Get amazing AI audio voiceovers made for long-form content such as podcasts, presentations and social media. (Get started for free)
The Evolution of Voice Cloning From Parody to Professional Production in 2024
The Evolution of Voice Cloning From Parody to Professional Production in 2024 - From Berkeley to Bedroom Studios The Birth of Voice Cloning in 1998
The seeds of voice cloning were sown in 1998 at the University of California, Berkeley, a moment that marked a turning point in the realm of audio technology. These early efforts, while rudimentary, concentrated on the fundamental task of replicating voices, establishing the foundation for a technology that would revolutionize sound design and content creation. The ensuing years witnessed a steady progression, fueled by the rapid advancements in machine learning and natural language processing. Voice cloning gradually shifted from its initial, often playful, uses in parodies to a more serious and professional role within various audio production realms. Today's voice cloning systems possess a sophisticated ability to capture the subtle nuances of a speaker's voice, allowing creators to generate synthetic voices that bear a striking resemblance to the originals. This has empowered independent producers, podcasters, and others to leverage these tools for enhancing their projects and streamlining workflows. As this technology continues to mature, it presents both thrilling possibilities for creativity and prompts crucial reflections on the concepts of authorship and the ever-evolving role of voice in narratives and audio experiences across the multimedia spectrum.
The genesis of voice cloning can be traced back to 1998, when researchers at the University of California, Berkeley, pioneered concatenative synthesis. This approach essentially involved recording and stitching together fragments of human speech to generate relatively natural-sounding voices. Early experiments were quite demanding, requiring extensive speech databases and careful phonetic balancing to maintain consistency in the synthetic voice's tone across different phrases and contexts.
Interestingly, these initial efforts relied heavily on mathematical models to mimic human speech patterns, rather than the machine learning methods we see today. This period also marked an unusual partnership between university researchers and tech companies, demonstrating the power of interdisciplinary collaboration in pushing technological boundaries.
The immediate impact was felt in audiobook production, where voice cloning offered a faster and more affordable approach. However, concerns arose about the emotional impact and authenticity of synthetic voices, which were far from flawless at that stage. These early experiments typically occurred in controlled laboratory and studio environments, prioritizing pristine audio fidelity. This strict focus on high-quality output established a high benchmark for subsequent efforts aimed at developing consumer-grade voice cloning applications.
In its early years, voice cloning was mostly considered a curious novelty. This led to a certain paradox where the technology's capabilities were clear, yet its acceptance into professional audio production workflows was initially slow. The progress made in 1998 kicked off a fervent pursuit to refine synthesis techniques within both research labs and commercial settings. This ultimately shaped the production and consumption of podcasts and other audio content in later years.
Public reception to voice cloning was initially diverse, with some enthralled by the novelty and others worried about the possibility of creating deceptive audio. The core technologies developed in 1998, however, laid the foundation for today's sophisticated neural network approaches. It's a fascinating example of how earlier, seemingly simpler breakthroughs can eventually become the foundational elements for more complex systems that continue to reshape audio production and editing practices.
The Evolution of Voice Cloning From Parody to Professional Production in 2024 - Early Struggles Capturing Speech Nuances in Voice Replication
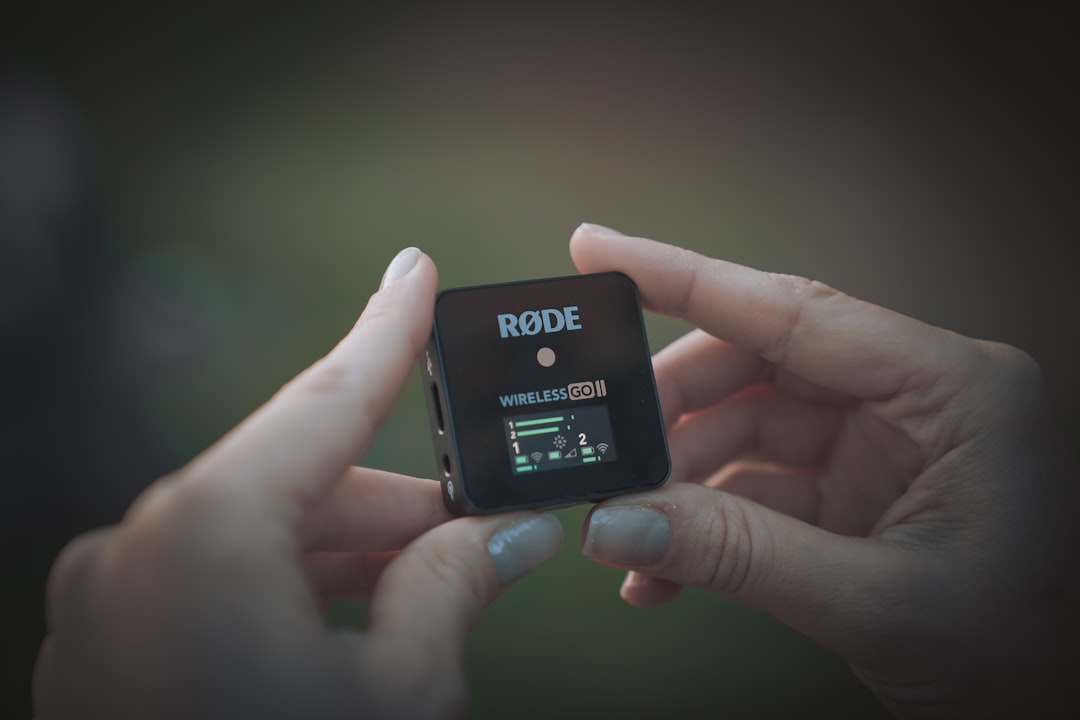
The early stages of voice replication faced significant hurdles in accurately capturing the subtle nuances that characterize human speech. Initial efforts, relying heavily on piecing together pre-recorded speech fragments, often resulted in synthetic voices that sounded robotic and lacked the emotional depth and variability of authentic human communication. These early systems, while demonstrating the potential of voice cloning, struggled to reproduce the subtle inflections, pauses, and emotional cues that make human speech so engaging. This limitation stemmed from the technology's dependence on large datasets of pre-recorded speech and a rigid adherence to specific phonetic structures, which restricted the natural flow and expressiveness of the generated voice.
Despite the progress driven by machine learning and deep learning approaches, the challenge of replicating authentic human vocal expression continues to be a central focus. Achieving a truly natural-sounding voice, especially in applications demanding emotional depth, such as audiobooks or podcast narration, remains a difficult task. This constant pursuit of improvement highlights the crucial need for continued development and refinement in this field, particularly as concerns about authenticity and the perceived emotional connection fostered by a voice become increasingly relevant. The quest for truly natural and emotionally expressive synthetic voices is an ongoing journey, pushing the boundaries of what's possible in audio production.
Early attempts at capturing the nuances of human speech in voice replication faced significant challenges. One hurdle was the sheer complexity of phonetic balancing. A single language can have up to a thousand distinct phonemes, each needing careful consideration in how they interact within different contexts. Achieving natural-sounding speech required a deep understanding of these intricate sound relationships.
Furthermore, early voice cloning struggled to replicate the subtle emotional cues that make human communication engaging. Synthesized voices often lacked the expressiveness of a real person, sounding robotic and devoid of natural tone and emphasis. This was a significant limitation in applications like audiobooks, where conveying emotion is vital for storytelling.
The very mechanics of voice production, relying on the vibration of vocal folds, also presented a challenge. Mimicking these intricate biological processes with accuracy was a significant hurdle for early synthesis efforts. Engineers had to develop methods that could faithfully reproduce the nuanced effects of vocal fold length, tension, and shape on the resulting sound.
The fidelity of early synthesized speech was often limited by the granularity of the audio waveforms. This resulted in noticeable, unnatural artifacts, creating a discernible difference between human and artificial speech. Moving from methods like concatenative synthesis to waveforms with finer detail significantly improved the quality of synthetic voices.
Early models also found it difficult to capture the diversity of human speech, particularly with regional dialects and accents. This inability to accurately reproduce diverse speaking styles hindered the broader adoption of these systems, as they struggled to adapt to different cultural contexts.
In addition, the critical role of natural language processing (NLP) in voice cloning was initially underestimated. Later advancements incorporated NLP techniques to provide context and nuanced inflections to synthetic speech, greatly enhancing the narrative quality of audio productions. Without this improved understanding of language, the resulting voices felt disjointed and lacked the flow of human conversation.
The substantial size of speech databases required by early models presented a major logistical challenge. Some required as much as 100 hours of recorded speech from a single individual to build an accurate representation of their voice. The collection and management of such datasets proved to be costly and time-consuming.
Likewise, subtle aspects of language, such as the rhythm and emphasis of speech (prosody) were often missed in early models. Researchers discovered that incorporating these details was essential for increasing the authenticity and listener engagement of synthetic voices. It became clear that factors like stress patterns play a key role in the overall quality of synthetic speech across a range of applications, including podcasts and audiobooks.
The reception to early synthetic voices was mixed. Many listeners found the mechanical quality of the speech jarring and rejected it as unrealistic. This resistance fueled a drive to make artificial voices more appealing and acceptable to a broader audience, driving further refinements in the development of these technologies.
Finally, voice replication benefited greatly from integrating insights from fields like linguistics and cognitive science. This interdisciplinary collaboration provided a deeper understanding of human communication patterns and the complexities of language. The exchange of knowledge across these diverse fields helped build models that more effectively replicated the intricate aspects of human speech, accelerating the maturation of the field.
The Evolution of Voice Cloning From Parody to Professional Production in 2024 - Machine Learning Breakthroughs in Natural Language Processing
The intersection of machine learning and natural language processing (NLP) has profoundly impacted the realm of voice cloning. Recent breakthroughs in deep learning, particularly the emergence of large language models, have empowered the creation of synthetic voices that are increasingly sophisticated and human-like. This has allowed for a more nuanced capture of emotional expression and even the reproduction of regional accents within generated speech. These advancements have proven particularly valuable in audiobook production and podcasting, where the ability to convey a sense of authenticity and emotional depth is vital for audience engagement.
Despite these noteworthy strides, achieving a completely natural-sounding synthetic voice that mirrors the complexity of human speech remains an ongoing pursuit. While voice cloning has matured considerably, replicating the subtle nuances of human vocal expression—the pauses, inflections, and subtle changes in tone—remains challenging. This inherent complexity continues to drive further research and development within the field, aiming to bridge the gap between synthesized and naturally occurring speech. As voice cloning continues to evolve and expand its capabilities, its influence on storytelling and communication through sound is becoming increasingly significant. This ongoing evolution represents a fascinating interplay between technological advancement and the creative potential of sound design and audio production.
The field of Natural Language Processing (NLP) has experienced a remarkable transformation, largely driven by machine learning breakthroughs, particularly in the realm of voice cloning. The advent of transformer architectures like BERT and GPT has revolutionized NLP, granting AI models a deeper understanding of context and a newfound ability to generate truly coherent text. This has proven invaluable for voice cloning, as it allows for more lifelike and nuanced dialogue within synthesized speech, an essential element for high-quality audio productions like podcasts and audiobooks.
Moreover, a shift towards end-to-end learning has streamlined the process. Rather than needing painstaking manual extraction of phonemes, modern systems can directly learn from raw audio inputs. This approach significantly improves the subtle details captured in the synthesized voice, minimizing the often-criticized robotic qualities that plagued earlier generations of voice cloning.
Another interesting development is the growing integration of emotion recognition into these systems. Not only can they replicate voices now, but they can also imbue them with a range of emotional cues. By analyzing variations in speech patterns and tone, these models can produce voices expressing happiness, sadness, or urgency, significantly enriching the storytelling potential within audiobooks and podcast narratives.
The need for massive datasets in the past has also been addressed with improved speaker adaptation techniques. Voice cloning models can now adjust quickly to new speakers, requiring only minimal data. This increased adaptability is a substantial step forward, allowing more people to utilize the technology without being constrained by a requirement for extensive voice recordings.
However, the importance of data diversity cannot be overstated. Ensuring that the datasets used to train these models represent a wide spectrum of accents, dialects, and emotional expressions is crucial for building truly inclusive technologies. Failing to address this can lead to unintended bias, where dominant speech patterns are favored, creating an uneven playing field in audio content creation.
Further bolstering the quality of synthetic speech are the significant advancements in waveform synthesis techniques like WaveNet and neural vocoders. The result is a marked increase in audio fidelity, reducing the discernable differences between human and artificial voices. This translates to a smoother and more enjoyable listening experience for individuals consuming audiobooks or interacting with virtual assistants.
Additionally, evaluation methods for synthesized speech have matured. Earlier, rather rudimentary metrics have given way to comprehensive assessments incorporating listener perception. Crowdsourced feedback plays a significant role in ranking the naturalness and emotional engagement of synthetic voices, a necessity for applications emphasizing immersive storytelling, like cinema or narrative-focused podcasts.
Beyond simple replication, today's voice cloning systems offer a new degree of control over the voice's characteristics, allowing for adjustments to pitch, tone, and even speech speed. This opens up opportunities for content creators to fine-tune synthetic voices to align with specific emotional contexts or audience preferences, further enriching the personalization of audio content.
The power of embeddings and latent space representations has also been harnessed to manipulate and blend various voice characteristics in innovative ways. This capability allows for the merging of features from multiple voices to craft unique and adaptable synthetic voices, potentially leading to more compelling and versatile audio projects.
Finally, the development of cross-lingual capabilities within voice cloning is exciting. Single models can now generate speech in multiple languages, heralding a new wave of globalization for podcasts and audiobooks. This advancement fosters greater accessibility and inclusion by allowing creators to reach wider audiences without having to create and maintain separate models for each language.
The intersection of NLP and voice cloning is fostering an exciting era of audio production, one filled with both tremendous potential and some important ethical considerations. The continued development of these technologies promises to change how we interact with audio content, offering new ways to tell stories and communicate across the globe.
The Evolution of Voice Cloning From Parody to Professional Production in 2024 - 30-Minute Recordings The New Standard for Professional Cloning
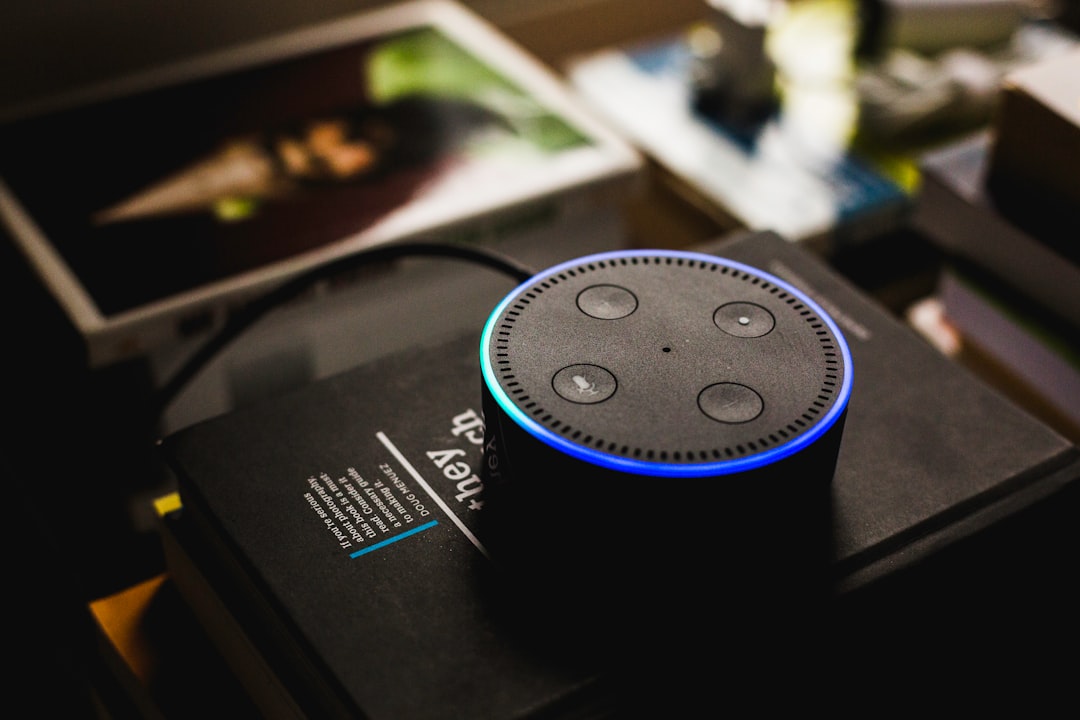
The evolution of voice cloning has brought about a new standard for professional applications: the requirement of 30-minute audio recordings. This shift signifies a growing emphasis on capturing the intricate details of a speaker's voice, allowing for a more accurate and nuanced replication. Previously, simpler methods could generate voices using short audio snippets, but the demand for higher quality in areas like audiobook production and podcasting has driven a need for richer datasets. These longer recordings give machine learning algorithms more data to analyze the unique characteristics of a voice, resulting in a more authentic synthetic output. However, even with this advancement, challenges remain in truly capturing the subtle nuances of human speech, like emotional delivery and natural pauses. While the technology has shown great promise in producing high-fidelity voice clones, it's important to examine the potential ethical implications and the effect on both storytelling and the very art of voice acting in a world where synthetic voices are becoming increasingly sophisticated. This push towards a 30-minute standard raises important questions about the future of voice in content creation.
The shift towards 30-minute recordings as the new standard for professional voice cloning is intriguing. It seems to address some of the limitations we've encountered in the past with creating truly natural-sounding synthetic voices. By capturing a more extensive sample of a person's speech patterns within a relatively manageable timeframe, we can potentially enhance the quality of synthesized voices in diverse applications. For instance, in audiobook production or podcast narration, this could translate to a more seamless and emotionally resonant experience for listeners.
One area where this change is notable is the refinement of voice characteristics. We've seen research suggest that subtle emotional cues embedded within a voice—elements like pitch, tone, and speech speed—have a significant impact on audience engagement. With 30-minute recordings, voice engineers have a broader canvas to carefully adjust these subtle features, potentially creating more immersive and impactful experiences.
This approach also seems to address the efficiency of machine learning in voice cloning. Although recent advancements allow for cloning with very limited audio snippets, professional quality requires a broader set of data points. This new standard allows for the capture of more diverse speech patterns within a relatively limited time commitment, potentially reducing the size of the necessary audio datasets compared to older methods.
Furthermore, capturing prosodic features—aspects like rhythm and emphasis—has proven crucial in fostering natural-sounding speech. The 30-minute window seems to create a sweet spot, allowing for the capture of a more fluid and natural speech pattern, which benefits the generative capabilities of voice cloning models.
Interestingly, the 30-minute format also aligns well with emerging trends in user personalization. We're seeing increasing customization options for synthetic voices, allowing adjustments to elements like pitch and tone. A more extended recording like this provides a better basis for these adjustments, leading to outputs finely-tuned to a specific audience or audio product, potentially maximizing listener retention.
There's also a potential benefit to representing dialects more accurately. This extended recording time allows for a better capture of a speaker's natural variations in speech, leading to more adaptable synthetic voices that can switch smoothly between accents or dialects. For content like audiobooks and podcasts, this increased diversity can expand the reach to a more diverse listener base.
Additionally, the 30-minute recording format seems to create a more comfortable listening experience. Studies suggest that listeners experience less cognitive fatigue when listening to synthetic voices that mirror natural speech patterns more accurately. This seems to be a direct consequence of the improved flow and natural cadence generated from the longer, more context-rich recordings.
The recent advances in emotion recognition algorithms also benefit from this standardized format. By using 30-minute recordings to train the models, content creators can more precisely instill desired emotional cues within the synthetic voice. For storytelling applications, the ability to convey a wide spectrum of emotions is essential for audience engagement.
The ability to readily conduct A/B testing with longer recordings provides a greater chance to find a specific voice that resonates with the desired audience. The extended format enables researchers to present distinct variations of synthesized audio, generating listener feedback that informs crucial refinements for optimized content production.
Finally, the rise of this 30-minute recording format also necessitates increased discussion around the ethical implications of voice cloning. With the capacity to capture a more robust representation of a person's speech and its associated emotional expression, crucial discussions around voice cloning recognition, ownership, and consent must continue. This heightened level of realism within synthesized voices introduces a need for clearer guidelines and protocols within audio content creation to ensure responsible use of these powerful tools.
In conclusion, the adoption of 30-minute recordings for professional voice cloning holds the potential to significantly improve the quality and application of synthetic voices. While this change offers considerable advantages, the growing sophistication of these tools also necessitates ongoing conversations about ethical implications to ensure responsible and respectful use of this rapidly developing technology.
The Evolution of Voice Cloning From Parody to Professional Production in 2024 - Video Game Industry Pioneers AI Voice Actor Partnerships
The video game industry is currently exploring a new frontier by partnering with AI voice actors, which signals a major shift in how voice talent is used in game development. Agreements have been established that enable professional voice actors to license their voices for use with AI platforms. These partnerships are attempting to build a new collaborative model that maintains the creative integrity of the performers while utilizing innovative AI technology. Though these developments present new avenues for game creation, they have prompted worries from voice actors regarding job security and the potential for AI-generated voices to diminish the artistic value of their work. The industry's experimental strategy may offer valuable lessons but also carries the risk of muddying the distinction between human and AI-created voices. As this technology advances, it's essential to contemplate its impact on storytelling in gaming and the future of audio production in a wider context.
The video game industry's embrace of AI voice actor partnerships is leading to noteworthy changes in production workflows and creative possibilities. Game developers are leveraging voice cloning to drastically reduce production timelines, particularly when creating multiple voice versions for various characters. This quick turnaround facilitates faster iteration cycles and allows for immediate player feedback loops throughout the development process.
Currently, AI voice cloning systems are proving capable of generating voices that convincingly reflect human emotional states. This capability is crucial for enhancing player immersion, as games increasingly rely on emotionally charged narratives to connect with audiences. However, even with these advances, the extent to which synthetic voices can replicate the subtleties of human emotional expression continues to be a point of discussion.
This partnership between game developers and AI voice cloning studios has also opened doors to greater localization. AI voice cloning can be easily adapted to different dialects and accents, enabling studios to create more culturally-relevant content and reach larger, more diverse player bases with minimal additional recording efforts.
The quality of AI-generated voiceovers has risen significantly with the integration of advanced neural vocoders. These systems process and replicate audio waveforms with remarkable accuracy, minimizing the artificial qualities that were often a drawback of earlier synthetic voice technologies. The outcome is a smoother, more natural-sounding audio experience for players.
Further refinements in voice synthesis algorithms are allowing for the nuanced representation of emotional cues. AI is becoming adept at capturing subtle shifts in tone and pitch, akin to the way humans express emotions. This capacity presents developers with new tools for storytelling, enabling them to craft characters that convey complex feelings in a more realistic fashion.
To improve the quality and authenticity of AI-generated dialogue, many voice cloning systems are incorporating language models to maintain contextually accurate responses within the game's narrative. This contributes to more believable interactions and strengthens the sense of immersion for players.
With ongoing improvements in AI voice technology, it's now possible for game characters to have unique and evolving vocal identities. Players might experience a noticeable shift in a character's voice as the narrative unfolds, adding another layer to the storytelling experience.
However, one persistent challenge is replicating the spontaneous and, at times, imperfect aspects of human speech. While AI can generate highly articulate voices, achieving the natural, off-the-cuff quality of human communication remains a point of contention for many developers. They recognize a need to strike a balance between efficiency and the expressive nuances of human interaction.
AI-based voice acting partnerships are expanding into the realm of interactive gaming, where a player's choices can directly impact a character's dialogue in real-time. The real-time synthesis of voices within these interactive environments is a considerable development, offering an enriched level of responsiveness for gamers.
Finally, there's a burgeoning trend of creating hybrid voice characters. AI voice cloning techniques allow for the blending of features from different actors, allowing the creation of unique synthetic voices and a new level of character design freedom. This method allows developers to maintain vocal diversity within games without requiring extensive recordings from a large pool of actors. While offering exciting possibilities for game creators, this approach further emphasizes the evolving nature of vocal performance in a world of increasingly advanced artificial voices.
The Evolution of Voice Cloning From Parody to Professional Production in 2024 - CoquiAI Claims Three-Second Voice Cloning in 2024
CoquiAI has sparked significant interest in 2024 by claiming the ability to clone voices in a mere three seconds. This represents a potential game-changer for audio production workflows, especially in areas like audiobook creation and podcasting. The idea is that creators can now generate realistic synthetic voices from incredibly short audio snippets, eliminating the need for extensive voice recordings. This focus on efficiency, however, prompts questions regarding the fidelity and emotional richness of these cloned voices, particularly in applications where subtle storytelling is critical. As CoquiAI continues to develop its technology, the trajectory from the playful world of voice cloning parodies to sophisticated professional production tools continues to reshape our understanding of voice and storytelling in the world of audio. It's a development that rightfully invites close scrutiny of the ethical considerations and potential consequences within the evolving audio production landscape.
CoquiAI has recently made a claim that could significantly impact the landscape of voice cloning: they're aiming for three-second voice cloning in 2024. This rapid turnaround time is a fascinating development, potentially making voice cloning a tool accessible to a wider range of creators. Their generative text-to-speech (TTS) model is designed to require only a short audio clip, significantly lowering the barrier to entry for utilizing this technology. Imagine being able to create a voice model in seconds rather than the 30-minute recordings that are now seen as standard for high-fidelity clones. This could revolutionize audio book creation, podcasts, or even interactive applications by making it quicker and easier to generate voices for various characters or scenarios.
However, it's important to acknowledge that the complexity of human speech remains a challenge, even with the impressive speed gains achieved by CoquiAI. The capability of capturing nuanced emotional cues, pauses, or subtle variations in tone within such a short sample is yet to be seen. We'll need to observe the outputs of these models to understand if they truly capture the intricate characteristics that make human voices so captivating. Furthermore, the ethical implications surrounding voice cloning are increasingly important as these technologies mature. The use of someone's voice without their explicit consent, and the potential for malicious misuse, raise concerns that need careful attention. It's essential to consider how this rapid pace of development interacts with existing laws and community standards around authorship, identity, and creative expression.
CoquiAI's approach leverages advanced AI techniques to clone voices across thirteen languages. This multilingual capability opens a path toward broader audio content accessibility. Their tools and models are accessible via their API and Coqui Studio, putting the power of voice cloning into the hands of a potentially larger community of developers. It is interesting that they are also committed to open science, which indicates a transparent approach and an intent toward collaborative development. This approach, if carried out effectively, may help address some of the anxieties around the ethical implications of the technology. While CoquiAI's goal of three-second voice cloning seems ambitious, it undeniably demonstrates the forward momentum in voice replication and the growing number of tools and techniques readily available for anyone who wants to dabble in the world of synthesized speech. The future of voice in various audio contexts seems to be rapidly evolving, and the role of these AI tools in shaping that future is certainly worth watching.
Get amazing AI audio voiceovers made for long-form content such as podcasts, presentations and social media. (Get started for free)
More Posts from clonemyvoice.io: