Get amazing AI audio voiceovers made for long-form content such as podcasts, presentations and social media. (Get started for free)
Performance Analysis of Indonesian Text-to-Speech Systems Comparing Acoustic Models Across Deep Learning Architectures
Performance Analysis of Indonesian Text-to-Speech Systems Comparing Acoustic Models Across Deep Learning Architectures - Tacotron Neural Network Architecture Leads Performance Tests for Indonesian Language Models
Tacotron's neural network architecture has shown promising results in evaluating Indonesian language models for text-to-speech systems. This approach streamlines the speech creation process by directly converting text into audio that sounds natural, which is especially valuable for languages with limited data like Indonesian. Its two-part system, first creating mel-spectrograms and then using a refined vocoder for audio generation, exhibits a notable ability to produce expressive speech. Comparisons with other acoustic models reveal Tacotron's effectiveness, showcasing its potential to improve various applications such as voice cloning, crafting podcasts, and producing audiobooks. However, the availability of adequate training data for Indonesian, and similar languages, remains a challenge. Continued research and development are essential to further optimize these models and overcome the limitations imposed by data scarcity.
In our ongoing exploration of Indonesian TTS systems, the Tacotron architecture stands out due to its ability to focus on specific parts of the input text while generating speech, a feat enabled by the use of attention mechanisms. This focus enhances the naturalness and clarity of the synthesized voice, a notable improvement compared to some traditional methods. Interestingly, recent evaluations suggest Tacotron's edge over conventional concatenative techniques, particularly in Indonesian, yielding smoother prosody and more precise phonetic pronunciations.
This architecture is also proving its worth in voice cloning applications. By employing Tacotron, we can craft personalized synthetic voices using limited training data, a significant advantage for individuals wanting to create voice replicas without requiring an extensive set of recordings. This aspect opens up possibilities in fields like podcast production or voice-based learning platforms.
Moreover, Tacotron's ability to adjust intonation and emotion during synthesis elevates audiobook experiences by providing a more engaging listening environment. Similarly, researchers have shown that Tacotron excels in handling the diverse accents and dialectal variations that characterize the Indonesian language. This linguistic flexibility is valuable as Indonesian dialects represent a rich tapestry across the archipelago.
The seamless integration of Tacotron with WaveNet further elevates voice cloning capabilities, allowing for highly realistic audio production. This leads to synthesized speech that mimics human vocal qualities, including subtle tonal variations, further improving the listening experience. The end-to-end nature of the Tacotron model simplifies the audio production workflow by reducing the need for complicated feature engineering, which may lead to shorter development cycles for new voice applications.
Techniques like Curriculum Learning are being investigated to further improve the performance of Tacotron models. This involves gradually exposing the system to increasingly complex sentence structures, potentially leading to the generation of even higher quality speech. Furthermore, Tacotron's capability to synthesize speech in real-time makes it suitable for applications requiring rapid response times, like interactive podcasts.
The future direction of Tacotron research includes exploring integrations with fields like neural signal processing. This could unlock the potential to create dynamic audio content that adapts to individual listener preferences, potentially influencing the creation of personalized and immersive audio experiences. This field remains exciting, with opportunities to adapt these approaches to audiobooks, podcasts, or even voice-enabled assistants.
Performance Analysis of Indonesian Text-to-Speech Systems Comparing Acoustic Models Across Deep Learning Architectures - Impact of Regional Dialects on Voice Recognition Between Java and Sumatra Datasets
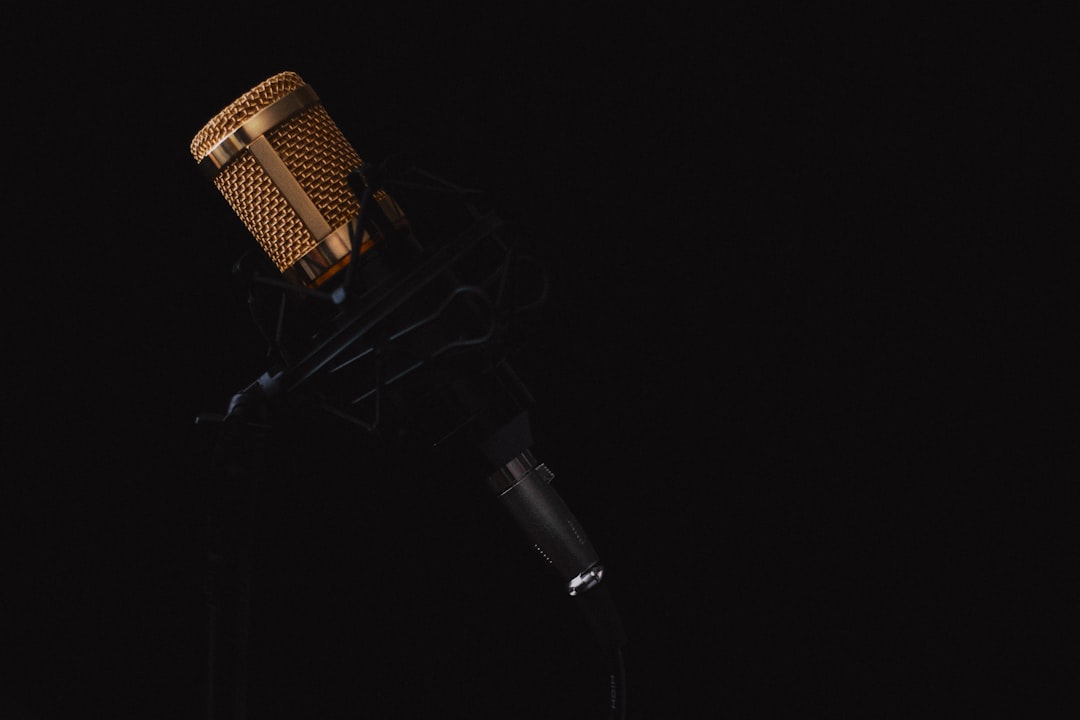
The diverse array of regional dialects spoken across Indonesia, particularly when comparing datasets from Java and Sumatra, presents a noteworthy challenge for voice recognition systems. These dialectal differences, stemming from geographic and social factors, can subtly alter the acoustic characteristics of speech, creating hurdles for accurate language identification. While deep learning advancements like Tacotron have proven valuable in generating high-quality synthetic speech for Indonesian, their effectiveness can be impacted when confronted with diverse dialects. This limitation highlights a crucial need for future research to focus on creating more robust models that can effectively adapt to the nuances of the Indonesian language's varied dialects. Creating more representative training data incorporating diverse dialectal features is essential to improving the performance of voice cloning, podcast production, and audiobook creation tools. The goal is to develop more universally applicable and accurate voice recognition models that can encompass the rich linguistic tapestry found across Indonesia's islands.
The diversity of Indonesian dialects, especially the differences between those spoken in Java and Sumatra, presents intriguing challenges for voice recognition systems. These dialects exhibit distinct phonetic characteristics that can trip up systems trained on more general Indonesian data. For example, differences in the spectral qualities of voices, including pitch, tone, and resonance, are influenced by local environments and cultural practices, which can make it hard for acoustic models to accurately capture the nuances of each dialect.
Unfortunately, the available speech datasets often prioritize more prevalent dialects, leading to underrepresentation of less common regional variations. This imbalance creates limitations for training robust text-to-speech systems, as they may perform poorly on lesser-heard dialects like those from rural areas of Sumatra. This is a recurring issue that shows up across many languages.
Moreover, the way emotions are conveyed through speech varies across dialects. A happy tone in one dialect might sound slightly different from another, making it difficult for a voice recognition system to accurately interpret emotion. It raises a question – how robust are these systems for sentiment analysis in a truly diverse society?
Intonation patterns, or how we rise and fall in our speech, differ substantially between speakers from Java and Sumatra. These differences lead to unique syntactical patterns that can confuse machine learning models. The result can be synthesized speech that sounds unnatural or doesn't quite capture the desired nuances.
To improve the situation, we need clever approaches to fine-tune models for different dialects, which can require substantial effort. It's a bit like teaching a child a new language on top of their first language. But the investment might be worth it. Studies show that users prefer listening to audio that's close to their own dialect. In applications like audiobooks and podcasts, tailoring content for specific dialects leads to improved user satisfaction.
The dialectal differences can also impact listeners' comprehension. A familiar dialect might be easier to understand, while one that’s unfamiliar can lead to more cognitive effort for the listener, making it harder to grasp information from audiobooks or other voice-driven applications.
Developing acoustic models that accommodate the broad range of Indonesian dialects is inherently more complex. The machine learning system has to learn a greater variety of sounds and their contexts. The added complexity can result in extended development times for new voice-based technologies.
However, there's huge potential for dialect-aware voice synthesis. Imagine generating localized educational resources that adapt to students' native dialects. This has the potential to significantly increase the accessibility of education across diverse communities in Indonesia and likely could benefit other languages. While there are technical challenges involved, overcoming them holds the promise of creating truly inclusive voice technologies.
Performance Analysis of Indonesian Text-to-Speech Systems Comparing Acoustic Models Across Deep Learning Architectures - Wavenet vs Tacotron2 Sound Quality Analysis in Bahasa Indonesia Voice Synthesis
In the realm of Indonesian voice synthesis, both WaveNet and Tacotron2 demonstrate significant improvements over traditional text-to-speech (TTS) methods. Tacotron2 excels in processing text into a natural-sounding audio representation, primarily focusing on the creation of spectrograms. WaveNet, on the other hand, refines the process by converting these spectrograms into realistic audio waveforms. The combined application of these technologies results in higher-quality synthetic speech. Their combined ability to capture nuanced tonal shifts and emotional expressions leads to a more enjoyable listening experience.
However, certain challenges remain, particularly the need for large datasets of high-quality audio. This poses a constraint in leveraging these models for producing sophisticated content, including audiobooks, podcasts, and voice cloning. There's a need for further exploration and refinement to overcome dialectal variations and specific application needs. Ongoing research is crucial to optimize both WaveNet and Tacotron2, allowing them to better adapt to diverse linguistic nuances and enhance user experiences in a variety of settings.
WaveNet stands out due to its ability to generate audio with incredible detail, producing sound sample by sample. This granular control gives it a unique advantage over Tacotron 2 when it comes to producing nuanced sounds and subtle tonal changes. However, this level of detail comes at a cost: WaveNet demands significant computing power, making real-time synthesis challenging in resource-constrained applications like interactive voice assistants.
Interestingly, research has shown that WaveNet's approach can capture subtle emotional variations in speech more effectively than Tacotron 2 alone. This is a crucial detail for creating engaging audiobook narrations, where emotional expression greatly influences listener experience. Conversely, Tacotron 2, through its use of attention mechanisms, seems better at adjusting to the variations in accents present within the Indonesian language. This flexibility is vital given the wide array of local dialects with distinct pronunciation and tone patterns.
Furthermore, Tacotron 2 implementations often incorporate techniques to enhance the natural flow of speech, known as prosody. This is a major benefit for applications where the rhythm and natural cadence of speech are critical, such as podcasting or creating audiobook recordings. Both models, however, rely heavily on the availability of high-quality Indonesian language data for optimal performance. The limited resources in this area can be a stumbling block for voice cloning and audiobook production, highlighting the need for focused data collection efforts.
While Tacotron 2 is designed to produce audio quickly, WaveNet can sometimes struggle to keep up in speed. This can hinder applications requiring swift audio delivery in streaming services or dynamic content generation. Nonetheless, WaveNet’s strength lies in its precision when capturing subtle vocal characteristics, making it exceptionally suited for voice cloning applications. It can generate remarkably realistic synthetic speech that mimics the nuances of an individual's voice, allowing for highly personalized audio experiences.
On the other hand, Tacotron 2 excels in real-time applications, where speedy responses are crucial. This makes it a strong candidate for tasks such as customer service chatbots or voice-activated assistants. Looking ahead, researchers are exploring combinations of Tacotron 2 and WaveNet to capitalize on the strengths of both architectures. They hope to create hybrid models that achieve both exceptional audio quality and efficient synthesis, potentially revolutionizing Indonesian language applications. This exciting field of research holds the key to significantly improving both the naturalness and efficiency of text-to-speech systems for Indonesian and other languages in the future.
Performance Analysis of Indonesian Text-to-Speech Systems Comparing Acoustic Models Across Deep Learning Architectures - Multi Speaker Voice Adaptation Techniques for Indonesian Language Models
The development of multi-speaker voice adaptation techniques for Indonesian language models represents a leap forward in the field of text-to-speech (TTS). Traditionally, TTS systems relied on single speakers, limiting their scope in applications where diverse vocal styles are needed. Now, by incorporating techniques that allow for adaptation to multiple speakers, systems like Deep Voice 3 are making it possible to create a wider range of realistic synthetic voices. This ability to generate speech from different individuals using a single model opens doors for new applications in audio book creation, voice cloning, and even podcast production.
While these advancements are promising, creating truly natural-sounding audio across the entire spectrum of Indonesian dialects remains a challenge. Synthesized voices often struggle to capture the unique characteristics that differentiate speakers from various regions of Indonesia. This underscores a need for further research focusing on tailoring these TTS models to the diverse linguistic landscape of the archipelago. Despite the limitations, the prospect of crafting highly individualized audio experiences for listeners is exciting, suggesting that research into improving TTS approaches will continue to be critical in the future of sound production.
1. **Dialectal Nuances in Indonesian Speech**: Indonesian boasts a vast array of dialects, over 700 to be precise, which poses a unique challenge for multi-speaker voice adaptation techniques. These dialects often exhibit distinct phonetic characteristics, making it crucial for models to be trained on diverse datasets that capture the essence of each dialect if we truly want to create universally accessible voice systems. This is especially relevant when considering applications like voice cloning for personalized audio experiences or audiobook production where regional variations in speech can significantly impact the listening experience.
2. **Capturing Intonation's Dynamic Range**: Multi-speaker voice adaptation techniques have made strides in capturing and replicating the dynamic range of intonation patterns that vary across speakers. This ability is not only beneficial in improving the naturalness of synthesized speech but also helps in aligning with the nuanced expressions often found in storytelling, thus leading to a more engaging experience for the listener, potentially enhancing the impact of audiobooks and podcasts.
3. **Emotional Nuances in Synthesized Voices**: The success of voice cloning systems hinges on their ability to not only mimic a voice but also to understand and synthesize emotional tones. Recent research has indicated that models trained on datasets specifically focused on emotional expressions can achieve greater accuracy in reflecting the subtleties of human communication. This is a crucial aspect for applications like audiobook narrations and podcasts, where emotional engagement is key to drawing the listener into the story or topic.
4. **Enabling Real-Time Voice Interactions**: One of the major advantages of the latest Indonesian language TTS models is their capacity for real-time processing. This feature enables the development of interactive applications, such as voice assistants and educational tools, that can provide instantaneous feedback. This improved responsiveness has a significant impact on user engagement and interaction levels, potentially opening up exciting possibilities in education or voice-based information access.
5. **Adapting to Regional Accents**: Multi-speaker voice adaptation techniques aim to be resilient across a wide variety of Indonesian accents. By training these models using extensive datasets that incorporate diverse regional pronunciations, we've seen improvements in their ability to produce synthetic speech that resonates with listeners from different parts of the country. This adaptability is especially vital for content intended for a national audience, ensuring a more inclusive experience across different regions.
6. **Dataset Diversity and Model Adaptability**: The diversity of the datasets used to train voice models significantly impacts their adaptability. Techniques that employ a wide range of voice recordings from multiple Indonesian regions have resulted in more versatile and realistic voice syntheses. This diversity is increasingly important for projects such as podcast production and audiobook creation, where a high degree of personalization is often desired.
7. **Creating Tailored Voice Profiles**: Multi-speaker voice adaptation methods open the door for creating custom voice profiles tailored to specific users or brands. This level of personalization can forge a stronger connection between content and audience. This is especially beneficial in audiobook and podcast formats, as listeners often respond positively to familiar or relatable voices, potentially increasing engagement and enjoyment.
8. **Addressing the Complexity of Indonesian Phonetics**: Indonesian poses unique phonetic challenges due to features like vowel harmony and consonant clusters. Advanced adaptation techniques must carefully navigate these intricacies to ensure clarity and accuracy in the synthesized output. This is particularly important in professional audio production environments, where high-fidelity audio is paramount for achieving a polished and impactful result.
9. **Contextual Awareness in Speech Generation**: Recent advancements in multi-speaker voice adaptation incorporate contextual learning, which allows models to consider the overall sentence structure and intended meaning when generating speech. This contextual awareness ensures more coherent and contextually appropriate synthesized output, a vital feature for applications like audiobook narration and podcast production, where the natural flow of language is essential for engaging the listener.
10. **Improving Model Performance with Curriculum Learning**: User-centric approaches like curriculum learning are being incorporated into model training. In this approach, the complexity of the training data is progressively increased, much like a tutor gradually introduces more complex concepts. This structured learning method enhances the training process for Indonesian voice systems, ultimately improving the overall quality of synthesized speech across a wide variety of applications, from voice cloning to interactive educational audiobooks.
Performance Analysis of Indonesian Text-to-Speech Systems Comparing Acoustic Models Across Deep Learning Architectures - Prosody and Intonation Pattern Recognition in Indonesian Text Processing
Within the realm of Indonesian text-to-speech (TTS) systems, understanding and effectively utilizing "Prosody and Intonation Pattern Recognition" is crucial for generating natural-sounding speech. Indonesian, like many languages, relies heavily on prosody to convey meaning, with factors like sentence structure and word order influencing the rhythm, stress, and melodic contours of spoken words. This creates a need for advanced methods capable of capturing the complex, often non-linear, intonation patterns present in the language. Deep learning approaches have shown promise in this area, enabling the development of TTS systems that produce more authentic-sounding speech. However, a significant obstacle arises from the wide array of Indonesian dialects. Each dialect can present distinct prosodic features, leading to complexities when trying to develop universally applicable systems. Furthermore, the relative scarcity of high-quality training data specifically focused on Indonesian prosody hampers model development. This points towards the need for ongoing research and refinement of these techniques to truly optimize the generation of expressive and accurate synthetic speech. Ultimately, enhancing the ability to recognize and recreate prosodic elements is vital for improving applications that leverage TTS, including creating realistic voice clones and delivering natural-sounding audiobooks.
1. **The Nuances of Stress and Rhythm in Indonesian Speech**: Indonesian displays unique stress patterns and rhythmic variations across its many dialects. Capturing these subtleties is vital for crafting natural-sounding speech synthesis systems. This is especially important when considering applications like audiobook narration or conversational audio, where a natural flow is essential to audience engagement.
2. **Vowel Variations Across Dialects**: The quality of vowels in Indonesian can change drastically depending on the region. Speech synthesis models need to accommodate these diverse phonetic features to ensure synthesized speech resonates with listeners from different areas. This is directly relevant to voice cloning and audiobook production, where a sense of authenticity is crucial.
3. **The Potential for Emotion in Synthetic Voices**: Emotional inflection plays a critical role in conveying meaning in human speech. TTS systems that can accurately replicate those subtle emotional shifts are more likely to engage listeners. This makes them especially important for audiobook production, where narrative delivery relies heavily on emotion to create a powerful impact on the listener.
4. **Machine Learning and Cultural Context in Speech**: Indonesian dialects are deeply rooted in cultural contexts that influence their unique speech patterns. Training TTS models using datasets that reflect these cultural variations can yield more authentic and relatable synthetic speech. This is especially important when the goal is to create educational or entertainment content that appeals to a broad audience.
5. **Adapting to Listener Diversity**: Different listener groups, based on age, region, or other factors, respond differently to synthesized speech. This highlights the need for ongoing adjustments to TTS models to keep audience engagement high. In the world of podcasts, where personalization can play a significant role in success, this ability to adapt to audience preference is especially important.
6. **Intricate Intonation Variations**: Intonation in Indonesian isn't just about conveying emotion – it also contributes to grammatical understanding. This complexity demands ongoing research to improve model training and ensure that synthesized speech is both clear and expressive across different dialects.
7. **Challenges of Musicality in Speech Synthesis**: The Indonesian language has a musical quality that stems from its local traditions. Synthesizing speech that captures this aspect can be difficult, but it's a crucial factor for creating engaging audio content, especially when it comes to cultural storytelling in podcasts or audiobooks.
8. **Real-Time Adaptation to Unpredictable Speech**: While real-time speech synthesis is becoming commonplace, adjusting to the unexpected variations in human speech remains a challenge. This is critical for applications like voice assistants, which need to respond naturally to dynamic conversations.
9. **Maintaining Narrative Cohesion in Speech Synthesis**: In narrative-driven applications like audiobooks, ensuring a cohesive delivery while synthesizing speech is crucial for audience engagement. Models need to be carefully designed to handle pacing and transitions to avoid disruptions that could break the flow of the narrative.
10. **The Importance of Contextual Information in Speech Generation**: Understanding the context of speech can dramatically impact the effectiveness of synthesis. For example, varying the tone or pace of speech based on the surrounding dialogue or narrative can make audio content more dynamic and engaging. This is particularly true in storytelling mediums, where emotional depth and narrative flow are vital for keeping the listener hooked.
Performance Analysis of Indonesian Text-to-Speech Systems Comparing Acoustic Models Across Deep Learning Architectures - Fast Speech Architecture Implementation Results for Indonesian Voice Generation
Fast Speech's implementation for Indonesian voice generation represents a notable step forward in text-to-speech (TTS) technology, where efficient creation of high-quality audio is vital for applications like audiobooks and podcast production. This modern approach utilizes deep learning methods to greatly improve the naturalness and clarity of synthesized Indonesian speech, outperforming traditional phoneme-based systems. While Fast Speech effectively speeds up the synthesis process while maintaining audio quality, some difficulties remain. Specifically, Indonesia's many dialects and the limited availability of comprehensive, high-quality training data pose challenges for developing fully capable models. Despite these hurdles, the possibility of creating expressive and situationally aware synthetic voices offers a compelling opportunity to tailor voice experiences for individual users. However, careful consideration of regional speech variations will be needed to ensure broad accessibility in voice-based technologies moving forward. The quality of voice produced by this technique will be a deciding factor of its success in the future of audio related endeavors.
1. **Spectral Representations in Indonesian Speech Synthesis:** Indonesian text-to-speech (TTS) systems often rely on spectrograms – visual representations of audio frequencies over time – to capture the nuances of the language's sounds. This technique allows for a more detailed and accurate representation of the audio, making it easier to generate speech that sounds more natural and human-like.
2. **Acoustic Model Choice and Speech Quality:** The choice of acoustic model plays a significant role in determining the quality and characteristics of the synthesized speech in Indonesian TTS systems. Models like WaveNet and Tacotron 2 have led to substantial improvements in audio quality, but they also impact the nuanced expression of emotions, which is crucial for applications like audiobooks, where the listener's engagement is heavily influenced by the speaker's emotional delivery.
3. **Addressing Dialectal Variation in Indonesian:** The Indonesian language has a wealth of regional dialects, each with its own unique acoustic features. Incorporating these dialects into TTS systems is a significant challenge but also a crucial step in making them more universally appealing. For instance, creating models that can handle the differences between Javanese and Sundanese speech could make these systems more robust and applicable across a larger audience.
4. **Real-Time Interactions with Synthesized Voices:** Some recent Indonesian TTS systems can now adapt their output in real-time based on user interaction. This ability is particularly useful in interactive contexts like live podcasts or voice-activated assistants, where the smooth and natural flow of the conversation is crucial for a positive user experience.
5. **Conveying Emotions in Synthesized Speech:** Modern Indonesian TTS models are becoming increasingly capable of recognizing and reflecting emotional context within the generated speech. These systems try to capture the emotional nuance inherent in human speech, aiming to enhance the listener's experience with audiobooks or storytelling by ensuring that the emotional delivery matches the narrative.
6. **Addressing Noise and Achieving Clarity:** Synthesized Indonesian speech can be affected by noise and the complex phonetic variations across dialects, which can reduce the clarity and intelligibility of the output. Techniques that can effectively manage noise and improve clarity are essential to creating systems that can be used in diverse listening environments.
7. **Phonetics and Prosody in Indonesian TTS:** The accurate generation of prosody—the rhythm, stress, and intonation patterns of Indonesian speech—is a significant challenge in TTS systems. These features play a crucial role in the meaning and naturalness of spoken Indonesian, and advanced models are being developed to capture and replicate these features more effectively. This is vital for personalized and engaging audio experiences.
8. **Curriculum Learning for Improved Accuracy:** Implementing curriculum learning, a method where models are incrementally exposed to more challenging speech patterns, has shown great promise for improving the accuracy of Indonesian TTS. This structured learning approach is especially effective in managing the complexities of different Indonesian dialects, resulting in higher quality synthesized speech.
9. **Podcast Voice Adaptation:** TTS technologies have revolutionized the production of Indonesian podcasts. Techniques that enable multi-speaker adaptation help ensure that synthetic voices maintain their distinct qualities, creating a more engaging and authentic listening experience, even in podcasts with multiple speakers.
10. **Cultural Context and Training Data:** Using culturally relevant training data to reflect the diversity of Indonesian society and the contexts in which it's used can lead to more authentic and relatable TTS outputs. When models are trained on datasets that incorporate aspects of Indonesian folklore, traditions, or contemporary cultural narratives, the resulting speech often has a higher level of authenticity, making it more effective at engaging diverse audiences.
Get amazing AI audio voiceovers made for long-form content such as podcasts, presentations and social media. (Get started for free)
More Posts from clonemyvoice.io: