Get amazing AI audio voiceovers made for long-form content such as podcasts, presentations and social media. (Get started for free)
The Evolving Landscape of Voice Cloning in Audiobook Production 2024 Update
The Evolving Landscape of Voice Cloning in Audiobook Production 2024 Update - AI-Driven Voice Replication Reaches New Heights in Audiobook Production
The landscape of audiobook production is undergoing a significant transformation in 2024, driven by advancements in AI-powered voice replication. Platforms are increasingly capable of creating remarkably realistic and diverse audio experiences. Services like ElevenLabs are at the forefront, offering a high level of control over voice customization, while others, such as Speechki, provide a vast library of voices spanning numerous languages. The trend towards personalized narration is also gaining traction, exemplified by platforms that enable authors to use their own voice or a cloned version, creating a more intimate connection with the listener. AI's ability to handle a wide array of genres is becoming evident with the successful generation of audiobooks from classic literary works, highlighting the technology's flexibility. The expanding range of AI tools available for audiobook creation holds promise for both increased efficiency and more immersive listening experiences. However, the broader societal impact of these developments requires careful consideration and ongoing discussion.
The field of AI-driven voice replication has seen remarkable growth within audiobook production, with several platforms demonstrating significant improvements in the realism and adaptability of generated voices. ElevenLabs stands out as a notable example, showcasing its ability to generate remarkably lifelike voices with detailed customization options. Platforms like Speechki, armed with a vast library of 1,100 voices across 80 languages, are transforming the process of turning text into audiobooks. Furthermore, platforms like AuthorVoices.ai are catering to a more personalized approach, letting users choose from cloned voices or even leverage their own voice for narration.
The capacity of these technologies to bring classic literature, like "Alice in Wonderland" and "The Picture of Dorian Gray", to life through AI narration is a testament to the breadth of applications. VEEDIO distinguishes itself by going beyond simple voice replication, integrating video editing features into its AI audiobook tools. Meanwhile, Speechify is carving a niche by offering a broad selection of natural-sounding voices across a range of languages, opening avenues for audiobooks to connect with diverse global audiences.
The landscape is rich with AI voice generators like Murf AI, which provides an extensive selection of voices and broad language support, enhancing audiobook accessibility. Real-time voice cloning is becoming more attainable, allowing users to create unique voice clones and generate personalized speech from text input. It's notable how the tools and software are proliferating, focusing on streamlining the creation process and enhancing the authenticity of the narration, creating a dynamic shift in the landscape of audiobook creation.
The progress in deep learning algorithms has notably allowed AI to not only generate speech, but to dynamically adjust the emotional tone of the narration based on the content, potentially enriching storytelling through more nuanced voice acting. These developments are intriguing but raise questions about the role of human narrators and whether the growing accessibility of AI-powered voice cloning will blur the lines between human and machine narration. The legal and ethical concerns are growing alongside the technology; questions surrounding intellectual property and the potential for unauthorized use of voices are surfacing. It will be interesting to see how the industry evolves in navigating these challenges in the coming years.
The Evolving Landscape of Voice Cloning in Audiobook Production 2024 Update - Streamlining the Production Process with Synthetic Voice Technology
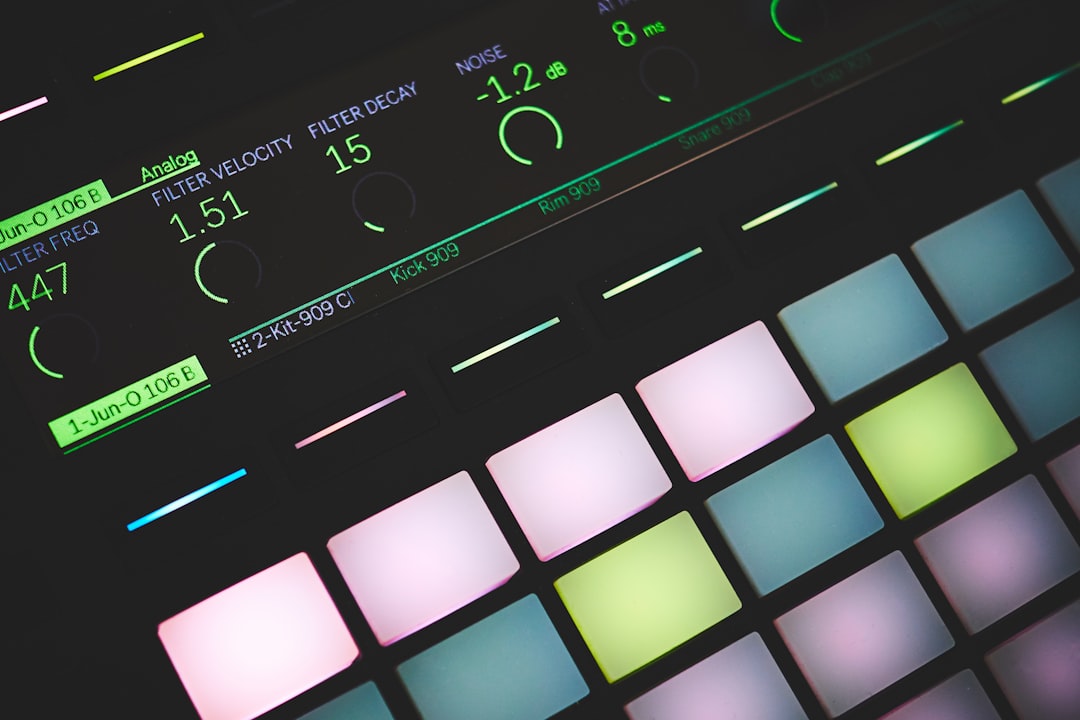
Synthetic voice technology is rapidly changing how audiobooks are produced, making the process smoother and more creative. The ability to recreate voices using just a few audio samples is speeding up production timelines and reducing the need for traditional voice actors. Modern Text-to-Speech (TTS) systems now create speech that sounds much more natural and expressive, leading to a better listening experience for audiobook fans. The possibilities for unique and diverse audio content seem endless as AI improves, though this progress raises important ethical concerns around authenticity and the rights associated with using voices. While synthetic voices are pushing audiobook production forward, the industry must carefully consider the balance between innovation and ethical responsibility.
The field of AI-driven voice synthesis is rapidly evolving, leading to some intriguing advancements within audiobook production. For example, deep learning models are becoming increasingly adept at analyzing the emotional undertones of written text and adjusting the synthetic voice's delivery accordingly. This dynamic adaptation of tone and pacing offers the potential for a more nuanced and engaging listening experience, adding another layer to the art of storytelling.
Furthermore, the accuracy of voice cloning technology has reached remarkable heights. Some systems consistently achieve a mean opinion score (MOS) of 4.5 or higher, suggesting that many listeners perceive little difference between AI-generated and human narration in terms of quality and realism. This leap forward raises the bar for what can be considered a high-quality audiobook production.
Moreover, the capacity to seamlessly transition between languages within a single audiobook opens new opportunities for accessibility. With AI, audiobooks can cater to multilingual audiences, breaking down language barriers and reaching a much broader range of readers. Real-time voice cloning technologies are also emerging, which empowers narrators to create personalized voice models with significantly less effort and time than traditional methods. Instead of lengthy editing processes, authors can now potentially generate unique voice clones within a few hours.
In terms of customization, there's a burgeoning trend toward greater control over specific phonetic elements. AI platforms allow users to fine-tune accents, pitch, and speech pace, providing greater creative agency in how they want their story to be delivered. Voice transfer learning techniques have also seen promising advancements. AI systems now can adapt and build voice models using a limited number of samples, making the cloning process more efficient and requiring less source material. This opens the door for creating high-quality voices with significantly reduced recording time and effort.
Some innovators are even experimenting with the integration of voice cloning and visual media. Companies like VEEDIO are developing platforms that allow users to combine audiobooks with synchronized visuals like animations or illustrations, promising a richer and more interactive audiobook experience. However, this increased realism brings forth questions about authenticity. The boundary between human and AI-generated narration is becoming increasingly blurred, leading to questions about the role of human narrators and the impact on listener's perception of the storyteller and their connection to the content.
The speed of audiobook production is also significantly accelerating thanks to AI. In some cases, what previously took weeks is now achievable in a matter of days. This rapid development allows authors to capitalize on market trends and potentially react to seasonal demands more effectively. Additionally, some systems are being designed to incorporate listener feedback. The idea is that as listeners interact with audiobooks and provide feedback, the AI systems can learn and adapt, continuously refining the quality and suitability of the generated voices. This constant feedback loop is likely to further improve the library of available AI voices over time and make them more responsive to listener preferences.
The Evolving Landscape of Voice Cloning in Audiobook Production 2024 Update - Minimal Sample Requirements for Accurate Voice Cloning
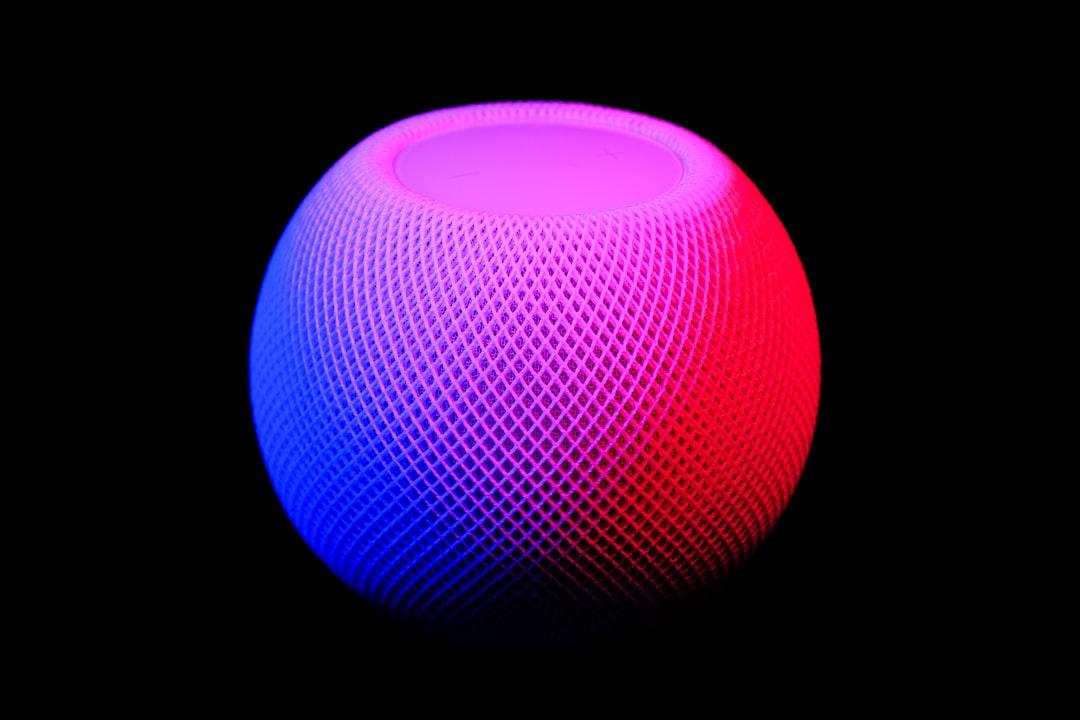
The field of voice cloning is experiencing rapid advancements, leading to a better understanding of the minimal audio needed for accurate voice replication in audiobook production. Achieving professional-grade synthetic voice generation generally requires a minimum of 30 minutes of high-quality audio featuring a single speaker. This emphasizes that quality audio is paramount for optimal results. The recordings should be devoid of background noise and other audio distortions to ensure the AI model can accurately capture and replicate the unique vocal traits of the speaker. While achieving remarkable results with relatively short audio samples is impressive, the increasing ability to create near-perfect voice clones raises concerns about authenticity and ethical implications. As this technology progresses, it's essential to have an open discussion on the responsible use of AI-generated voices and the impact this has on listeners and the industry as a whole. The evolving relationship between innovation and the ethical considerations involved will continue to shape how voice cloning technology is implemented in audiobook production.
### Minimal Sample Requirements for Accurate Voice Cloning: Surprising Insights
It's remarkable how advancements in voice cloning have allowed for accurate vocal reproduction using surprisingly few audio samples. We've seen that sometimes just a brief snippet of audio, perhaps 10 to 30 seconds, can be enough to generate a basic voice clone. This suggests the variety within the captured samples might be more important than simply the total duration.
The success of voice cloning appears strongly linked to the phonetic diversity within the sample set. A concise dataset encompassing a wide range of pronunciations, emotional tones, and speech rhythms often produces a more adaptable and realistic voice model compared to a larger but more homogeneous dataset.
However, the circumstances surrounding the recording are crucial. The recording environment, the quality of the microphone, and even the speaker's physical state can significantly influence how well the voice clone is generated. Unexpected variations in these aspects can lead to less believable synthetic voices.
The temporal resolution of the audio samples also seems to matter. High-quality samples enable more precise adjustments to pitch and inflection during the synthesis process, leading to a more natural-sounding outcome. This is particularly important for applications where subtle nuances in vocal delivery are important, like audiobook narration.
Interestingly, voice cloning is showing promise in bridging language barriers. Recent research suggests that we can successfully create voice clones that function across different languages using the same minimal set of samples. This implies that shared phonetic and tonal properties can transcend linguistic differences, opening up opportunities for a wider global audience.
Different voice cloning algorithms handle minimal sample datasets in unique ways. Generative adversarial networks (GANs), for example, have demonstrated a strong capacity for creating realistic voice clones from limited input, suggesting a promising future for their application in audiobook production.
The ability to integrate listener feedback directly into the voice cloning process offers an intriguing opportunity to continually refine voice models. By incorporating constructive criticism back into the system, we can improve future voice cloning outcomes, even starting with minimal initial audio.
The ability to adapt a voice to different accents using just a few samples is an exciting development. Voice cloning systems can leverage brief snippets of a target accent to significantly enhance their capability to imitate and reproduce speech in that particular accent.
Furthermore, the emotional richness of synthetic speech can be surprisingly robust even with limited data. Modern AI algorithms are becoming quite adept at extrapolating emotional undertones from context, resulting in variations in vocal inflection that add a layer of depth to audiobook narration.
The reduced sample size needed for effective voice cloning is already impacting fields like podcasting. Creators can now personalize their voice or even create custom voice clones for guests using just a few minutes of recorded audio. This streamlined approach greatly reduces production time without necessarily compromising the quality of the final audio. This is a significant development that could lead to more creative and efficient methods of producing engaging audio content.
The Evolving Landscape of Voice Cloning in Audiobook Production 2024 Update - Time and Resource Optimization in Audio Content Creation
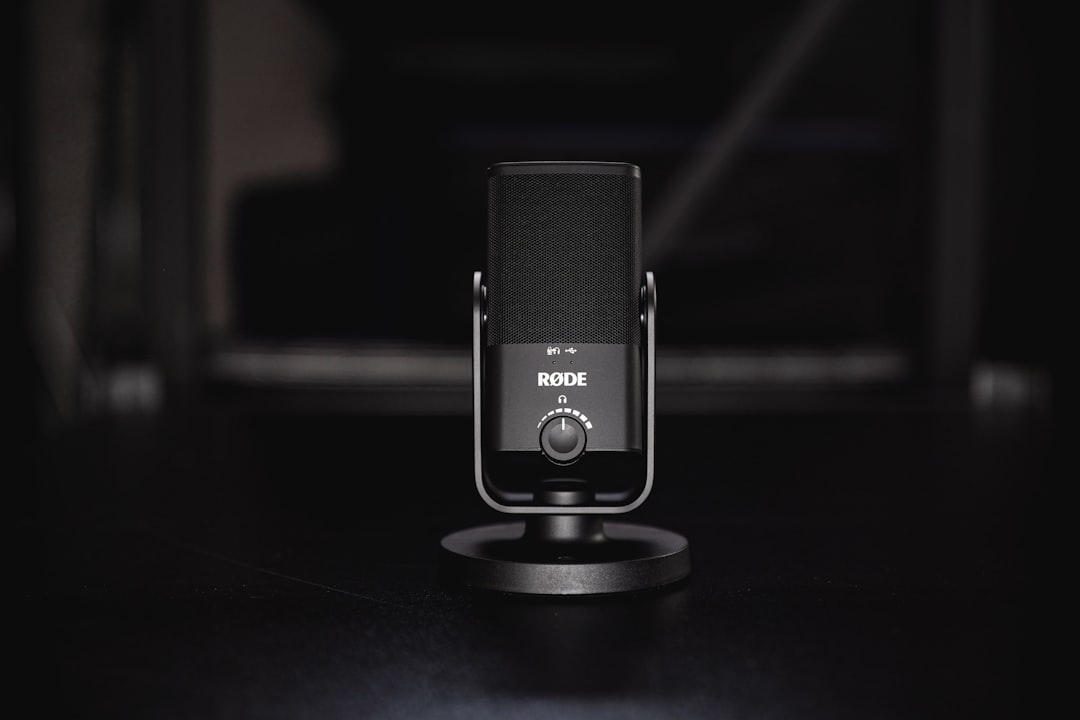
The creation of audio content, including audiobooks and podcasts, is experiencing a surge in efficiency thanks to advancements in technologies like voice cloning. These innovations allow creators to produce high-quality audio using a smaller amount of source material, streamlining workflows and reducing production time. This efficiency extends beyond simply faster completion of projects; it enables a new level of personalization, such as letting authors narrate their own audiobooks using a cloned voice or creating distinctive voices for podcast characters. While the technological improvements are exciting, they also bring up valid concerns about the authenticity of the generated audio and the ethical implications of replicating voices. The industry is challenged to find a balance between promoting innovation and ensuring the responsible use of these capabilities. Despite these challenges, the future of audio content is promising, with voice cloning paving the way for more diverse and engaging content. However, it's crucial to acknowledge the broader societal impact of these advancements and address the questions they raise in a thoughtful and proactive manner.
Within the realm of audio content creation, particularly audiobook production, the evolution of voice cloning technology has brought about remarkable efficiency. Using advanced systems, engineers can now generate a professional-quality synthetic voice from surprisingly brief snippets of audio, potentially as little as 10 to 30 seconds. The key to this efficiency seems to lie in the variability of the audio sample rather than its overall duration. It's an interesting finding that suggests the breadth of vocal expressions within a short sample set may be more critical than its length for generating a convincing voice clone.
The importance of phonetic diversity within the voice sample data cannot be overstated. A wider range of pronunciations and emotional tones packed into a short snippet frequently produces a more adaptable and realistic voice model compared to a larger, more homogenous dataset. This is especially valuable for applications like audiobooks where nuanced emotional delivery can greatly enhance the narrative experience.
The role of algorithms like Generative Adversarial Networks (GANs) in this process is exciting. They’ve demonstrated a remarkable capacity to synthesize realistic voice clones from minimal audio input. While still developing, these technologies have the potential to fundamentally alter how we create and consume audio content.
Audiobooks are now seeing significant reductions in production time. Tasks that could take weeks in the past can now be completed in days, or even hours in certain instances. This remarkable increase in speed allows authors to respond more quickly to market shifts, potentially capitalizing on trends or seasonal changes.
The capacity of AI systems to render a wide spectrum of emotions is impressive. Modern synthetic voices can now subtly adjust pitch and inflection, convincingly conveying emotion. This development is especially significant in areas like narration, where emotional depth was previously considered the sole domain of human voice actors.
Techniques like voice transfer learning are making it possible to fine-tune voice models with fewer audio samples. This enhances the overall efficiency of voice cloning, allowing for high-quality replication with significantly less recording effort.
One of the more remarkable outcomes of this research is the development of multilingual audiobooks using a single voice model. By capitalizing on shared phonetic features across languages, synthetic voices can reach a wider audience without the need for separate recordings for each language. This represents a significant step forward for audio accessibility.
The potential for real-time adaptation is another exciting element of this technology. By incorporating listener feedback into the production pipeline, audio engineers can refine and adjust synthetic voices in response to audience preferences. This adaptive approach allows for continuous improvements in voice quality with relatively quick development cycles.
It's also important to acknowledge that the quality of the recording environment significantly affects the accuracy of the voice cloning process. Aspects like microphone quality and the presence of background noise can dramatically impact the fidelity of the resulting synthetic voice. It highlights the importance of high-quality recording environments for optimal results.
Finally, this emerging technology is reshaping the podcasting landscape as well. Creators are starting to utilize it to generate unique voice clones for guests, which enables them to produce more personalized audio content. This process requires only a few minutes of recorded audio and streamlines content creation, but simultaneously questions our traditional understanding of voice authenticity in the context of audio storytelling. The ramifications of this development in the field of audio content are likely to be far-reaching and warrant continued research.
The Evolving Landscape of Voice Cloning in Audiobook Production 2024 Update - Addressing Representation and Bias in Voice Models
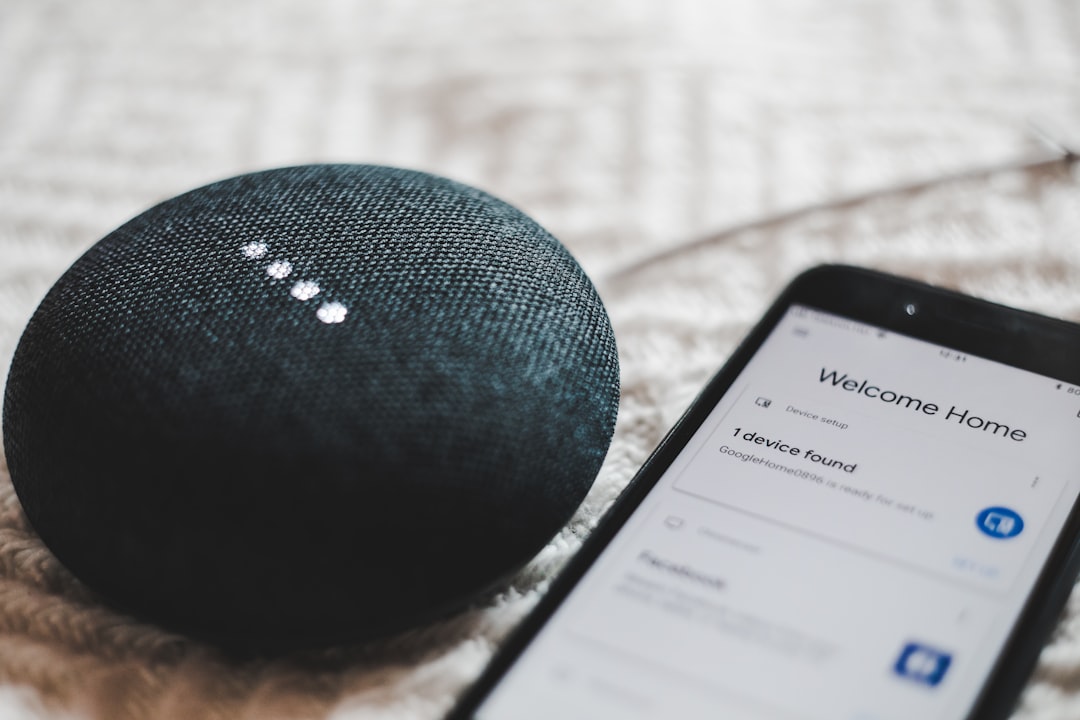
The surge in AI-powered voice cloning within audiobook production brings into sharp focus the crucial issue of representation and bias embedded within these synthetic voice models. As these technologies become more prevalent in delivering audiobooks to diverse listeners, it's increasingly clear that there's a significant gap in the languages and dialects represented. The fact that a very small percentage of AI models cater to the world's most common languages highlights a troubling lack of inclusivity. This limited representation can inadvertently reinforce existing biases and restrict access to audiobooks for a wide range of audiences. Moving forward, it's vital for the audiobook industry to prioritize ethical considerations around the diverse nature of human voices and ensure that voice cloning and AI-related technologies aren't contributing to these imbalances. Open and ongoing discussions, along with decisive steps towards fostering fair representation, are needed to guide the responsible development of voice technology within the audio space. Ultimately, the goal is to ensure that these innovations benefit and serve all listeners in an equitable manner.
The development of voice models, while exciting, presents some concerning challenges related to representation and bias. A significant issue is the underrepresentation of certain accents, dialects, and voices from marginalized communities. Many models are primarily trained on data from specific regions or demographics, leading to a skewed and often inaccurate portrayal of how people speak. This can be particularly problematic when we consider the varying perceptions listeners have based on their own backgrounds and experiences. For example, listeners from diverse linguistic groups might judge the quality and authenticity of a synthetic voice differently, reflecting existing biases within our auditory experiences.
Beyond simply accuracy, there's the issue of cultural nuance. Voice models often fail to fully grasp the rich tapestry of cultural expression found in language—things like idioms, slang, and the subtleties embedded in speech patterns unique to various groups. This can lead to misunderstandings and misinterpretations, especially in audiobooks aimed at broad audiences. A similar problem exists with gender bias in many synthesis databases. A large number of voices are skewed toward a single gender, potentially influencing listener perceptions of authority and trustworthiness based on the voice they hear.
This issue of bias isn't solely a matter of the datasets used for training. The quality of those datasets themselves can introduce issues. Poorly curated datasets can inadvertently perpetuate stereotypes or negative portrayals linked to certain demographics, which further reinforces potentially harmful narrative structures in the content. The lack of capacity in many voice models to accurately reproduce regional accents not only reduces authenticity but isolates listeners who may not feel represented by the technology. This again highlights the need for inclusive voice technologies.
Furthermore, there's an interesting dynamic with listener preferences. Studies show a strong bias toward voices that resonate on an emotional level with individuals. This reinforces the need to ensure a wide range of emotional expression in our voice models to cater to the diverse needs of the audience. The potential for abuse through voice cloning introduces significant ethical and legal hurdles. Using a person's voice without their consent, especially when it reinforces harmful biases, is ethically questionable and could lead to legal battles and public disapproval.
The issue of bias can be further compounded by the way AI learns. If a model is trained on a biased dataset, those biases can be reinforced through feedback loops. As the AI interacts with users and receives feedback, it might simply learn to strengthen the existing biases in the data. This underscores the importance of constantly monitoring and updating training datasets to prevent the escalation of bias.
Thankfully, the field is progressing. Emerging voice synthesis technologies now allow for real-time adjustments to voice attributes. This could be a powerful tool for audiobook and podcast creators, potentially enabling them to fine-tune voice characteristics on-the-fly and create more inclusive and balanced narrative experiences. The field of voice cloning is clearly evolving quickly, and understanding the potential impact of these technologies, especially on diversity and inclusion, is crucial for responsible development and application.
The Evolving Landscape of Voice Cloning in Audiobook Production 2024 Update - Navigating the Evolving Regulatory Landscape of Voice Cloning
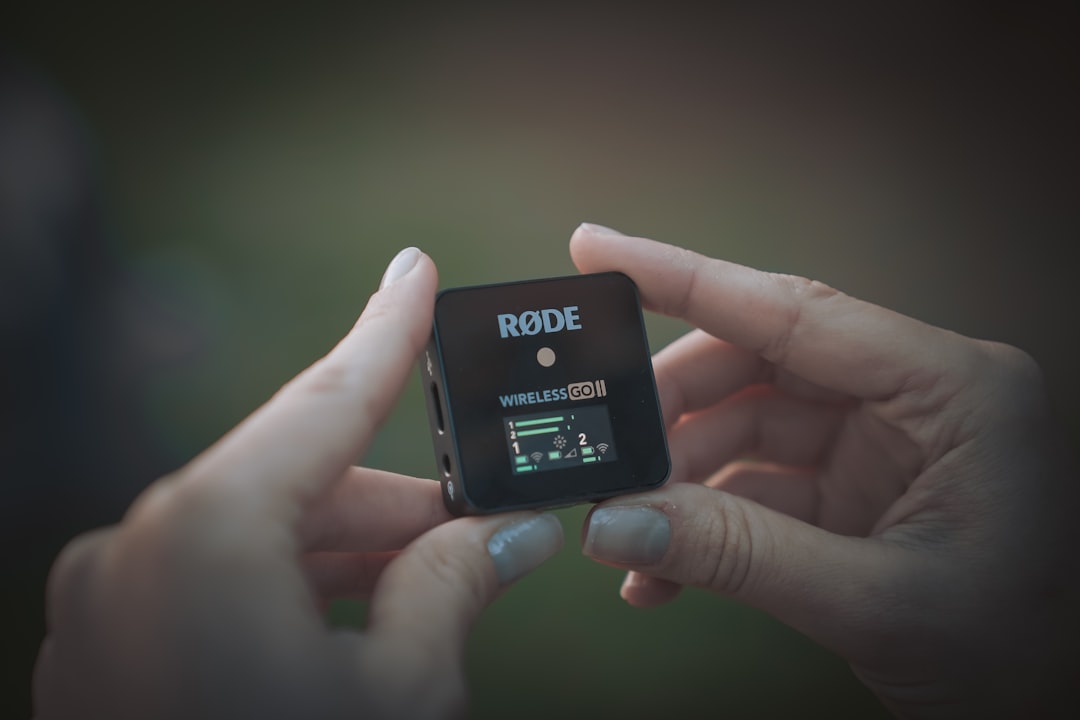
The rapid advancements in voice cloning technology are prompting a shift in the regulatory landscape. Organizations are increasingly concerned about the potential for misuse, particularly the creation of convincingly realistic human voices that could be used deceptively. This has led to initiatives focused on establishing guidelines and regulations for the development and deployment of AI-powered voice synthesis. The goal is to strike a balance between promoting innovation in fields like audiobook production and podcasting, and protecting consumers from potential harm.
This evolving regulatory environment brings into sharper focus the ethical considerations associated with voice cloning. Creators using these technologies have a responsibility to be transparent about their use of synthetic voices, and to ensure they obtain proper consent when utilizing individuals' voices. The conversation surrounding voice cloning necessitates a careful balancing act—one that fosters technological advancements while mitigating the risks of deception and misrepresentation. The challenge lies in ensuring that the benefits of this powerful technology are realized responsibly, protecting the authenticity and integrity of audio content while advancing its potential.
The field of voice cloning is rapidly evolving, with technologies capable of analyzing written text to dynamically adjust the emotional tone of narration. This dynamic emotion modulation creates a more immersive listening experience, mimicking the subtle delivery styles of human narrators, potentially enriching the storytelling aspect of audiobooks. Interestingly, the success of this technology doesn't always depend on the length of the audio sample. Instead, it seems that capturing phonetic richness within a short snippet is more crucial. High-quality samples with a diversity of pronunciations and emotional tones can yield remarkably realistic voice models, even from as little as 10 to 30 seconds of recorded audio.
However, voice cloning technology still struggles with accurately capturing cultural nuances. Idioms, slang, and the subtleties of various speech patterns can be lost in translation. This raises concerns about accurately representing the richness of diverse cultural expressions within audiobooks, which could lead to misunderstandings or misinterpretations. Additionally, the training data used to create these voice models often underrepresents certain accents and dialects, skewing the portrayal of speech patterns. This bias can alienate certain listener demographics, as it might inadvertently perpetuate harmful stereotypes in audio storytelling.
Fortunately, new developments allow for real-time adjustments to voice characteristics during narration. Content creators can now make on-the-fly changes to tone, pitch, or accent, which promotes greater inclusivity and responsiveness to audience preferences. Furthermore, these technologies are also beginning to bridge language barriers by identifying shared phonetic and tonal elements across different languages. This capability enables the creation of multilingual audiobooks using a single voice model, expanding accessibility for global audiences.
The use of Generative Adversarial Networks (GANs) in voice cloning has also been particularly promising. They demonstrate a remarkable ability to synthesize high-quality voice models from minimal audio input, which has the potential to significantly reduce the time and resources typically involved in audio production. The success of synthetic voices, however, is heavily influenced by listener perception. Studies show that listeners tend to have strong preferences for specific vocal characteristics, suggesting that emotional resonance plays a major role in how people evaluate the quality of audiobook narration.
The quality of the recording environment also plays a crucial role in the fidelity of the synthetic voice. Factors like background noise, microphone quality, and the speaker's physical state can all significantly influence the outcome of the voice cloning process. This highlights the importance of capturing audio in well-controlled settings. It's also worth noting that the iterative nature of AI learning can inadvertently lead to bias reinforcement. If training data contains biases, those biases can be further amplified through user interactions and feedback loops. This dynamic underscores the need for continuous evaluation and refinement of datasets to mitigate the influence of bias in synthetic voices. Overall, as this technology continues to evolve, understanding the potential impact on diversity and inclusion is essential for the responsible development and application of voice cloning within the audiobook industry.
Get amazing AI audio voiceovers made for long-form content such as podcasts, presentations and social media. (Get started for free)
More Posts from clonemyvoice.io: