Get amazing AI audio voiceovers made for long-form content such as podcasts, presentations and social media. (Get started for free)
Precision Pacing Optimizing Word Count for 10-Minute Voice Cloning Projects
Precision Pacing Optimizing Word Count for 10-Minute Voice Cloning Projects - Optimizing Audio Input Quality for Voice Cloning Accuracy
The quality of the audio input directly impacts the accuracy of voice cloning. Essentially, the better the audio, the more precise the resulting synthetic voice will be. This means recordings need to be of high fidelity, capturing the nuances of the target speaker's voice. Improvements in audio processing are continuously refining the quality of the audio input, effectively minimizing the impact of noise and other artifacts that can hinder the cloning process.
Furthermore, choosing the right training datasets is vital. This includes leveraging both high-quality and low-quality audio sources to fine-tune the cloning model and maximize its ability to replicate the speaker's voice effectively. The journey of voice cloning is continually evolving, with ongoing development of new algorithms pushing the boundaries of how accurately we can synthesize speech. This advancement highlights the ongoing struggle to balance voice authenticity with the constraints inherent in using a limited amount of reference data for a speaker.
Achieving accurate voice clones hinges on the quality of the input audio. The microphone's frequency response, ideally spanning 20Hz to 20kHz, plays a major role in capturing the complete range of human vocal nuances. If certain frequencies are amplified or attenuated, it can skew the characteristics of the voice during the cloning process.
Background noise, even seemingly subtle sounds, can contaminate the recording. Research shows that these artifacts can degrade the fidelity of voice models, leading to less accurate clones. Microphones with a cardioid polar pattern can effectively filter out unwanted sounds from the sides and back, isolating the speaker's voice and enhancing audio clarity for cloning.
The bit depth and sample rate of the audio are paramount for capturing subtle vocal details without loss or distortion. Aiming for at least 24-bit and 48kHz ensures that the richness and complexity of the voice are preserved. However, we've found that the closer the microphone is to the mouth, the more pronounced the bass frequencies become (known as the proximity effect). This alteration in frequency response can inadvertently impact how the voice is ultimately cloned.
Unwisely implemented audio compression can introduce artifacts, effectively masking the natural tonal characteristics of the voice. Lossless formats are the preferred choice for preserving the integrity of the source audio during cloning. Techniques such as de-essing are crucial for preparing audio recordings, as excessive sibilance and plosive sounds can interfere with clarity. These imperfections will impact the accuracy of the voice models trained from this audio.
Room acoustics also influence the integrity of the recording. Uncontrolled environments can cause unwanted reflections and interference patterns that obscure the voice's clarity and intelligibility, making cloning more complex. While Automatic Gain Control can be useful for maintaining a consistent level, we've observed that it can introduce fluctuations which can disrupt the audio's uniformity. Manual gain settings are often a better solution for a cleaner, more consistent signal throughout the recording, contributing to higher-quality clones.
The selection of the audio interface is equally significant. A high-quality audio interface boasts better digital converters, minimizing latency and preserving the full dynamic range of the audio signal. This translates into a more accurate representation of the speaker's voice during the cloning phase. This allows for more precise modeling and a closer representation of the original voice.
Precision Pacing Optimizing Word Count for 10-Minute Voice Cloning Projects - Balancing Word Count and Recording Duration for Efficient Cloning
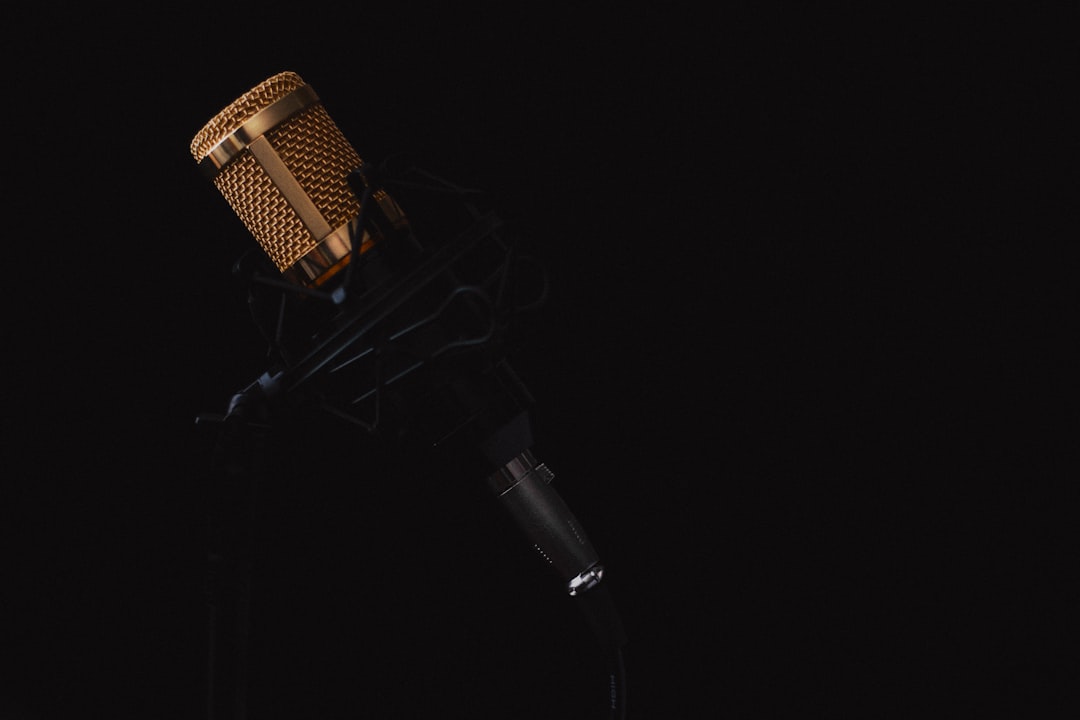
In the realm of voice cloning, finding the sweet spot between the number of words spoken and the overall recording length is crucial for efficiency and quality. The quantity of audio directly influences the time and effort needed for the cloning process, demanding careful consideration of the ideal recording duration for your specific project. While brief audio snippets might be adequate for fast cloning, longer recordings offer a richer source of data that allows the model to better understand the unique qualities of a voice. This delicate balance helps optimize the precision of the cloned voice and streamlines the entire workflow. This careful approach ensures the final output matches the desired level of accuracy and authenticity without adding unnecessary complexity. By understanding these interconnected factors, one can significantly improve the outcomes of voice cloning endeavors, particularly in fields such as audiobook and podcast production where subtlety and nuance in vocal delivery are paramount to the listener's enjoyment.
When aiming for efficient voice cloning, especially within a 10-minute timeframe, the interplay between word count and recording duration becomes crucial. While a 10-minute recording might typically yield around 1,500 to 2,000 words, the actual word count can deviate substantially due to factors like speaking pace and natural pauses. This variation makes it challenging to predict the optimal recording length for a particular speaker.
Individual speech patterns introduce further complexity. Some individuals speak rapidly, potentially compressing words and altering the usual word-to-time ratio. Conversely, others speak more slowly, incorporating longer pauses, requiring a different approach to achieve effective cloning. Similarly, variations in pronunciation, influenced by regional accents and dialects, can create a wider spectrum of word and phrase realizations, significantly impacting both the recording pace and the resulting cloned voice's quality.
The way a speaker manages their breath also plays a role. Controlled breathing can contribute to better recording quality and improved timing. By paying attention to breath placement, the speaker can maintain a more consistent rhythm and clarity, reducing the need for extensive post-production edits. Interestingly, the emotional tone conveyed through speech often influences the speaking rate. Research indicates that people tend to alter their pace based on emotional content, which introduces further intricacies for voice cloning models as they must accurately reflect this natural emotional variability in synthetic speech.
The recording environment itself can impact the perceived duration of the audio. Rooms with poor acoustics might increase reverberation, leading speakers to adjust their pace to maintain clarity. This can result in longer recordings, indirectly complicating the voice cloning process.
Compression techniques, while useful for storage efficiency, can introduce artifacts that distort certain frequencies. These distortions can subtly alter the perceived naturalness of the voice, requiring a careful consideration of the balance between word count and recording quality. It's also important to remember that voice cloning models require significant training data. Researchers are finding that augmenting datasets with specific, carefully chosen supplementary recordings can improve the model's ability to adapt to different speaking styles, enhancing cloning fidelity.
Furthermore, the sensitivity of advanced voice cloning algorithms to different input features like word complexity and length varies considerably. This variability underscores the need for thorough experimentation to find the sweet spot where adjustments to these factors contribute to optimal synthetic voice quality.
Real-time feedback during recording can be immensely valuable. Utilizing tools that allow speakers to monitor their pacing and clarity as they record can help them identify and adjust potential issues on the fly, resulting in more coherent and effective voice clones. Ultimately, navigating the relationship between word count, recording duration, and a range of other factors impacting voice quality is an ongoing challenge. As voice cloning technologies continue to develop, our understanding of these nuances will become even more critical.
Precision Pacing Optimizing Word Count for 10-Minute Voice Cloning Projects - Leveraging Advanced Microphone Techniques for Clearer Voice Samples
Capturing high-quality voice samples is crucial for various applications, including audiobook production, podcast creation, and voice cloning projects. The effectiveness of microphone techniques plays a vital role in achieving clear and detailed recordings. Choosing the right microphone is critical, as different types are better suited for various recording environments and speaking styles. Strategic microphone placement and the use of cardioid polar patterns are beneficial for minimizing background noise and isolating the speaker's voice. The clearer the initial audio sample, the better the cloning or production outcome. Furthermore, developing techniques like conscious breathing and incorporating vocal exercises can refine the voice's clarity and help capture the subtleties in a speaker's tone. Paying close attention to these nuanced techniques can enhance the quality of the recordings, leading to more successful outcomes in the respective projects, producing more natural and authentic-sounding audio that's enjoyable for listeners.
Capturing high-quality voice samples is fundamental to accurate voice cloning, and microphone techniques play a crucial role in achieving that goal. Different microphone polar patterns, like the cardioid pattern, can effectively isolate a speaker's voice while reducing unwanted background noise, contributing to cleaner recordings. However, keeping the microphone close to the mouth – a common practice to capture more intimate sounds – triggers the proximity effect, boosting bass frequencies that might alter a voice's natural tone in the cloning process. It's important to consider this phenomenon to avoid unforeseen changes in the cloned voice.
The recording environment itself introduces another factor to consider. Uncontrolled acoustics, like in rooms with many hard surfaces, cause unwanted reflections, creating muddiness and making the cloning process harder. To counter this, incorporating sound-absorbing materials can drastically improve the clarity of a recording. The quality of audio capture, measured in bit depth and sample rate, also influences the faithfulness of cloning. A high bit depth of 24-bit and a 48kHz sample rate can preserve the most intricate details of a voice, leading to more lifelike voice clones. Similarly, choosing an audio interface with a wider dynamic range allows for recording a broader range of vocal expressions, from subtle whispers to powerful shouts.
Achieving a consistent speaking pace throughout recordings is essential for a successful voice cloning project. Controlled breathing techniques are crucial in maintaining a steady rhythm, which directly affects how the cloned voice sounds. Additionally, using noise gates during recording can help remove low-level background sounds, thus reducing extraneous noise and leading to a clearer voice sample. Interestingly, while audio compression can be helpful for storing voice data efficiently, using lossy compression methods carries the risk of losing important vocal details, which might affect the resulting cloned voice. Consequently, it's advisable to utilize lossless formats for preserving the quality of the original voice.
The distance between the microphone and the speaker can also have a significant impact. If the microphone is positioned too closely, the proximity effect becomes even more pronounced. On the other hand, placing it too far might reduce the capture of finer vocal nuances. Finding the optimal balance is necessary to obtain the desired audio fidelity. Moreover, capturing a speaker's voice is complicated by the inherent variability in how they speak – their pace, accent, and the emotional tone they convey. These elements create complexities for voice cloning algorithms that must effectively adapt to this variability to reproduce the speaker's voice accurately. Thus, a diverse training dataset is essential to capture a broader spectrum of speaking characteristics, making the clones more accurate and representative of the individual's vocal style. The challenges faced with capturing high-quality voice samples and the impact of different microphone and recording techniques emphasize the ongoing research and development surrounding voice cloning technologies.
Precision Pacing Optimizing Word Count for 10-Minute Voice Cloning Projects - Adapting Script Content to Maximize Phonetic Diversity in 10 Minutes
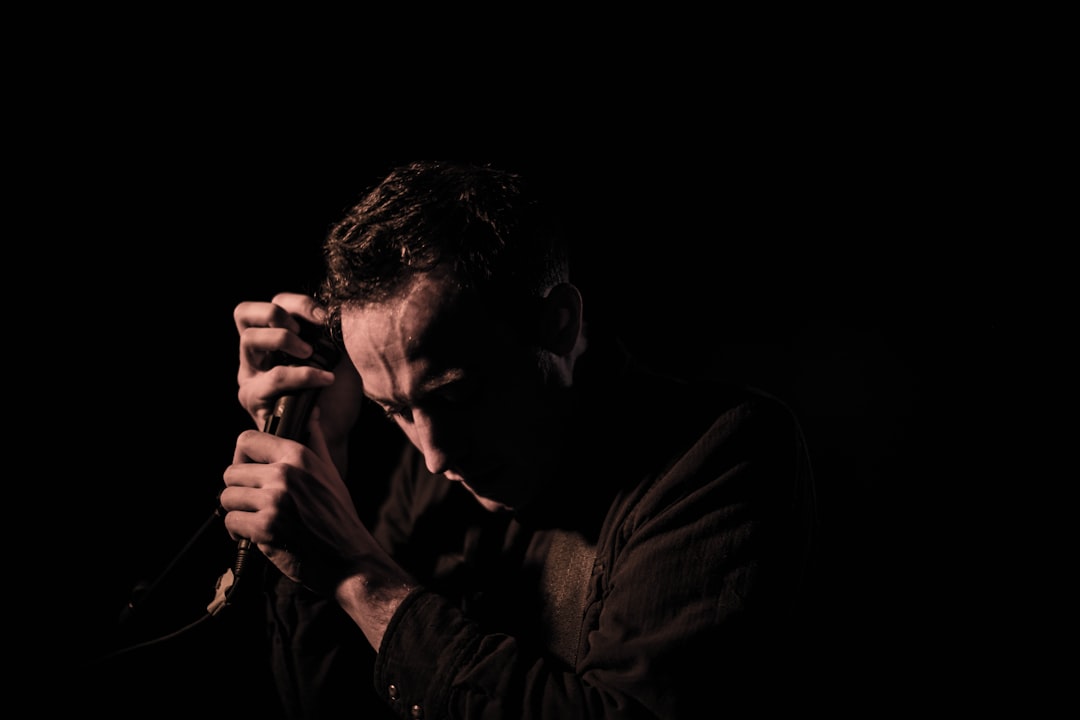
When aiming to create a high-quality voice clone within a tight 10-minute timeframe, crafting script content that maximizes phonetic variety becomes crucial. By including a wide range of sounds and pronunciations, we can help the voice cloning model develop a more nuanced understanding of the target speaker's vocal patterns. This results in a synthetic voice that sounds more human and natural, as opposed to robotic or monotone.
Methods like using AI-driven language generation tools can assist in expanding the phonetic landscape within the script. Carefully structuring the script to include diverse word choices and sentence structures helps ensure a broad range of sounds. Paying attention to the intended emotional tone of the script and how that might impact speech rhythm and inflection is also important. Understanding how individuals naturally vary in their speech patterns, whether it be pace, accent, or dialect, can further enhance the script adaptation process. This awareness helps in crafting a script that mirrors the target speaker's characteristics as authentically as possible.
The objective is to move beyond simply generating spoken words, and instead create an audio experience that feels authentic and engaging for the listener. By thoughtfully adjusting the script and employing intelligent tools, we can unlock the full potential of voice cloning for applications like audiobook narration and podcast production.
Within the realm of voice cloning, particularly when aiming for 10-minute projects, we've found that the diversity of sounds within the audio script has a substantial impact on the quality of the cloned voice. Essentially, a wider range of phonetic elements helps the cloning model learn a more complete representation of a speaker's vocal characteristics. This suggests that focusing on the variety of sounds, not just the words themselves, is important.
For instance, the rhythm and timing of speech influence how natural the cloned voice sounds. Research indicates that scripts with a deliberate mix of short and long words and phrases help the cloning model understand how humans naturally vary their pace, leading to a more lifelike outcome. The type of vowel sounds used can significantly impact clarity. Cloning systems sometimes struggle with vowels that sound similar, especially when dealing with audio that's relatively short. We've observed that intentionally including diverse vowel sounds can resolve some of these issues.
Beyond vowel sounds, the specific structure of sounds – like how consonants and vowels are combined – offers further avenues for improvement. Complex sounds like diphthongs and triphthongs can provide a lot of information for the model. Essentially, these more intricate combinations force the model to work harder, resulting in more nuanced vocal recreations. Currently, a lot of attention is being paid to the syllable structure of words. Since syllables represent basic units of speech, a better understanding of the syllable structure can help generate more dynamic cloned voices.
Consonant sounds – which range from relatively simple sounds to complex combinations – provide another challenge for voice cloning models. Scripts that feature a wide variety of these types of sounds appear to lead to better results, as the models learn how to replicate a broader range of articulatory motions.
Interestingly, even casual speech patterns like using contractions or dropping sounds (for example, saying "gonna" instead of "going to") can be beneficial for voice cloning. By incorporating these common features into the scripts used for training, the model gets exposed to how people naturally simplify their speech, improving its ability to produce more conversational synthetic voices.
Another factor we've been exploring is the relationship between emotion and sound. It appears that using scripts with different emotional content helps the model understand how those emotions influence the specific sounds a person makes. This is particularly important for applications where emotional delivery is crucial, such as storytelling or audiobook narration.
Our ongoing research suggests that using a variety of phonetic elements across multiple training sessions can have a cumulative effect on voice quality. This approach can potentially avoid over-reliance on specific sounds which can limit the overall performance of the model. Additionally, the overall "load" or balance of different types of sounds seems to affect how well the voice is captured. We've seen that ensuring both common and less frequent sounds are represented in the audio helps to more accurately capture the unique tone of a speaker's voice.
It is clear that carefully considering the specific sounds included in training data for voice cloning is an important factor to consider. This is particularly true when working within time constraints like those imposed by a 10-minute training session. However, this is an area where ongoing research is helping us to understand these complex relationships between how we speak and how effectively we can clone a person's voice.
Precision Pacing Optimizing Word Count for 10-Minute Voice Cloning Projects - Implementing Real-Time Adjustments During Short Recording Sessions
When working with short recording sessions for voice cloning, especially within a 10-minute timeframe, the ability to make real-time adjustments is crucial for achieving high-quality results. These brief sessions require careful management of elements like pacing, tone, and emotional expression to ensure the cloned voice aligns with the desired outcome. The capacity to monitor these aspects as the recording progresses allows for immediate corrections. This could involve fine-tuning the speed of delivery, strategically inserting pauses for emphasis, or even adjusting the overall tone to ensure it matches the original speaker's nuances.
By incorporating these on-the-fly corrections, one can mitigate the risks associated with short recording sessions and ensure the cloned voice maintains a natural flow and avoids sounding robotic or monotonous. These immediate adjustments help to capture the subtle complexities of human speech, which is essential for replicating a speaker's voice accurately. While it's still a challenge to perfectly replicate the diverse spectrum of human speech in a short timeframe, real-time adjustments significantly improve the ability to achieve a more realistic and engaging cloned voice. Furthermore, incorporating real-time feedback mechanisms within voice cloning workflows will likely become even more critical as the technology advances and pushes towards ever-increasing levels of authenticity.
During short recording sessions, especially those focused on 10-minute voice cloning projects, managing the proximity of the microphone to the speaker is vital. If the microphone is too close, the resulting audio can have an unnatural boost in low frequencies, potentially distorting the cloned voice's tonal characteristics. This highlights the importance of understanding this "proximity effect" and adjusting microphone placement for optimal results.
The ability to monitor the audio during recording is invaluable. Using tools that provide real-time visual feedback, like volume or frequency visualizations, enables the speaker to make instantaneous adjustments to the recording, ensuring it remains within the ideal parameters for cloning. This can lead to a cleaner, more coherent audio input that ultimately translates into a higher-quality synthetic voice.
Maintaining consistent pacing during the recordings is another challenge. Controlled breathing can help in this area. If breath control isn't managed effectively, the resulting audio might exhibit inconsistencies in the pace of speech, potentially impacting the natural flow of the cloned voice.
When training a voice cloning model, the detailed structure of syllables proves significant. A deeper understanding of how these structures are used in spoken language can help the model learn more intricate patterns of human speech, resulting in a more natural-sounding clone.
Voice cloning models sometimes struggle with differentiating between vowels that sound very similar. Including a diverse range of vowels within the training script can help mitigate this issue. Ensuring a wider range of vowel sounds helps the model better distinguish between various vocalizations, ultimately enhancing clarity in the synthesized speech.
Optimizing the overall quality of the cloned voice necessitates designing scripts with maximal phonetic variation. The inclusion of a wider spectrum of sounds and pronunciation within the training data helps the model learn a more complete representation of the speaker's voice. A well-crafted script that caters to this objective contributes to the overall accuracy and naturalness of the synthetic voice.
The emotional tone a speaker conveys heavily influences aspects like pace and pronunciation. This natural interplay between emotion and speech can be challenging for voice cloning models to capture. When the models are trained using audio that includes a range of emotional content, it leads to a more effective simulation of natural emotional inflection, crucial for applications like storytelling.
The acoustic conditions of the recording environment are another important factor. Unwanted reflections caused by a poorly treated space can interfere with the clarity of the voice. Introducing sound absorption to the recording area can lessen the impact of these reflections, leading to a clearer and more suitable audio input for cloning.
Expanding the diversity of the training dataset is a continuous effort. Research has shown that using techniques to augment the training data, adding diverse vocal samples, can lead to models with improved accuracy and a greater capacity to generalize across various voices. This emphasizes the significance of continuous improvement in the training data.
Utilizing audio compression is common, but it's crucial to employ it judiciously. While compression can make storage of audio more efficient, poorly implemented lossy compression can discard valuable vocal nuances. Using lossless compression techniques ensures that the vital information needed to create a highly accurate voice clone is retained throughout the audio workflow.
In conclusion, understanding the nuanced factors involved in creating quality audio inputs for voice cloning is critical, especially within the confines of a short recording session. By acknowledging the impact of aspects like microphone placement, breathing control, syllable structures, and audio compression, we can produce better training datasets that allow for the generation of higher-quality synthetic voices. The continuous research in this area will contribute to further advancements and an increasingly natural sound in synthetic speech.
Precision Pacing Optimizing Word Count for 10-Minute Voice Cloning Projects - Analyzing Voice Sample Consistency for Improved Cloning Results
When aiming for improved voice cloning outcomes, carefully examining the consistency within voice samples becomes critical. High-quality recordings are a good starting point, but ensuring a speaker's vocal characteristics remain uniform across the sample set is equally important. The nuances of speech—including emotional expression, speaking pace, and even the subtle changes introduced by the recording environment—can greatly influence the cloning process. If these elements aren't consistently represented in the voice samples used for training, it can limit the ability of voice cloning models to accurately replicate a speaker's unique voice.
Researchers are increasingly focusing on standardizing the approach to gathering and processing audio samples. This effort emphasizes consistency across all aspects of sample collection—from microphone placement to room acoustics. Through this type of disciplined approach, cloning models can more accurately capture the full range of a speaker's vocal identity. This precision in sample preparation leads to more reliable and accurate voice clones, a feature of particular importance when considering applications like audiobooks and podcasts where maintaining a listener's engagement through natural-sounding speech is crucial. The pursuit of uniform voice samples is thus a key area of ongoing refinement in the development of voice cloning technology.
Analyzing the consistency of a voice sample is crucial for achieving better voice cloning results. The emotional consistency during recording seems to have a direct impact on the cloning outcome. If the emotional content varies greatly, the cloning algorithms can struggle to produce a natural sounding synthetic voice. This highlights the need for controlled, stable delivery during the recording phase.
The concept of "phonetic density" also appears to play a vital role in the cloning process. It seems that having a wider variety of sounds in a given period improves the model's understanding of a speaker's voice, which could lead to more authentic-sounding clones. This is further supported by the observation that cloning systems often encounter challenges when trying to distinguish between similar vowel sounds. Providing a greater variety of vowel sounds in the training data appears to significantly improve the clarity of the resulting synthetic speech.
Interestingly, the characteristics of the microphone used can introduce subtle distortions. For example, a microphone's frequency response can make certain parts of the voice more pronounced while others may be dampened. This emphasizes the importance of microphone selection in the initial recording phase. Creating a diverse dataset, incorporating various speaking styles and patterns, leads to improvements in cloning fidelity. This is a step toward developing voice cloning that can be more adaptable to different voices and speakers.
The rhythmic and intonation patterns of speech, also known as prosody, seem to heavily influence the resulting clones. Cloning models that are trained with attention to these prosodic features tend to create synthetic voices that are more lifelike and emotionally expressive. Additionally, the ideal microphone placement isn't a fixed position. Different distances and angles can lead to varying results in the captured voice, impacting the quality of the final clone.
Lossy audio compression, if used carelessly, can introduce artifacts that hinder the original voice's quality. This highlights the need to use lossless formats in voice cloning to avoid the loss of key vocal features. Similarly, consciously applying breathing techniques such as diaphragmatic breathing during recordings can promote a more steady vocal output, which, in turn, contributes to a more natural-sounding cloned voice.
The acoustic properties of the room where the voice is recorded can also introduce complexities. If the room has a lot of hard surfaces that cause reflections, it can degrade the audio quality. Careful management of these reflections by using sound-absorbing materials can result in a much cleaner recording and improves the accuracy of the cloning process. These are just some of the interesting insights that are emerging as we continue to refine voice cloning techniques and explore how to produce the best possible clones. As the technology continues to develop, the ability to manage these subtleties will become even more important for producing the next generation of realistic synthetic voices.
Get amazing AI audio voiceovers made for long-form content such as podcasts, presentations and social media. (Get started for free)
More Posts from clonemyvoice.io: