personalized listening journey.
Certain NLP techniques are specifically designed to extract emotional undertones from both audio and lyrics. This opens the possibility of precisely matching music to a listener's current emotional state or preferences, building a deeper connection to the music they experience.
The applications of NLP extend beyond music streaming. In audiobook production, for instance, NLP aids in identifying irregularities in the spoken text, such as repeated phrases or inconsistencies in vocal delivery. This capability ensures a more refined and polished auditory experience for the listener, a key concern in enhancing audio quality for consumers.
Lastly, traditional collaborative filtering, a method used to recommend music based on the preferences of like-minded users, can be significantly enhanced through NLP. By analyzing the lyrical preferences of users, the system can uncover shared musical interests and suggest new music with a higher probability of resonating with a listener's taste. This is a powerful example of how NLP can enrich traditional techniques, pushing the boundaries of music discovery.
In conclusion, while audio fingerprinting laid the foundation for efficient audio identification, NLP adds a new layer of understanding to the sonic world, moving beyond raw sound data and into the realm of meaning. This new layer is enabling more nuanced and personalized musical experiences, from genre classification to emotion-based recommendations. It’s an exciting time in the evolution of audio, and the integration of NLP promises to fundamentally shape how we interact with and discover music in the future.
The Science Behind Playlist Curation How Audio Algorithms Shape Modern Music Discovery - Deep Neural Networks and Their Impact on Cross Genre Music Discovery
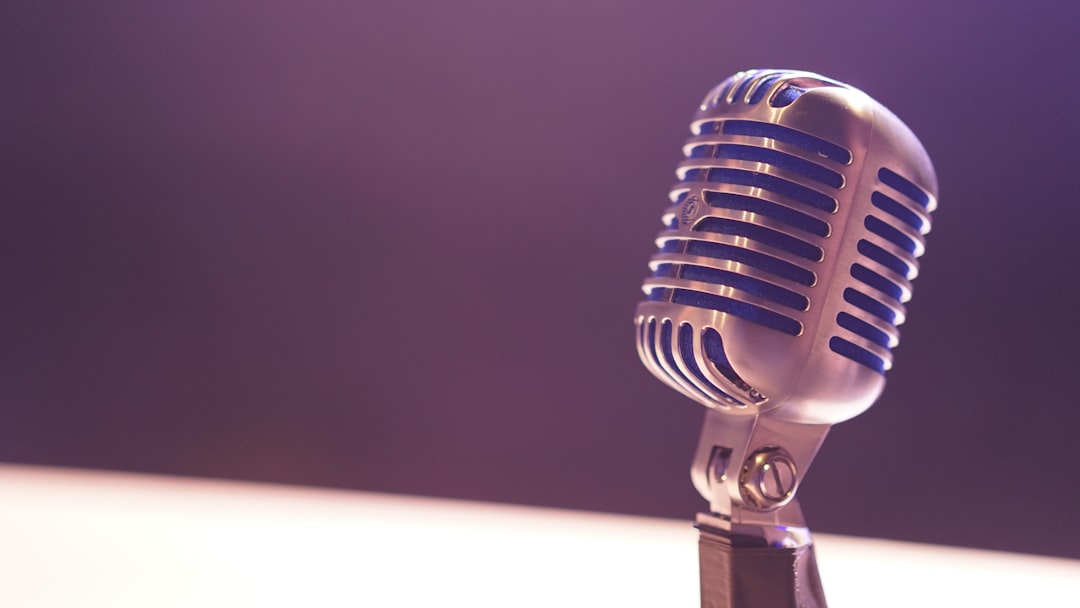
Deep neural networks (DNNs) are transforming how we discover music across genres. They achieve this by analyzing both audio characteristics and lyrics in ways traditional methods couldn't. This detailed analysis leads to significantly improved accuracy when categorizing music into different genres. With access to vast musical datasets, DNNs can uncover subtle connections and patterns between diverse musical styles, allowing for a richer understanding of music. The ability to incorporate both audio and lyrics into the analysis process allows for more refined music recommendations that inspire listeners to explore genres they may not have considered before. This creates a more dynamic and personalized music experience. While these advancements are promising, there's a risk that over-reliance on algorithms might restrict the discovery of a broader range of music, ultimately impacting the diversity of our musical journey.
Deep neural networks (DNNs) are progressively being used to enhance music discovery across genres, offering a more sophisticated understanding of music by examining both the sonic characteristics and the lyrics. These networks have the ability to go beyond traditional methods of music classification, which often rely on simple binary categorizations, allowing for a more nuanced exploration of music based on subtle emotional shifts and underlying musical textures.
Algorithms based on deep learning are being developed to analyze and arrange music playlists, significantly improving music recommendation systems. For instance, one study demonstrated that a specific approach to music genre classification using deep learning significantly increased accuracy, moving from roughly 81% to over 90%. This improvement is largely due to the capability of these models to extract a broader range of features from the audio data compared to earlier methods. Researchers have utilized datasets like MuMu, encompassing a massive collection of albums and songs, to train deep learning models for multi-label music genre classification. Raw audio formats such as MP3, WAV, and FLAC play a crucial role in the training of these algorithms because they preserve the expressive traits of the original music signals.
The technology behind music generation has seen significant advancements, evolving from earlier artificial neural networks to sophisticated deep learning approaches. DNNs excel at extracting higher-level feature expressions from massive and complex datasets, which helps in improving the understanding and classification of musical genres. Various deep learning architectures, including convolutional neural networks (CNNs) and depthwise separable convolutions, are utilized in audio processing tasks such as music recommendation and transcription. Furthermore, incorporating complementary information derived from both the audio and the lyrics contributes to more efficient music retrieval and recommendation algorithms.
Ongoing research in this field is exploring novel architectures for musical representations, aiming to enhance the applications of deep learning in audio and music domains. While the results are promising, there are still areas that need attention. For example, these DNNs are often trained on massive datasets which may contain inherent biases that can affect the outcomes of the recommendations. Furthermore, a reliance on these automated systems could potentially limit the exposure to diverse musical genres. However, with continued research, DNNs show great promise in facilitating cross-genre music discovery and can potentially create more personalized and insightful music experiences for users.
A fascinating aspect is the use of transfer learning, a technique where DNNs trained on a specific type of music can be adapted to identify related patterns in other genres. This approach demonstrates that DNNs can bridge the gaps between seemingly unrelated genres, identifying shared features or patterns that are hard to discern by human ears. Additionally, the ability of DNNs to analyze vocal styles, beyond simply the musical instruments, offers new possibilities for personalization. DNNs can decipher subtle changes in vocal tones and characteristics to provide a more accurate and nuanced match between listener and music.
Furthermore, the application of DNNs is expanding beyond music. Podcasts and audiobook production are also beginning to leverage the power of these technologies. By analyzing the patterns within the spoken words in podcasts and audiobooks, DNNs can provide insight into content that might be of interest to a listener based on their musical listening habits. This also has implications for the production quality of audiobooks, allowing producers to identify and address any inconsistencies or quality issues.
While DNNs have a great deal of potential, their continued development necessitates a critical evaluation of their implications. The impact on music diversity and the potential for bias in the data and algorithms used to train these systems needs ongoing consideration. It will be important to ensure that the use of these systems does not inadvertently restrict access to the incredible diversity of music available.
Despite these challenges, DNNs are pushing the boundaries of how we interact with and experience music. With continued research, the application of deep learning in music and related fields like podcast and audiobook production will lead to increasingly sophisticated and tailored auditory experiences.
The Science Behind Playlist Curation How Audio Algorithms Shape Modern Music Discovery - Psychoacoustic Models Applied to Personalized Music Selection
Psychoacoustic models are becoming increasingly vital in tailoring music recommendations to individual listeners. By understanding how humans perceive and react to sound, these models can go beyond basic audio analysis to consider the emotional impact of music. This insight enables the creation of recommendation systems that connect audio features with emotional responses, resulting in a more engaging and personalized listening experience. The ability to generate playlists that are not just based on genre but also on a listener's emotional state showcases the power of these models. This integration of psychoacoustics with modern algorithms has paved the way for more intuitive and effective playlist curation. Nonetheless, it's crucial to acknowledge the potential downsides. While these technologies refine the music discovery process, there's a risk of limiting the diversity of musical experiences if not carefully managed. As we move toward a future of more immersive and individualized audio experiences, the role of psychoacoustic models in shaping the future of sound design, particularly in areas like voice cloning and podcast production, will undoubtedly continue to expand, shaping the sonic landscape of future listening experiences.
Psychoacoustic models delve into the fascinating connection between how we perceive sound and our musical preferences. They analyze how humans react to sonic elements like pitch, tempo, and dynamics, ultimately helping to create more tailored and emotionally resonant music selections. These models can essentially decode the emotional impact of music, leading to a deeper understanding of how sound influences our feelings.
It's intriguing that even subtle shifts in frequency can drastically alter our emotional response to music, as psychoacoustic research has shown. For instance, a slight change in tuning can make a song feel more uplifting or melancholic, highlighting the importance of these small details in personalized recommendations. Algorithms must be sensitive to these nuances to accurately reflect the intended emotion in musical compositions.
In voice cloning, psychoacoustic principles act as a guide for how a synthesized voice should adapt its tone and inflection to convey various emotions, making it sound more natural and relatable. This approach enhances the experience whether the listener is immersed in a piece of music or an audiobook. However, we are still learning about the most appropriate ways to generate and implement human vocal nuances.
One of the benefits of integrating psychoacoustic models into music selection is their ability to anticipate listener preferences based on their environment. For example, music that maintains emotional depth while remaining perceptually clear could be ideal in noisy settings, creating an optimized listening experience. This understanding can lead to algorithms that account for a variety of listening conditions.
Exploring the use of psychoacoustic analysis in podcast production presents a novel path for dynamic audio adjustments, adjusting audio quality based on the content's emotional tone. An algorithm might manipulate background sounds or enhance clarity using psychoacoustic filters to guarantee a more engaging experience despite potential fluctuations in audio quality. It would be interesting to test this with listeners with sensory sensitivities.
Psychoacoustic research suggests that rhythm and tempo can significantly impact cognitive load. This could lead to personalized playlists not only catering to mood but also designed for enhanced focus or productivity. This ability opens up new possibilities for how music can complement our mental state. There may be unforeseen implications for those prone to sensory overload and the study needs to include those with medical issues in future investigations.
Psychoacoustic models can also inform audio mixing practices within audiobook production. Ensuring emotional highs and narrative turns are balanced with the desired tone can enhance listener engagement and retention. By skillfully controlling volume fluctuations and emphasizing certain frequencies, producers can create a more compelling auditory experience. It would be valuable to research which genres of audio books work best with which mixing techniques.
Interestingly, some psychoacoustic theories suggest specific frequencies can induce physical responses like relaxation or tension relief. If this is accurate, music recommender systems can potentially select tracks that complement or counteract a listener's current physiological state. The research here has interesting implications, particularly in the use of music for emotional well-being and therapeutic purposes. More experimentation is warranted.
The integration of psychoacoustic principles into voice recognition technology allows systems to better discern emotional cues from a user's voice, expanding the interaction beyond simple commands. The systems would then respond not just to what the user says but also to how they say it, adding a layer of emotional nuance. The data and safety concerns associated with emotionally driven voice recognition need to be examined with more scrutiny.
Despite the benefits of psychoacoustic models, challenges remain, especially in recognizing that individuals perceive sound differently based on experiences and cultural backgrounds. This inherent variability highlights the need for continuous refinement in order to create inclusive and accurate music recommendations for a broad range of listeners. We must understand the underlying mechanisms that lead to subjective experiences to move forward in a responsible manner.
Get amazing AI audio voiceovers made for long-form content such as podcasts, presentations and social media. (Get started for free)
More Posts from clonemyvoice.io: