Get amazing AI audio voiceovers made for long-form content such as podcasts, presentations and social media. (Get started for free)
The Impact of Speaking Rate on Voice Cloning Accuracy A 2024 Analysis
The Impact of Speaking Rate on Voice Cloning Accuracy A 2024 Analysis - Speaking Rate Variations Influence Voice Cloning Fidelity
The speed at which someone speaks, or speaking rate, is a significant factor in how well voice cloning technology can recreate a person's voice. The quality of the cloned voice, including how natural it sounds and how closely it matches the original, is strongly tied to these variations in speaking pace. While research hasn't shown a clear connection between the pitch of a voice and speaking rate impacting voice cloning quality, the speed of speech itself has a noticeable influence on the realism of a generated voice. For instance, faster speaking can change how breath groups are structured, which affects the overall flow and feel of the audio. Creating truly accurate voice clones requires careful selection of training data that reflects a wide variety of the speaker's voice characteristics, encompassing different emotional states and speaking styles. Despite advancements, producing convincingly authentic synthetic voices continues to present challenges for the field, underscoring the need for ongoing efforts to enhance both the input quality and processing of speaking rates. As voice cloning technology continues to evolve, improving how it handles different speaking speeds and refining the quality of the training data remain critical aspects of future development.
How a speaker's pace influences the quality of a cloned voice is a key aspect of this research area. We typically speak between 4 and 5 syllables per second, and deviations from this natural rate can noticeably affect how realistic a cloned voice sounds. When speech goes too slow or too fast, the algorithms that attempt to reproduce it struggle to maintain accuracy, often resulting in robotic-sounding outputs.
Our brains process language at a rapid pace, absorbing between 200 and 300 words each minute. If a cloned voice can't match this natural rhythm, it sounds artificial and can disrupt comprehension. Beyond just the speed, the way we stress syllables and change our tone (prosody) also shifts with our speaking rate. If a cloning model doesn't capture this, the cloned voice can sound emotionally flat.
In applications like audiobook production, pacing is crucial for audience engagement. A slow pace can build suspense or create intimacy, while a fast pace can generate urgency. Voice cloning systems that fail to adapt to these diverse pacing needs may fall short of providing truly immersive experiences.
Another hurdle is capturing the natural, often irregular flow of conversation. Pauses, hesitations, and changes in pace that are normal in human speech often confuse these systems, hindering the ability to create a natural-sounding clone. This highlights a common misconception. Producing effective voice clones often needs diverse audio data with variations in speaking rate. This training data helps the algorithms learn to adapt dynamically to the ever-changing pace of spoken language.
There’s a link between how we feel and how quickly we talk. We tend to speak faster when excited, slower when thoughtful. Cloned voices need to mirror this relationship for authenticity. Podcast production is another area where speaking rate is important. Educational podcasts might favor slower speech to allow listeners to process information, while other genres might benefit from a more rapid pace. Cloning systems need to be adaptable to suit these differing content needs.
Lastly, the training data used to create voice clones is vital. If the training data focuses mainly on one speaking rate, the cloning system will struggle to replicate variations in pace, which in turn limits its usefulness in a wider range of applications. In the broader field of AI-driven voice cloning, ensuring a wide variety of speaking rates within the training data is key to creating natural and adaptable clones.
The Impact of Speaking Rate on Voice Cloning Accuracy A 2024 Analysis - ZSTTS2 Systems Revolutionize Voice Synthesis from Limited Samples
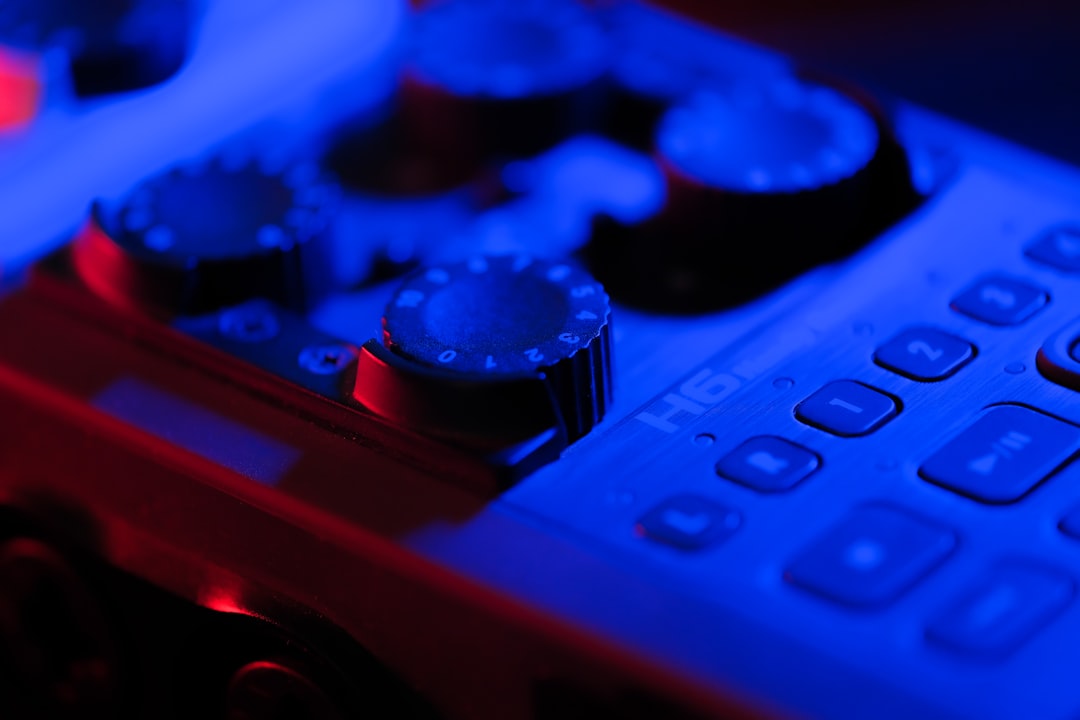
The emergence of ZSTTS2 systems represents a notable breakthrough in the field of voice synthesis. These systems are revolutionizing how we create artificial voices, specifically by achieving high-quality voice cloning from remarkably small amounts of audio. Essentially, they're able to learn the nuances of a speaker's voice and replicate it using sophisticated deep learning techniques, even with just a few sound samples. This capability has immediate applications in fields like audiobook creation and podcasting, where a voice that sounds human-like is crucial for engaging listeners.
While these new systems offer great promise, there's still room for improvement. Generating truly realistic synthetic voices remains a complex undertaking. One key hurdle is replicating the subtle changes in pace, rhythm, and tone that naturally occur in human speech. For example, capturing the way someone might naturally pause or emphasize certain words, as well as how their speech speed varies depending on the situation, are aspects that are still challenging to replicate perfectly. Future developments in this area must focus on overcoming these limitations, particularly in the context of how speaking rate influences the final quality of a synthesized voice. The ability of synthetic voices to adapt to various speaking styles and speeds will be crucial for making them more natural, engaging, and ultimately more useful in diverse audio applications.
Recent advancements in voice synthesis, particularly with systems like ZSTTS2, have revolutionized the field by enabling high-quality voice cloning from surprisingly limited audio samples. This development significantly reduces the amount of training data needed, making personalized applications like audiobook narration or podcast production much more accessible. It's intriguing how these systems, relying on a concept called "few-shot learning," can quickly adapt to new voices while maintaining impressive voice fidelity. This approach contrasts sharply with older methods that demanded extensive audio samples for effective training.
ZSTTS2's architecture utilizes advanced neural networks, capable of dynamically adjusting to changes in speaking rate – a critical aspect of achieving natural-sounding cloned voices. This adaptability addresses a long-standing challenge in voice cloning technology. What's particularly interesting about ZSTTS2 is its ability to capture the subtle emotional nuances present in human speech. This is particularly advantageous for applications like audiobook storytelling or character-driven podcasts, where conveying emotion is crucial.
A key element in ZSTTS2's success is the high-quality phoneme-level annotations within its training data. These annotations allow the system to accurately learn speech timing and pacing, which are vital for producing realistic and nuanced synthetic speech. This system also paves the way for significant improvements in real-time voice synthesis applications. This is beneficial for interactive platforms like virtual assistants or customer service chatbots where immediate responses are essential.
Interestingly, ZSTTS2's efficiency can lead to a decrease in the cost of producing audiobooks. By requiring less audio data, smaller publishers can create professional-quality narrations without needing substantial resources. It's been suggested that varying the speech rate within the same audio sample can actually improve listener engagement. ZSTTS2's real-time speech rate manipulation adds a creative element to audio production that can maintain audience interest.
Beyond basic speech reproduction, ZSTTS2 can capture unique speaker characteristics like accents or dialects, even with a small amount of audio. This opens up new possibilities for creating content that resonates with diverse audiences. However, the rise of these powerful systems also raises crucial ethical considerations, particularly around consent and authenticity. As cloned voices become increasingly indistinguishable from real human voices, we need to carefully consider how we deploy this technology. The line between genuine and generated speech is becoming blurred, demanding careful oversight to ensure responsible use.
The Impact of Speaking Rate on Voice Cloning Accuracy A 2024 Analysis - CNN Models Achieve 94% Accuracy in Detecting Cloned Voices
Convolutional Neural Networks (CNNs) have recently demonstrated a remarkable ability to detect cloned voices with a 94% accuracy rate. This achievement underscores the ongoing efforts to counter potential misuse of voice cloning technology. As synthetic voices become more realistic, CNNs leverage advanced deep learning techniques to pinpoint subtle differences between genuine human speech and artificial voice clones. This ability is particularly important for applications such as audiobook production and podcasting, where maintaining authenticity is crucial for listener experience and trust. Although progress is evident in generating synthetic voices, replicating the natural flow and subtle emotional variations in speech continues to be a challenge. As the field of voice cloning develops, efforts to refine these detection models become crucial to ensure responsible use of this increasingly powerful technology in content creation.
1. **Fine-Grained Timing in Voice Cloning**: Research reveals that even subtle differences in the timing of speech, down to milliseconds between syllables, can greatly affect how natural a cloned voice sounds. Successfully replicating a speaker's voice requires algorithms that are acutely sensitive to these temporal aspects to avoid producing artificial-sounding speech.
2. **The Role of Phonemes**: The phoneme, the basic unit of sound in language, is a central element in voice cloning. However, how these sounds are represented and synthesized can change dramatically based on the speaker's style, emotional state, and speaking pace. This variability underscores the importance of having training data that includes a wide range of these factors.
3. **Emotional Nuances in Voice Cloning**: Studies show that humans significantly alter pitch and intonation during emotionally charged moments. For voice cloning to truly mimic a person's voice effectively, it needs to be able to capture these emotional fluctuations, particularly in applications like audiobooks or podcasts where listener engagement is key.
4. **Interplay of Pitch and Speaking Rate**: While speaking rate undeniably impacts voice cloning accuracy, the pitch of a person's voice is equally vital for individual identification. Understanding the relationship between pitch and speaking rate, specifically how pitch changes when someone speaks faster, poses a challenge for creating entirely authentic synthetic voices.
5. **Human Perception of Synthetic Speech**: Psychological studies indicate that human listeners are highly attuned to subtle deviations in speech. They're remarkably good at detecting when a voice is artificially generated, often at rates above 70%. Furthermore, the faster a voice, the more challenging it is for listeners to accept the synthetic nature of the sound.
6. **The Significance of Pauses**: Pauses in speech play a crucial role in conveying meaning and impacting comprehension. Voice cloning systems that struggle to naturally integrate pauses can produce output that not only sounds robotic but also hinders the clarity of the message.
7. **Training Data as a Limiting Factor**: The performance of a voice cloning system is heavily reliant on the quality of its training data. Datasets that have errors in annotation or lack sufficient variety can limit the system's capabilities, ultimately impacting the versatility of the cloned voices across diverse contexts.
8. **Adapting to Listener Feedback in Real-Time**: Emerging voice synthesis models are beginning to integrate the ability to make real-time adjustments based on listener feedback. This means that future systems might be able to dynamically alter their outputs to suit a particular application, which could significantly enhance user experiences in areas like podcasts.
9. **Evolution of Voice Cloning**: The field of voice cloning has undergone a dramatic transformation from basic waveform synthesis in the late 20th century to the highly advanced deep learning models used today. This history highlights the impressive technological advancements in understanding and replicating the complexities of human speech.
10. **Ethical Concerns of Voice Cloning**: As these systems continue to improve in producing convincing synthetic voices, ethical concerns arise about consent and the potential for misuse. The growing difficulty in distinguishing between cloned and real voices necessitates careful consideration of how this technology is used to ensure transparency and accountability.
The Impact of Speaking Rate on Voice Cloning Accuracy A 2024 Analysis - High-Quality Datasets Crucial for Improved Voice Cloning Results
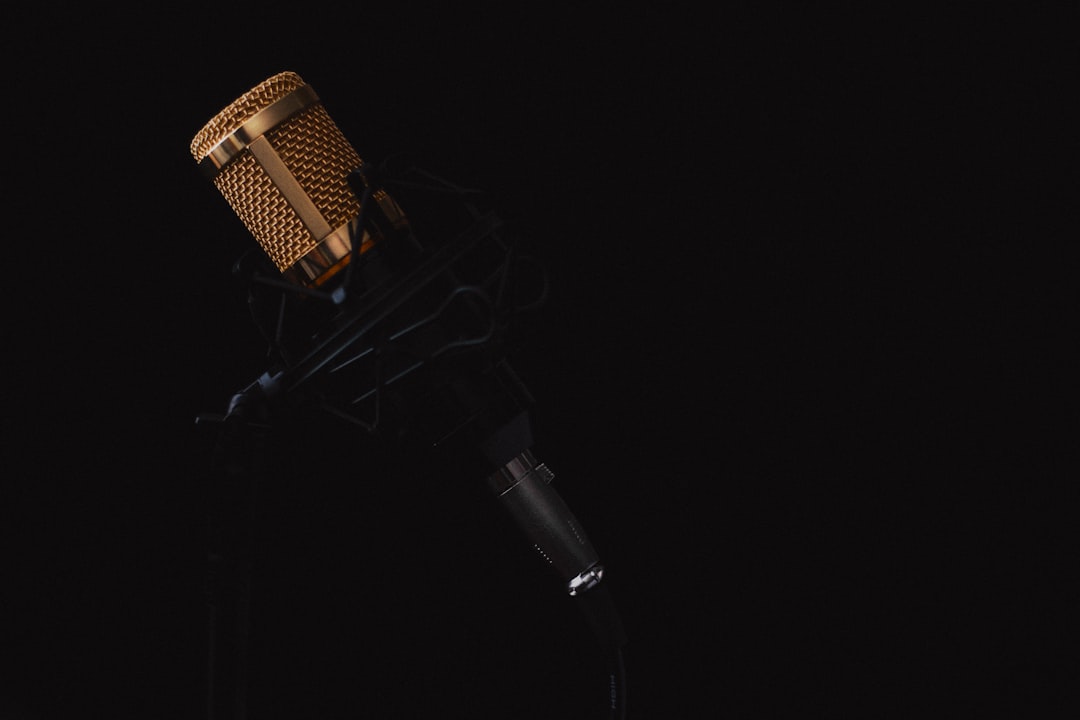
The quality of the data used to train voice cloning systems is a major determinant of how well they can recreate a human voice. Using high-quality datasets is essential for achieving realistic synthetic voices that closely mirror the original speaker's characteristics. Research suggests a clear correlation between the quality of the training data and the final output, showing that improved datasets lead to more authentic cloned voices.
This means that datasets should encompass a variety of the speaker's voice, including variations in their speaking speed and emotional expression. For example, a diverse dataset used in audiobook creation would include a speaker reading different passages at varied paces and with different emotional tones, allowing the voice cloning system to better capture the nuances of their speaking patterns.
As voice cloning finds uses in applications like audiobook and podcast production, the quality of the datasets used becomes crucial for achieving a high level of fidelity and naturalness. Without diverse and high-quality datasets, the cloned voices may sound robotic or unnatural, diminishing the listening experience.
The future of voice cloning relies on the constant refinement of training datasets to meet the increasing desire for authentic digital voices. As the technology evolves, so too must the quality and scope of the training data used. Only then will voice cloning achieve its full potential in creating engaging and natural-sounding voices for diverse applications.
The accuracy of voice cloning hinges heavily on the quality of the datasets used to train the underlying models. A diverse range of speaking rates within the dataset significantly improves the cloned voice's naturalness. When training data primarily focuses on a narrow range of speaking speeds, the resulting synthetic voices often sound artificial and fail to capture the dynamic variations inherent in human communication.
Furthermore, capturing the subtle timing nuances of speech is essential for high-fidelity voice cloning. Even slight differences in the timing between syllables, measured in milliseconds, can influence how authentic the clone sounds. This is particularly important in contexts like audiobook production, where precise timing can amplify dramatic impact.
Training data should encompass a wide range of emotional contexts, as human vocal patterns vary dramatically depending on emotional state. A robust dataset with diverse emotional representation will enable the cloning models to produce speech that mirrors the nuances of human sincerity and variability.
The way phonemes are pronounced changes depending on factors like speaking rate and emotional context. Providing the model with a variety of phonetic examples allows it to produce more convincing speech, aligning more closely with natural human vocal patterns.
Research shows that even slight pitch fluctuations, often as little as 1%, can be detected by listeners. Voice cloning models need to be trained to accurately represent these minute variations to achieve a convincingly authentic clone.
Natural speech frequently includes hesitations and filler words like "um" and "uh". Incorporating these aspects into the training datasets helps cloning systems produce outputs that sound less robotic, particularly when applied to conversational contexts such as podcasts.
Not only does speaking speed affect the accuracy of voice cloning, but it also impacts intelligibility for listeners. Datasets with a broad range of pacing can enhance how well the synthetic voice communicates meaning.
Context significantly impacts both the speed and the placement of pauses in speech. Training datasets that consider the context of the utterances allow the cloning systems to generate context-sensitive speech, contributing to greater realism and engagement, especially in audiobooks and narrative applications.
We can objectively assess voice cloning performance by comparing cloned voices to original recordings. These comparisons often reveal pronounced differences when training datasets lack diverse speaking styles, reinforcing the need for comprehensive training datasets.
As voice cloning technology advances, so do the ethical considerations surrounding its use. Ensuring datasets are acquired ethically and that the technology is implemented transparently is paramount in preventing potential misuse of these powerful tools. The ever-increasing ability to convincingly mimic human speech necessitates careful reflection on how this technology is deployed.
The Impact of Speaking Rate on Voice Cloning Accuracy A 2024 Analysis - Real-Time Speech-to-Speech Translation Advances with EchoSpeak
EchoSpeak represents a significant step forward in real-time speech-to-speech translation, showcasing the potential to bridge language barriers in a seamless manner. This technology demonstrates promise for applications like podcasting and audiobook creation, where maintaining the speaker's voice is paramount. However, its current limitations in supporting a diverse range of language pairs restricts its widespread usability.
Other methods, such as Translatotron and its refined version, Translatotron 2, also aim to translate speech directly between languages while preserving the original speaker's vocal characteristics. This is highly desirable for creating a more natural and engaging listening experience. Furthermore, research continues to grapple with simultaneous speech translation (SimulST), tackling the complexities of translating extended and uninterrupted speech in real-time.
Achieving high-quality translations while retaining the speaker's voice and mimicking the natural rhythm and nuances of human conversation remains a challenge. Future advancements in these technologies will likely need to focus on not only improving translation accuracy but also on refining the ability to seamlessly replicate the speaker's emotional tone and the natural flow of speech. This is crucial for creating truly engaging audio content across diverse platforms and genres.
1. **Speech Rate's Influence on Voice Fidelity:** Voice cloning technologies can struggle to maintain accuracy, especially when attempting to replicate faster speaking rates. This often results in an unnatural, somewhat choppy audio output, suggesting that the underlying algorithms haven't fully captured the subtle acoustic intricacies that become more pronounced at higher speeds.
2. **The Significance of Syllable Timing:** It's become clear that for truly realistic voice cloning, algorithms must account for incredibly subtle variations in the timing of each syllable—differences that can be measured in mere fractions of a second. If these timing nuances aren't precisely replicated, the cloned voice will likely sound artificial and robotic.
3. **Emotional Expression and Speech Pace:** A person's emotional state can significantly alter their speech pace, making it challenging for current cloning systems to maintain accuracy. To achieve a truly convincing emotional impact, voice cloning technologies will need to adapt dynamically to these emotion-driven variations in pace, which is crucial in applications like emotionally-driven audiobooks or character-focused podcasting.
4. **Interactive Voice Systems: A Hurdle for Real-Time Adaptation:** When it comes to applications that involve real-time interactions, like voice-activated assistants, the ability to adjust the speaking rate based on user feedback can greatly improve the user experience. However, accurately adjusting speech rates in real-time while maintaining quality continues to be a challenging area for development.
5. **Capturing Pronunciation Nuances through Phonemes:** Voice cloning that incorporates a wide range of variations in phoneme production—capturing the subtleties of how sounds are pronounced—generates far more realistic audio. These nuances are key to producing speech that authentically reflects the original speaker's distinct vocal style and characteristics.
6. **The Human Ear's Keen Detection of Synthetic Speech:** Humans are remarkably sensitive to subtle inconsistencies in speech. Studies consistently demonstrate that listeners can detect artificially generated voices at a rate of up to 70%, and this ability seems to become even more pronounced at higher speaking rates. This highlights the ongoing challenge of creating truly convincing synthetic speech.
7. **The Need for Genre-Specific Speech Pacing:** In applications like podcasting or audiobook production, the optimal speaking rate can vary considerably across different genres and content styles. Cloning systems that haven't been specifically trained on genre-related pacing may produce output that lacks the desired emotional resonance and fails to engage listeners in a meaningful way.
8. **Context and the Variability of Speech:** Human speech patterns aren't static. Our speaking rate and style are often dictated by the context of the conversation. For voice cloning to progress, it needs to integrate contextual awareness to create speech that feels relevant and consistent within a given situation or topic.
9. **The Role of Natural Speech Fillers:** The inclusion of natural speech fillers, like "um" and "uh", in training data can greatly enhance the realism of cloned voices, particularly in more conversational contexts like podcast discussions. This detail is crucial for creating outputs that sound more natural and less robotic.
10. **Ethical Implications of Voice Data Usage:** The increasing demand for diverse, high-quality training datasets raises important ethical considerations regarding consent and the appropriate use of voice data. As voice cloning becomes more sophisticated, ensuring that captured voices are used responsibly and with the speaker's consent is essential.
The Impact of Speaking Rate on Voice Cloning Accuracy A 2024 Analysis - Ethical Considerations in AI Voice Cloning Technology
AI voice cloning, with its capacity to create remarkably realistic replicas of human voices, presents a complex landscape of ethical considerations. The ability to mimic voices with such accuracy raises significant concerns about the potential for misuse, particularly in scenarios where consent is absent or where the cloned voice might be used deceptively. This includes the risk of identity theft and unauthorized impersonation, potentially leading to harm or exploitation.
Furthermore, the blurring of lines between genuine and synthetic speech necessitates a dialogue about transparency and authenticity. When encountering AI-generated voices in audiobooks, podcasts, or other content, we need to be aware of their origin and the potential for manipulation. This calls into question the role of creators and platforms in disclosing the use of these technologies.
The increasing sophistication of voice cloning technology highlights the urgent need for ethical guidelines and potentially regulatory frameworks. These guidelines would be crucial in preventing the malicious deployment of this powerful technology while ensuring its benefits, such as aiding communication for those who have lost their voice, are realized responsibly. As this technology becomes more integrated into the fabric of communication and creativity, we must actively address the ethical challenges it presents to ensure its usage remains beneficial and aligned with societal values.
The development of AI voice cloning technology, while promising for applications like audiobook production and podcasting, presents a range of ethical considerations that we, as researchers and engineers, need to examine carefully.
One key issue revolves around the inherent irregularities of human speech. Cloned voices often sound unnatural because the systems struggle to replicate hesitations, pauses, and the use of filler words. Training datasets need to be more comprehensive in representing these elements for more convincing outputs. Additionally, the impact of emotional expression on a person's voice can be a challenge for voice cloning systems. Emotional state directly affects speaking rate and vocal patterns, and replicating those nuanced changes is essential for creating a genuinely engaging listening experience in scenarios like audiobooks and narrative podcasts.
Further complicating the challenge is the uncanny ability of human listeners to detect when a voice is synthetic. Studies repeatedly show that we can identify cloned voices with surprising accuracy, often exceeding 70%. What's particularly interesting is that this accuracy seems to increase as speaking rates get faster, presenting a hurdle for creating truly indistinguishable synthetic voices.
Furthermore, voice cloning accuracy is influenced by how we pronounce individual sounds, known as phonemes. The way we say them varies with speaking rate and emotional state, highlighting the need for cloning systems to be trained on a wide range of phonetic examples to produce convincing and natural-sounding results. The precise timing of individual sounds—even fractions of a second—plays a role in vocal naturalness. For systems to reach true high-fidelity, their ability to replicate these temporal details is paramount.
Another crucial element is the quality of the training data used for voice cloning. Imperfect or limited datasets can result in clones that struggle with variable pacing and emotional expression, directly impacting the overall realism and usefulness of the synthetic voice. Encouragingly, though, newer models are starting to implement real-time adaptations based on feedback from listeners, potentially revolutionizing interactive audio applications like podcasts by making them more dynamic and responsive.
The genre of audio content also matters for voice cloning. For instance, slower speech may be ideal for educational podcasts, where comprehension is key, while fast-paced delivery might create excitement in a thriller audiobook. Cloning systems need to adapt and adjust their pace to effectively serve diverse content needs. The natural rhythm of breathing also impacts speech quality. While challenging to recreate, failing to do so can result in voices sounding robotic and artificial.
As the technology matures, the crucial ethical considerations of consent and authenticity become more pronounced. The growing capability of voice cloning to create convincing imitations emphasizes the need for clear guidelines on how to responsibly use this technology across various applications. We're entering a time where the line between genuine and artificial voices continues to blur, and thoughtfully addressing these ethical aspects will be critical for maximizing the positive potential of this technology.
Get amazing AI audio voiceovers made for long-form content such as podcasts, presentations and social media. (Get started for free)
More Posts from clonemyvoice.io: