Get amazing AI audio voiceovers made for long-form content such as podcasts, presentations and social media. (Get started for free)
The Evolution of Voice Assistants How LLMs are Reshaping Audio Interaction Design in 2024
The Evolution of Voice Assistants How LLMs are Reshaping Audio Interaction Design in 2024 - Neural Voice Synthesis Creates A Shift From Rule Based Text To Speech Systems
Neural voice synthesis represents a major leap forward from older, rule-based text-to-speech (TTS) technologies. The shift hinges on the power of deep learning, enabling systems to generate remarkably natural-sounding speech. We're now seeing synthetic voices that capture subtleties like intonation, pitch variations, and even emotional nuances, previously impossible with rule-based methods. This has opened doors in diverse fields, impacting the creation of audiobooks, podcasts, and the exciting possibility of voice cloning. It allows for the preservation of unique voices, offering new ways to interact with and remember individuals. However, this progress presents new questions. The ethical implications of generating synthetic voices that sound human are considerable. As the technology progresses, we must examine how voice synthesis influences the notions of authenticity and ownership, especially in the realm of artistic expression and personal communications. Neural voice synthesis is not just about improving user experience, it's forcing us to engage with crucial discussions about the future of how we communicate through technology.
The field of voice synthesis has undergone a dramatic shift with the advent of neural networks. We've moved beyond the rigid, rule-based systems of the past, where text-to-speech (TTS) often resulted in robotic and monotonous outputs. Neural Text-to-Speech (NTTS) leverages the power of artificial neural networks to analyze and learn from vast amounts of human speech data. This approach allows the synthesis of speech that is far more natural and expressive.
The ability to replicate the subtleties of human speech – the nuances of intonation, pitch, and rhythm – is a game-changer. We can now create voices that convey a wide range of emotions, which was previously unattainable. This capability has a huge impact across different fields. For instance, imagine audiobooks that feel more like a human narration, or interactive gaming experiences where characters’ voices genuinely reflect their personalities.
Further, think of how podcast production can be streamlined. NTTS can create voiceovers rapidly, lowering production costs and potentially freeing creators to focus more on content. The potential for voice cloning is also compelling, but it brings its own set of issues. We're able to craft highly individualized voices, which could be profoundly helpful in creating assistive technologies for people with speech challenges. However, this advancement also raises important concerns about privacy and potential misuse, as it’s now possible to clone a person's voice with remarkable accuracy.
We're also seeing NTTS technology being integrated into real-time applications like customer service, which requires instant and clear communication. The ability to tailor synthesized voices to various accents and dialects opens up possibilities for global applications, as voices can be customized for different regions or audience preferences. This has implications for the future of voice acting, prompting a reconsideration of how humans and AI will collaborate in creating audio experiences. The demand for original and unique AI-generated voices may grow, alongside the need for human voice actors who can help shape and refine these new sonic worlds. It’s a fascinating and complex area of research, highlighting both the possibilities and challenges as we further integrate artificial intelligence into the realm of sound and audio design.
The Evolution of Voice Assistants How LLMs are Reshaping Audio Interaction Design in 2024 - Contextual Memory In Voice Design Enables Multi Turn Conversations
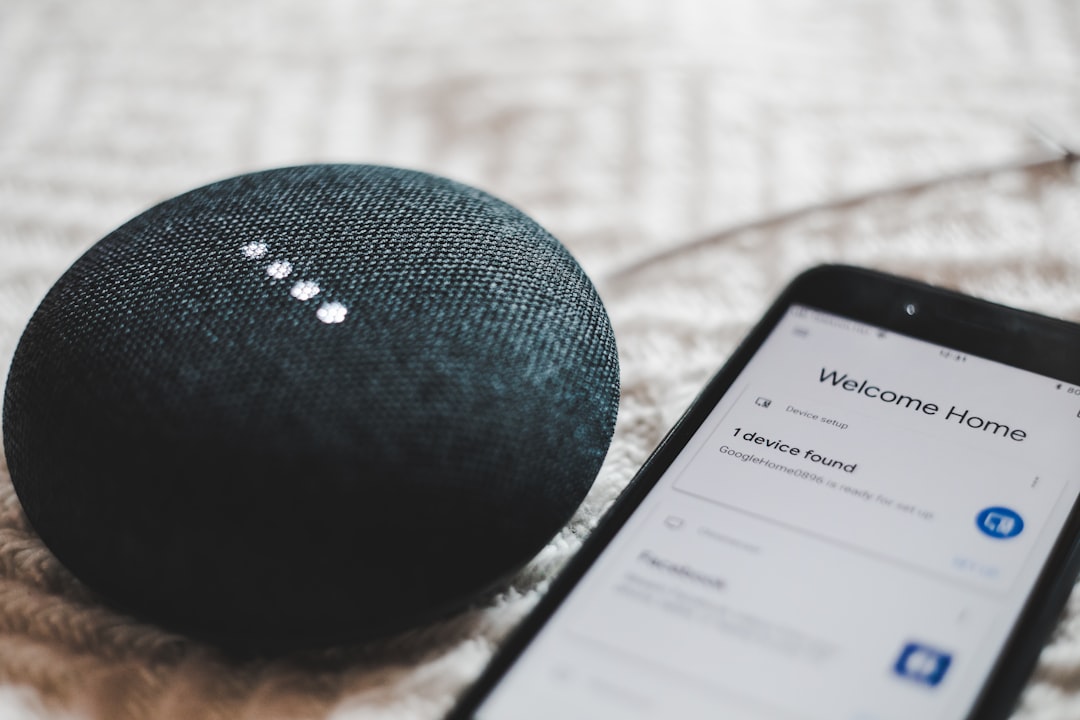
The ability of voice assistants to engage in multi-turn conversations is being fundamentally transformed by the integration of contextual memory. Previously, voice assistants often struggled to maintain a coherent understanding of the flow of a conversation, leading to disjointed and frustrating interactions. Now, with advancements fueled by large language models (LLMs), voice assistants can develop a more comprehensive grasp of the context of an ongoing dialogue. This enables them to provide responses that are more relevant and feel more human-like.
However, the path toward truly natural multi-turn conversations isn't without its challenges. Integrating real-time voice capabilities within LLMs still presents hurdles, particularly regarding latency. Reducing the delay between a user's input and the voice assistant's response is critical for maintaining a fluid and engaging conversational experience.
This evolution is impacting various areas of audio production. From enhancing the realism of audiobooks and podcasts to streamlining podcast creation, the ability to maintain conversational context improves the overall quality and user experience. Furthermore, the improved conversational ability has implications for other areas such as voice-powered customer service interactions, where fluid exchanges are essential.
The ultimate goal for voice assistants is to reach a level of conversational competence that rivals human interaction. While there's still much to be achieved in terms of creating truly nuanced and engaging conversations, this new focus on contextual understanding and responsiveness is shifting the way we interact with technology through sound, leading to more intuitive and engaging audio experiences.
Contextual memory is becoming increasingly important in voice design, particularly as we move towards more sophisticated multi-turn conversations. Think of it like a voice assistant's ability to remember the earlier parts of a conversation, allowing for more natural and coherent responses. This is a huge step forward from traditional voice assistants, which often struggled to understand the context of a conversation. They relied on interpreting each query in isolation, leading to a less fluid interaction.
Large language models (LLMs) are a game changer in this area, as they are capable of much deeper contextual understanding. However, there are some challenges here. Current voice interaction methods usually involve generating explicit text, which can be computationally expensive and slow down the response time, especially in multi-turn dialogues. Projects like IntrinsicVoice are attempting to address this by integrating real-time voice capabilities directly into LLMs, with the aim of overcoming those latency and computational issues.
The shift is clear – LLMs enable a more dynamic and human-like response generation. It's no longer about just producing a robotic-sounding response to a query. We’re now seeing systems that can adapt their responses based on the flow of the conversation. That being said, achieving truly natural, multi-turn conversations is still a work in progress, even for established voice assistants like Alexa.
Generative AI advancements are contributing to a more natural feel in voice interactions by improving the level of contextual awareness. We can see the progression from the initial voice assistants like Siri and Alexa, which set the stage for today’s more complex interactions.
The success of context-aware voice interactions depends heavily on how effectively the design incorporates contextual memory. It’s all about creating a better experience for the user. This naturally leads to further considerations on how we can optimize latency, and refine the design of interfaces for future developments. It’s a fascinating space where the goal is to improve the interaction between humans and technology through sound.
However, as the technology matures, it's important to acknowledge that there are some downsides. For instance, while voice cloning technology allows for incredible personalization, it also brings up important questions about ethics. The ability to replicate someone's voice with high fidelity raises concerns about potential misuse and the need for safeguards in terms of consent. It's a compelling space where progress in AI-powered voice interaction requires a careful balance between innovation and responsible development.
The Evolution of Voice Assistants How LLMs are Reshaping Audio Interaction Design in 2024 - Audio Generation Models Close The Gap Between Human And Synthetic Speech
The gap between human and synthetic speech is rapidly shrinking thanks to advancements in audio generation models. We're seeing a new era of audio production where synthetic voices are remarkably lifelike, capable of capturing the nuances of human speech, including emotional expression. Models like WaveNet and AudioLM are leading the charge, using deep learning techniques to analyze vast amounts of audio data and synthesize speech that sounds incredibly natural. This has opened up new possibilities in areas like audiobook production, where synthetic voices can now deliver engaging narratives, or in podcast creation, where they can be used to generate voiceovers efficiently. The increasing realism of synthetic speech is not only improving user experiences across various platforms but also raising profound questions. We're forced to consider the ethical implications of voice cloning technology and the broader impact on notions of authenticity and ownership in artistic expression and personal communication. As these audio generation models continue to improve, they challenge our understanding of what constitutes a "real" voice, potentially reshaping how we communicate and perceive identity in our digital world.
The field of audio generation has seen remarkable progress, particularly in the realm of speech synthesis. Models like AudioLM, leveraging sophisticated frameworks, are now able to produce incredibly realistic speech and even music by meticulously analyzing vast amounts of audio data. This has resulted in a substantial narrowing of the gap between human and synthetic speech, demonstrating impressive long-term consistency and exceptional fidelity.
Transformer-based architectures have proven pivotal in the development of these powerful audio models. They excel across a range of audio tasks, including automatic speech recognition and, importantly, text-to-speech (TTS) generation. This has led to exciting explorations like ConversaSynth, a proposed framework designed to generate synthetic conversations with multiple speakers, each possessing distinct personas. This is achieved through a clever combination of large language models (LLMs) and TTS systems.
Furthering the interaction between humans and LLMs, projects like FunAudioLLM are incorporating models for multilingual speech recognition, emotion identification, and natural speech generation. This adds another layer of sophistication to how we can interact with AI, making it more engaging and accessible. The quest for truly human-like voice quality has been greatly aided by models like WaveNet. This deep generative model focuses on raw audio and has achieved impressive results, substantially minimizing the discrepancy between synthetic and human voice quality, especially in TTS applications.
These advancements are significantly reshaping voice assistant technology. By incorporating these sophisticated audio generation techniques, voice assistants are becoming more natural and engaging. Deep learning models are demonstrating the capacity to create remarkably human-like voice outputs, opening up new avenues in areas like gaming and music production. We're observing a shift in the nature of voice interactions, with a growing emphasis on nuanced and contextually aware audio responses driven by the capabilities of LLMs.
The fusion of audio generation models and interaction design is reshaping user experiences across various platforms. This shift underscores the need for audio interfaces that are not only lifelike but also highly responsive. However, this increasing realism brings into sharp focus ethical questions. The ability to clone voices with great accuracy, while offering exciting possibilities for things like audiobook production and assistive technologies, raises serious concerns about potential misuse and the need to ensure users' rights and control over their own voices. The evolution of audio interaction design is leading to some fascinating and complex challenges as we move into the future of sound.
The Evolution of Voice Assistants How LLMs are Reshaping Audio Interaction Design in 2024 - Multimodal LLMs Transform Voice Assistants Into Universal Audio Interfaces
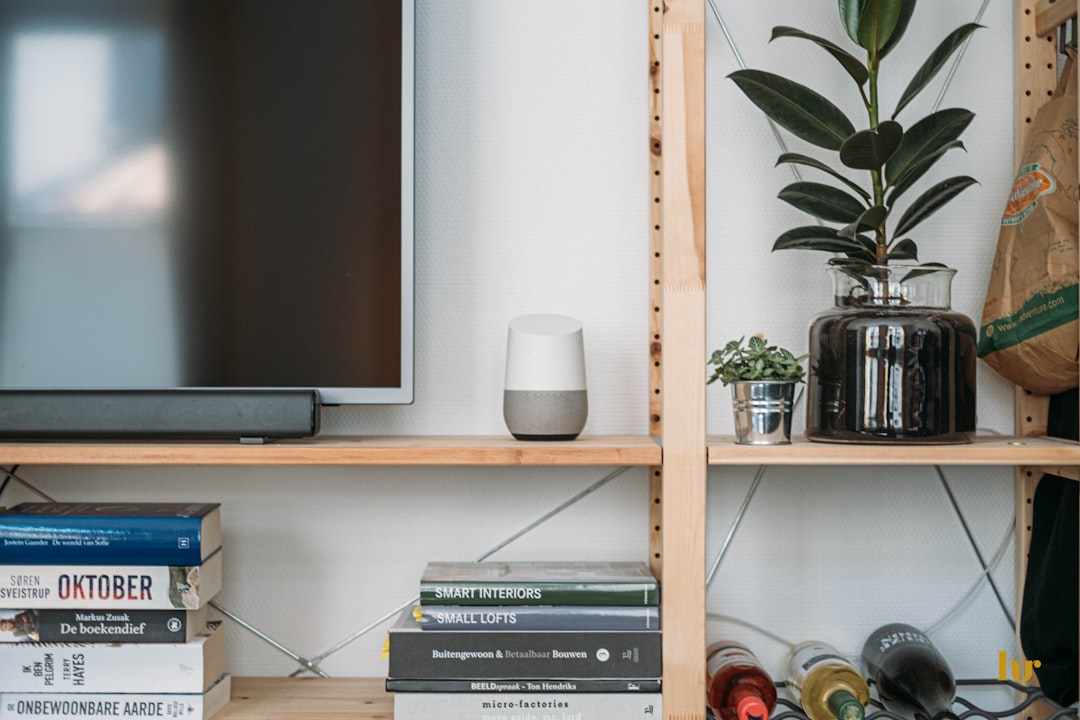
The emergence of Multimodal Large Language Models (MLLMs) has fundamentally altered the landscape of voice assistants, effectively transforming them into versatile audio interfaces. No longer limited to text-based instructions, these systems now possess the ability to process and react to a wide array of audio inputs. This newfound capability significantly improves the understanding of user intent and context, leading to voice assistant interactions that feel more natural and responsive.
This advancement benefits diverse audio-related fields. Audiobook production, for example, can leverage these enhanced capabilities to generate more lifelike narrations. Similarly, podcast creation can be revolutionized with more dynamic voiceovers. The increasing indistinguishability between synthetic and human voices, however, highlights ethical concerns. Questions surrounding voice cloning and its potential for misuse are now central to discussions about the future of voice assistants.
This evolution signifies a significant shift in the way we interact with technology, moving towards a world where audio interactions are paramount. While the potential for creating truly immersive audio experiences is undeniably exciting, it underscores the crucial need to develop these technologies responsibly. The future of voice interactions is intrinsically linked to our ability to navigate the ethical dilemmas that accompany the creation and application of increasingly sophisticated synthetic voices.
Multimodal Large Language Models (MLLMs) are fundamentally altering how we interact with voice assistants, essentially transforming them into versatile audio interfaces. These models go beyond traditional text-based interactions by processing and responding to audio data, creating a much richer conversational experience. The integration of advanced LLMs allows voice assistants to better grasp user intent and the overall context of a conversation, leading to more natural and meaningful interactions. This is a significant improvement over older voice assistants that relied on simpler language models, which often struggled with complex or nuanced requests.
Recent advancements in MLLMs have been especially noteworthy. Researchers have developed innovative training strategies that enable these models to handle multiple types of input and output more effectively. Models like MacawLLM illustrate this trend by easily aligning multimodal data with LLM embeddings, making it simple to incorporate diverse data types into the assistant's capabilities. The "one-stage instruction fine-tuning" approach employed by some MLLMs streamlines the process of adapting these models, leading to quicker deployment of new features in voice assistants.
The broader implications of these advancements are significant. We're witnessing a shift towards more intuitive audio interfaces, where voice becomes a primary means of interacting with technology. This could lead to a wide range of applications beyond the traditional keyboard and screen. For example, LLMs could empower voice assistants to produce a vast range of emotional vocalizations within audiobooks, creating a much more dynamic listening experience. Further, the potential for LLMs to generate customized sound effects that react to user input opens doors in interactive gaming and immersive storytelling applications.
Podcast production is another area where MLLMs could have a major impact. Podcasters could leverage audio generation capabilities within LLMs to create entire voiceovers, potentially revolutionizing production pipelines. The same contextualized voice cloning capabilities useful for dynamic podcast segments could be applied to creating individualized audiobooks, tailoring the delivery style to match the tone of the book. The ability to effortlessly switch between languages and dialects while maintaining conversation flow would open up audio interaction for global audiences, democratizing access to information and entertainment.
The ability of LLMs to integrate and adapt based on user interactions further refines the audio experience. Over time, the voice assistant learns user preferences, resulting in a unique auditory profile and more personalized interaction. This is a fascinating area of research, especially with the simultaneous rise of high-fidelity voice cloning technology. The potential for highly accurate voice replication creates a necessity for strong ethical guidelines and authentication methods to protect user identity. Simultaneously, the progress in voice cloning could revolutionize assistive technologies for people with speech impairments, opening up possibilities for personalized communication and greater accessibility.
This fusion of advanced audio models and LLMs creates a fascinating space in human-computer interaction. While the technical improvements are impressive, the evolution of voice assistants and their integration into our everyday lives necessitates careful consideration of the ethical implications as the technology matures. The potential benefits are enormous, but ensuring responsible development and the protection of user autonomy is crucial to ensure this evolving technology is used for good.
The Evolution of Voice Assistants How LLMs are Reshaping Audio Interaction Design in 2024 - Real Time Voice Interaction Processing Reduces Latency Below 100ms
The realm of real-time voice interaction is experiencing a significant shift towards lower latency, with efforts focused on achieving response times under 100 milliseconds. This pursuit of near-instantaneous responses is crucial for fostering a seamless and intuitive conversational flow, particularly vital for experiences like audiobooks and podcasting where continuous and fluid interaction is paramount. Innovative approaches, including systems like IntrinsicVoice and StreamVC, are attempting to address the challenge of integrating large language models (LLMs) with real-time voice processing, allowing for a smoother transition from textual understanding to spoken responses. The benefits of this reduced latency are apparent in a more responsive and engaging user experience, yet the implications raise questions about the nature of synthetic voices. As we progress through 2024, this technological evolution has the potential to reshape how we interact with audio, offering broader access and more adaptable voice-driven interactions across a wider range of applications. While these advancements offer a richer and more nuanced experience, we must consider the broader impact on the perception of authenticity and ownership of synthesized voices.
The pursuit of truly natural and engaging voice interactions hinges on minimizing delays, or latency. Researchers and engineers are striving to achieve a latency of under 100 milliseconds, a threshold where users perceive the interaction as instantaneous. Anything beyond this can create a jarring disconnect, making conversations feel robotic or frustrating, particularly in scenarios like customer service interactions or during real-time voice calls.
These efforts rely on cutting-edge neural signal processing techniques. These techniques are designed to analyze audio inputs in real-time, capturing not just the spoken words, but also the subtle cues of human speech, such as tone and emotion. This allows voice assistants and other AI-driven systems to understand the context of the interaction more deeply, leading to a more engaging experience.
However, achieving incredibly low latency often requires compromises. Maintaining a high level of natural-sounding speech, while simultaneously aiming for super-fast responses, poses a significant technical challenge. There's an inherent trade-off between voice quality and speed. Innovative compression algorithms are being explored to potentially bridge this gap, allowing for quick responses without sacrificing the richness of synthetic voices.
Moreover, real-time processing introduces opportunities for immediate error correction. Voice assistants can learn from their mistakes as they happen, which is essential for applications like audiobook narration, where a smooth and seamless flow is vital for listener engagement. This also applies to podcast creation, where maintaining momentum and preventing jarring disruptions is key.
The combination of real-time voice processing and advanced voice cloning techniques has unlocked new possibilities for dynamic voice profiles. For example, a voice assistant could effortlessly shift between a casual and formal tone depending on the context of the interaction. It's like having a voice actor that adapts their delivery instantly, providing a personalized and engaging experience for each user.
Furthermore, real-time voice interaction enables a continuous feedback loop. Voice assistants can monitor user reactions and adjust their responses accordingly. This offers interesting possibilities in areas like podcast production. A podcast might alter the delivery or content based on audience engagement indicators in real-time, creating a more dynamic and responsive listening experience.
Beyond improving the user experience, minimizing latency also reduces cognitive load. If there is a significant delay in a conversation, users can struggle to keep track of the flow, making it mentally taxing, especially for long-form content like audiobooks or extended podcast discussions.
The ability to process audio in real-time also expands the emotional spectrum of synthetic voices. This allows voice assistants to convey a much wider range of feelings, enhancing the sense of empathy and human connection. This could be especially important for applications like interactive storytelling or voice-powered customer service, where emotional responsiveness is crucial for building trust and rapport.
These developments are driving a reassessment of how we measure and define audio quality. Traditional metrics may not be adequate for scenarios where speed is paramount. Researchers are developing new methods to assess the quality of real-time audio processing, ensuring that synthesized voices remain clear and expressive even under extreme time constraints.
Looking forward, these advancements in real-time voice processing pave the way for a future where we seamlessly interact with technology using a blend of voice, gestures, and visual inputs. This fusion of modalities promises richer and more intuitive interactions across a variety of fields, including gaming, interactive storytelling, and enhanced user interfaces. While this is an exciting area, we need to constantly evaluate the ethical implications as we see more and more possibilities unfold in this space.
The Evolution of Voice Assistants How LLMs are Reshaping Audio Interaction Design in 2024 - Voice Assistant Architecture Moves From Cloud To Local Edge Devices
Voice assistant technology is moving away from relying solely on distant cloud servers and increasingly leveraging the processing power of local devices, often referred to as edge devices. This shift allows for quicker audio processing, making interactions feel more instantaneous and responsive – a crucial element for applications like audiobook narration or creating dynamic podcasts. Additionally, edge computing empowers devices with the ability to enhance voice clarity using methods such as beamforming, which isolates a user's voice amidst surrounding sounds, leading to more understandable communication. This trend of bringing voice assistant processing closer to the user opens up intriguing possibilities for future development, potentially leading to a more seamless audio experience while maintaining acceptable quality levels. However, this development also brings to the forefront the importance of considering privacy and the ethical implications of advanced technologies like voice cloning, particularly in the realm of sound production and creative applications. The capacity for local processing also makes cutting-edge voice technologies more accessible, potentially inspiring new avenues for artistic expression across diverse audio domains like podcasting and voice-related applications.
The evolution of voice assistants is taking a fascinating turn with the shift from cloud-based processing to local edge devices. This change is not just a matter of technological advancement, it fundamentally alters the way we interact with and perceive these digital companions.
One of the most immediate impacts is heightened user privacy. With voice commands processed locally, sensitive data stays on the device, reducing the risk of it being intercepted or misused during transit. This shift also brings noticeable improvements to performance, with faster processing speeds and a more fluid, responsive experience. The ability to achieve sub-100-millisecond latency is particularly exciting, especially for applications that require a continuous and dynamic interaction, like audiobook narration or interactive podcast features.
The decentralized nature of edge-based voice assistants opens doors for innovations like on-device voice cloning. Imagine having the ability to create a truly personalized voice assistant, tailoring it to your own preferences and communication style. This also expands opportunities for creators working in audio; think of easily producing customized voiceovers for a podcast, or having greater control over the sounds and tones used to create compelling audiobooks.
Furthermore, these local processing capabilities allow voice assistants to dynamically adapt to the user's environment. They can automatically adjust volume, tone, and even clarity in response to ambient noise, making them a much more useful tool for listening to podcasts in a busy cafe or narrating an audiobook while commuting on a train.
A crucial advantage of this shift is the reduced reliance on consistent internet connectivity. This is hugely beneficial in areas with patchy or unreliable service, as users can still access voice functionalities to produce podcasts, edit recordings, or even listen to audiobooks without any interruption.
The ability to store and utilize contextual memory locally improves conversation flow. Voice assistants can remember your preferences, past interactions, and maintain a coherent thread of dialogue, ultimately leading to a more natural and engaging experience when consuming audiobooks or interactive podcasts.
The shift also enables greater flexibility in audio generation. Edge-based assistants can generate unique audio effects and voice modulations in real-time, empowering audio creators and podcasters with new tools for enhancing their work. This ability to refine and tailor audio production on the spot opens a realm of possibilities.
One of the interesting side effects of this transition is that developers can create voice assistant experiences that are more consistent across devices and platforms. This consistency ensures that users encounter a consistent "voice" or sonic identity, no matter where they are interacting with the assistant, thus strengthening brand trust and user satisfaction in the quality of synthetic voice output for podcasts, audiobooks, or any other audio-related experiences.
Furthermore, the ability of these localized assistants to analyze and respond to a user's unique speech patterns, their natural voice inflections, and even emotional nuances, makes the experience more human-like and relatable. The voice assistant adapts its communication style to match that of the user.
Finally, and perhaps most importantly, this transition empowers real-time feedback loops in interactive audio applications. Imagine a podcast where the storyline shifts or adapts in response to the audience's engagement level—that's the kind of immersive experience now within reach thanks to local processing. The direct interaction with a user through voice offers incredible possibilities for crafting engaging and personalized audio experiences.
This change from cloud to edge is a major shift, one that will continue to shape the future of voice assistants. While the potential benefits are tremendous, it's crucial to remain mindful of the potential ethical implications. This area requires ongoing attention to ensure these evolving technologies are used responsibly and in a way that benefits all users.
Get amazing AI audio voiceovers made for long-form content such as podcasts, presentations and social media. (Get started for free)
More Posts from clonemyvoice.io: