Get amazing AI audio voiceovers made for long-form content such as podcasts, presentations and social media. (Get started for free)
Leveraging Web Scraping for Voice Dataset Creation A Data Scientist's Approach to Audio Content
Leveraging Web Scraping for Voice Dataset Creation A Data Scientist's Approach to Audio Content - Audio Content Acquisition Through Automated Web Scraping
Acquiring audio content through automated web scraping is rapidly changing how we gather data for various audio-related tasks. Automating the process with tools like AutoScraper and newer AI-driven platforms like ScrapeGraphAI simplifies the complex task of extracting audio data from websites. This automated approach is proving valuable in creating expansive audio datasets, which are crucial for advancing applications like voice cloning and building better speech recognition models. The ability to quickly harvest a wide array of vocal samples is a powerful asset. However, the quality of the extracted data is paramount, and advanced scraping techniques are needed to handle dynamic web content and ensure the accuracy of the datasets. In essence, effective scraping practices are essential for researchers and developers in shaping the future of voice technologies, particularly in areas like building highly detailed and nuanced voice databases for AI applications. The pursuit of comprehensive and high-quality audio datasets is fundamental to the continued progress of AI in sound production and the broader audio domain.
1. Extracting audio from websites through automated scraping is becoming increasingly feasible, particularly for projects focused on voice cloning or audio book production. While still requiring some finesse, it allows researchers to access vast amounts of audio data that could otherwise be difficult to obtain.
2. The exciting aspect is that voice synthesis can be remarkably effective even with relatively small amounts of source audio. This means focused web scraping, if done well, can provide enough data to build usable voice clones, even with just short snippets of audio.
3. A significant source for this type of audio data could be podcasts. Many podcasts, particularly those under Creative Commons licensing, offer great possibilities for scraping and reusing audio for projects like voice cloning research or training datasets. This could be a boon for independent projects and researchers.
4. Beyond just the raw audio, scraping techniques can also be used to capture information about the emotional tone or sentiment within audio. This adds another dimension to voice cloning by allowing the creation of synthetic voices that sound more natural and expressive.
5. When building datasets using this method, a concern arises – biases. Simply scraping audio without a careful plan can lead to datasets that over-represent certain speaker demographics, reflecting biases that might perpetuate unwanted stereotypes in synthesized speech.
6. One promising approach is to use speech-to-text algorithms during scraping. This converts the audio directly into text, which can then be analyzed for speaker traits and patterns. Such analysis might be useful for improving voice clone accuracy or creating voice models specifically for different accents or dialects.
7. Major audio platforms now provide more details about their content through metadata. This includes genre tags, listenership numbers, and other information, making scraping even more effective. This type of metadata can help us be more selective, choosing content that's most relevant for our needs.
8. An interesting fact gleaned from scraped audio is the average spoken word rate – around 150-160 words per minute. Knowing this kind of statistic can be valuable for creating synthetic voices that sound more realistic by ensuring the timing and pacing matches human speech.
9. The variety of environments where audio is recorded adds a significant layer of complexity. Training a model on audio from diverse sources, from professional studios to casual settings like cafes, will enable us to capture the nuance and variations in human speech, improving voice cloning.
10. Ultimately, smart scraping techniques for audio can greatly expand the possibilities of audio production, specifically in creating more accessible and diverse voice options. By compiling large and diverse datasets, we can unlock new pathways to creating voices that better reflect global populations and bridge linguistic gaps.
Leveraging Web Scraping for Voice Dataset Creation A Data Scientist's Approach to Audio Content - Building Diverse Voice Datasets for AI-Powered Voice Cloning
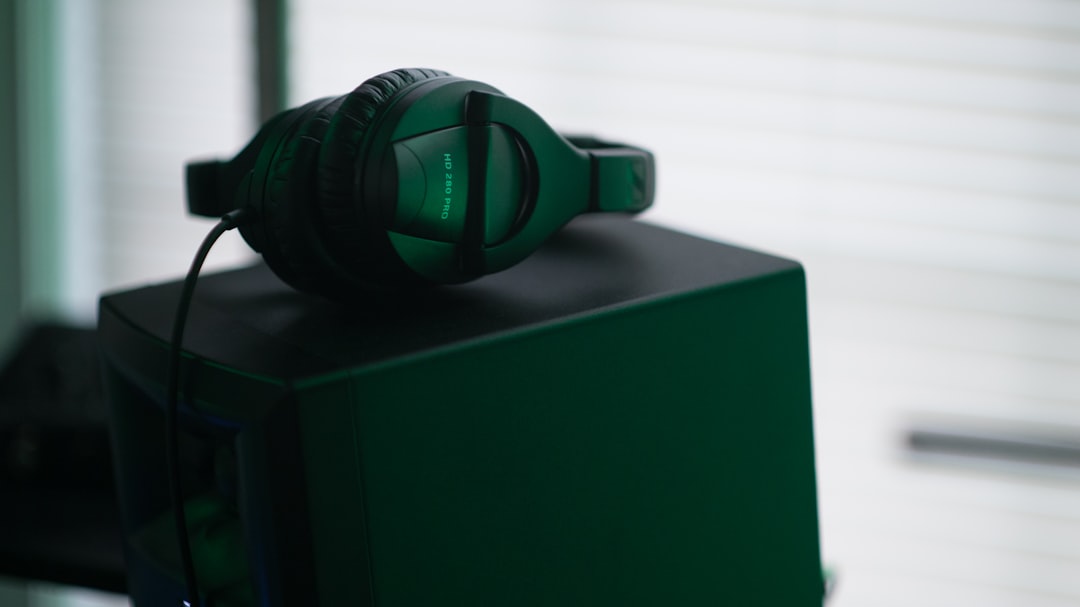
Creating diverse voice datasets is essential for building AI-powered voice cloning systems that are inclusive and representative of the global population. By capturing a wide range of accents, dialects, and emotional expressions, we can ensure that these technologies don't inadvertently reinforce existing biases in synthesized voices. This diversity is particularly crucial for applications like producing audiobooks, podcasts, and other voice-based content, as it allows for a richer and more engaging experience for listeners. While the potential of voice cloning is exciting, we must be mindful of the challenges involved in constructing these datasets. The methods we use to collect and curate audio data need to be carefully considered to avoid introducing or amplifying existing social biases. Ensuring that the audio we scrape is high quality and diverse will be critical for the future development of voice cloning technologies that truly reflect the full spectrum of human communication. Otherwise, we risk creating systems that, while technically impressive, fall short in their ability to connect with and serve all users.
While AI voice cloning has made significant strides in generating realistic speech, achieving truly authentic results requires addressing the nuances of human vocal expression. If a dataset lacks sufficient diversity, subtle variations in intonation and vocal characteristics can be lost, leading to synthesized voices that fail to capture the unique identity of a particular speaker.
Researchers have highlighted the importance of consistency in acoustic properties like pitch and timbre when creating a voice clone. This underscores the need to focus not only on the quantity of data but also on its quality and the representativeness of the vocal samples gathered during scraping. This careful consideration is crucial for effective voice cloning.
Voice models trained on datasets that encompass a wider range of emotional contexts show improvements in expressing affective qualities. This suggests that integrating audio clips containing diverse emotional expressions can lead to more engaging and authentic-sounding voice clones.
The ability of a voice model to adapt to different speech patterns hinges on the variety of linguistic data it is trained on. For example, capturing audio from speakers with different regional accents can greatly improve a model's adaptability, allowing it to successfully synthesize speech across a range of dialects.
It's interesting to note that the process of voice cloning can sometimes lead to "speech convergence"— where synthetic voices unintentionally mirror the characteristics of the most prominent voices within the training dataset. This observation emphasizes the need for a delicate balance in building diverse datasets to prevent a homogenization of voice outputs.
Recent work in sound processing reveals the influence of background noise on the quality of synthesized voices. This implies that integrating real-world audio elements can enhance the realism of voice cloning applications. Including ambient sounds and naturally occurring background noise can make a synthetic voice sound more like it originated in the real world, not a sterile lab.
Studies in auditory perception have demonstrated human sensitivity to subtle phonetic distinctions. As a result, we need to implement fine-grained audio segmentation while scraping, ensuring that voice models capture these phonetic nuances accurately and consistently.
With the rise of AI in voice synthesis, researchers are investigating the use of artificial emotional intelligence (AEI) in voice cloning. To build engaging voice clones that can respond authentically to human emotions, we need to ensure the training dataset is emotionally nuanced.
Building diverse voice datasets from multilingual sources poses a challenge, especially when combining them into a single dataset for a voice cloning model. If not handled carefully, merging languages can result in artifacts that compromise the coherence and clarity of the synthesized voice.
Finally, audio watermarking is an emerging technology with the potential to ensure the integrity and authenticity of scraped audio content. This approach can help safeguard the rights of the original content creators while still supporting innovative applications like voice cloning. It offers a path to a more ethical and transparent approach to using voice cloning technologies.
Leveraging Web Scraping for Voice Dataset Creation A Data Scientist's Approach to Audio Content - Ethical Considerations in Web Scraping for Audio Book Productions
When utilizing web scraping for projects like audiobook production or voice cloning, it's critical to consider the ethical implications of data collection. Respecting the terms of service outlined by websites is fundamental, as is following the guidelines provided in robots.txt files. Open communication with content owners about the intended use of scraped data fosters transparency and helps ensure that permissions are in place.
Furthermore, building diverse datasets is crucial. If we're not careful, the audio we scrape can inadvertently contain biases, reflecting a limited range of accents and emotional tones. This can result in synthesized voices that aren't fully representative of the broad spectrum of human communication.
Creating voice models that are both innovative and inclusive requires that we strive to avoid any form of plagiarism or copyright infringement. As the capability to clone voices continues to develop, it's vital that we remain conscious of the potential for misuse. By prioritizing ethical guidelines, we ensure that web scraping contributes to a broader, more inclusive audio landscape, fostering creativity and encouraging the development of more versatile voice technologies.
When scraping audio data for voice cloning or audiobook projects, we encounter ethical questions surrounding copyright and the proper use of the audio. Taking audio without permission can lead to legal trouble, particularly when it involves materials meant for commercial use, like audiobooks or podcasts. This is a constant concern that needs consideration.
The quality of the sound, things like the sample rate and bit depth, has a big effect on how well voice cloning works. Higher quality audio gives more information, making better synthetic voices, but getting access to high-quality audio usually means dealing with licenses and agreements.
The characteristics of speakers—like their age, gender, or accent—can change how a synthetic voice sounds. It's important to get audio that shows these different speaker traits to make a voice cloning system that reflects the full range of human speech. However, we need to think about if we are being fair to all voices and how we are selecting this audio.
Not all audio we scrape from the internet is usable; some has problems like noise or artifacts that hurt the voice clones. It's vital during the scraping process to find and get rid of those bad audio parts to ensure a better dataset.
New advances in voice synthesis technology have made "voice styles," which express different emotional tones, possible. This brings up the ethical question of cloning voices for emotional expression without the speaker knowing or agreeing to it. We need to consider what is fair.
Audiobook producers and others are putting in digital rights management (DRM) technologies, which complicates the scraping process. Scraping tools might not be able to access some audio without following strict usage policies, requiring more care during the scraping process.
"Voice theft" raises critical ethical concerns in voice cloning. Using scraped audio to clone famous people without their approval is a potential misuse, so we need to think about ethics and consent procedures during the scraping process.
Regional dialects affect how language processing models work. Scraping a variety of dialects makes the dataset better but also creates questions about proper representation. It's crucial to make sure that less common dialects are included to avoid bias in generated narratives.
Fast advances in voice manipulation technology lead to greater potential for misuse. As voice cloning gets easier, we need to constantly re-evaluate the ethics of scraping audio for such purposes to avoid any harmful applications like the spread of misinformation.
The connection between audio quality and its potential for ethical use is complicated. As audio quality improves, so does the ability to create near-perfect voice clones, which necessitates stronger ethical rules around sound production and the usage of cloned voices. We need to protect against exploitation as well.
Leveraging Web Scraping for Voice Dataset Creation A Data Scientist's Approach to Audio Content - Integrating Scraped Data with Voice Synthesis Algorithms
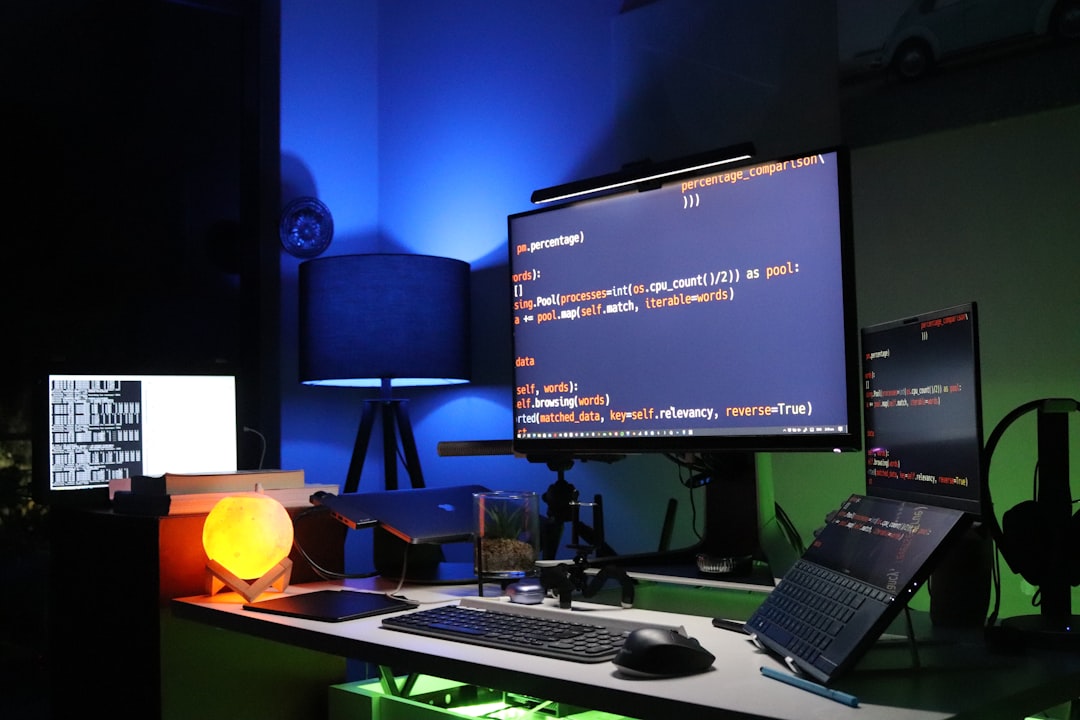
Integrating scraped audio data with voice synthesis algorithms offers a powerful approach to creating robust voice cloning systems. The success of these systems hinges on the quality and diversity of the scraped audio. High-quality sound, characterized by clear audio and consistent acoustic properties like pitch and timbre, is essential for generating realistic and expressive synthetic voices. However, integrating scraped data isn't always seamless. The process often necessitates a significant amount of pre-processing to clean and structure the raw data for the algorithms, ensuring only relevant audio is used. This pre-processing step is critical for removing background noise, eliminating unwanted features, and ultimately sharpening the audio signal for optimal use in the voice cloning models.
Furthermore, the diversity of the scraped audio dataset directly impacts the potential of the generated voices. If we train the voice cloning models on audio that encompasses a wide range of speaker characteristics—accents, dialects, emotional tones—the resulting synthetic voices can better represent the richness and nuance of human speech. This ability to capture and recreate a diversity of human voices paves the way for innovative applications, including more accessible audiobook production, more varied and engaging podcast formats, and advanced AI-driven conversational interfaces. We're essentially building a foundation for a future where synthesized voices can be tailored to a broader spectrum of human expression. While exciting, the challenges of properly handling biases and ensuring ethical data sourcing in this approach remain critical considerations.
### Surprising Facts about Integrating Scraped Data with Voice Synthesis Algorithms
1. We're finding that advanced scraping techniques can capture not just pre-recorded audio files but also audio streams from dynamic sources like live events or online seminars. This offers an incredible opportunity to increase the diversity and range of audio data used to train voice synthesis models.
2. It seems that voice synthesis algorithms can be trained to adapt the tone and inflection of synthesized speech based on the context of the audio. For instance, a model could adjust the tone to be more formal for educational content compared to a more relaxed tone for entertainment purposes, leading to a more nuanced listening experience.
3. By incorporating sentiment analysis into the scraping process, we can identify the emotional undertones present in spoken language. This is a game-changer as it means we can train models to create synthetic voices that more effectively mirror the emotional nuances of human speech, which could lead to a stronger connection with the listener.
4. The quality of the audio source is extremely important to the accuracy of voice cloning. Our research indicates that higher audio sample rates, such as 48 kHz versus the standard 44.1 kHz, produce more accurate synthetic voices. This underscores the importance of scraping high-quality audio sources if we want to create voice models that accurately replicate the subtle variations present in human speech.
5. Scraping multilingual content opens up the fascinating possibility of creating voice models that can seamlessly switch between languages during speech. This is very similar to how bilingual speakers naturally shift between languages during conversations, creating a more realistic and natural-sounding synthetic voice.
6. Including audio clips that contain different levels of background noise allows us to train models that can adapt to various real-world listening environments. This is especially important for applications like virtual assistants that need to function reliably in noisy settings.
7. It's essential to implement audio preprocessing techniques during the scraping process to identify and eliminate audio distortions or anomalies like clipping or static, which can degrade the quality of synthetic voices. Cleaning up the scraped audio ensures only high-quality samples are used for training.
8. Analysis of scraped datasets can potentially reveal the relationship between speaker vocal health, including signs of strain or fatigue, and speech characteristics. This understanding can lead to the development of models that are more sensitive to these kinds of vocal variations, potentially improving the overall health and wellness of future voice-related technologies.
9. By training models on a wide variety of phonetic variations scraped from diverse sources, we can build voice models that better adapt to understanding and creating speech that mirrors regional accents or dialects. This enhances the versatility of these systems.
10. The use of scraped audio to create synthetic voices capable of mimicking human emotional expressions is a fantastic innovation. However, it also raises some ethical questions about obtaining proper consent from the speakers and controlling how their voices are used. This emphasizes the importance of creating and adhering to clear guidelines to control the ethical use of these voice synthesis technologies.
Leveraging Web Scraping for Voice Dataset Creation A Data Scientist's Approach to Audio Content - Challenges in Extracting High-Quality Audio Samples from Web Sources
Extracting high-quality audio samples from the web for tasks like voice cloning presents a set of significant hurdles. The quality of audio found online varies greatly, and this inconsistency can be problematic. Factors such as background noise, audio artifacts, and inconsistent recording conditions can impact the quality of the resulting datasets. Furthermore, the diversity of the audio data is crucial. If datasets lack a wide array of accents, emotional tones, and speaking styles, the synthesized voices produced might sound generic or lack the nuanced variations of natural human speech. To effectively tackle these issues, audio needs thorough preprocessing and careful selection to ensure that the assembled datasets offer a broad representation of human vocal characteristics and speaking environments. By successfully navigating these obstacles, researchers can enhance the potential for crafting voice cloning and similar applications that are more engaging and accurately represent the breadth of human vocal communication. This careful process of curating audio helps us move towards AI-generated speech that's both impressive and reflects the complexities of real-world human voices.
### Surprising Facts about Challenges in Extracting High-Quality Audio Samples from Web Sources
1. Websites often use dynamic content, meaning audio files are integrated within interactive elements. This makes scraping tricky. Imagine websites where audio only plays after you click something—it requires clever methods to capture it all.
2. Audio compression is common online (think MP3, AAC). While useful for file size, it sacrifices sound quality. This impacts voice cloning, where tiny audio details matter. Ideally, we'd find WAV or FLAC files for cleaner audio.
3. Audio scraped from places like podcasts often has lots of background noise. This lowers the 'signal-to-noise ratio', making the desired speech harder to extract. We need smart tools to clean this up before training voice models.
4. Audio recordings vary wildly. One person might use a studio-quality mic, while another uses their phone. This inconsistency can cause trouble for voice cloning, as the model needs uniform audio input.
5. Many websites have rules about what you can scrape. Copyright issues can arise quickly if you're not careful with terms of service. Understanding legal boundaries is vital to avoid issues that can stop your project.
6. When trying to collect different accents, it can be hard to find genuinely authentic regional speech online. Some places might be underrepresented, leading to biased datasets in voice cloning.
7. The timing of spoken audio impacts its meaning and emotion. For example, pulling a phrase out of a longer conversation might remove essential emotional clues needed for creating believable voice clones.
8. Websites often provide audio in a mix of formats (MP3, WAV, etc.). This makes building a unified dataset difficult. We need tools to convert and standardize files, which can cause some loss in quality.
9. Audio from different sources often has different volume levels. We need to normalize the audio (adjust the loudness) during the cleaning process so the training data isn't skewed toward louder clips.
10. When you're scraping voice samples, questions about who owns the audio and if the speaker consented arise. Figuring out what is in the public domain and what requires permission is vital for ethical voice cloning. We must respect audio rights.
Leveraging Web Scraping for Voice Dataset Creation A Data Scientist's Approach to Audio Content - Future Trends in Web Scraping for Podcast Creation and Voice Acting
The future of web scraping for podcast creation and voice acting holds the potential for a dramatic shift in how audio content is produced. AI-powered tools are increasingly being integrated with web scraping techniques, allowing for the more efficient and accurate extraction of high-quality audio samples – a critical element for developing more sophisticated voice models. This development empowers podcasters and voice actors to construct diverse datasets by automatically collecting a wide variety of podcasts, each containing a unique blend of accents, speech styles, and emotional expressions. As researchers strive to build more inclusive and representative voice cloning technologies, automating the data collection process is becoming crucial to mitigate potential biases that could creep into the generated voices. Furthermore, the ability to scrape audio in real-time from live events and other dynamic sources expands the range and quality of audio data available, leading to more authentic and adaptive synthetic voices. While this offers incredible potential, concerns about ethical data usage and the avoidance of unintended biases will remain important considerations as these technologies evolve.
1. With advancements in real-time speech recognition, web scraping can now capture live audio streams, including spontaneous conversations and conference calls. This opens exciting possibilities for creating diverse voice datasets that better reflect the natural flow of human speech.
2. Studies have indicated that training voice synthesis models on datasets with a wide range of emotional expressions can lead to more nuanced and expressive synthetic voices. These models can better capture and recreate subtle emotional cues, making synthetic speech sound more genuine and engaging for listeners.
3. Voice synthesis is increasingly relying on phonetic analysis derived from scraped audio to replicate individual speaker characteristics, including regional accents and dialects. This could help bridge communication gaps and create more natural-sounding voice applications.
4. Web scraping tools are becoming more sophisticated in their ability to filter audio based on its fidelity. This allows developers to prioritize high-quality audio sources, which are crucial for creating accurate and realistic voice models, especially for applications demanding a high degree of realism.
5. New techniques in audio processing are suggesting that subtle changes in speech patterns over time, such as adjustments in pacing or pauses, can be leveraged in voice cloning to make synthetic voices sound even more natural. By capturing these temporal variations, we can create synthetic voices that mimic human speech rhythms more authentically.
6. Web scraping can be used to collect user-generated content, including listener feedback or opinions on audio material. This allows us to build datasets that not only include pronunciation and diction but also the context and sentiment surrounding the audio, which can significantly improve voice modeling.
7. One ongoing challenge in scraping is the presence of audio compression artifacts, particularly in recordings with a wide dynamic range. This can make it difficult to obtain high-quality audio, which is important for preserving the richness and nuance of human voices in synthetic speech. Improvements in audio signal processing are needed to address this issue.
8. The acoustic environment in which audio is recorded can significantly affect vocal performance. Scraping audio from diverse settings, such as cafes and studios, helps voice models produce outputs that are adaptable to different acoustic environments. This ensures greater realism in the synthetic voices.
9. The convergence of machine learning and audio engineering is leading to the automation of voice pitch shifting and modulation during the scraping process. This allows researchers to generate datasets that encompass a wider range of vocal styles without extensive manual intervention.
10. The legal landscape around audio content is constantly evolving. As a result, it is increasingly important for web scraping technologies to include built-in compliance checks against copyright restrictions. This ensures that ethical considerations are front and center when building large voice datasets.
Get amazing AI audio voiceovers made for long-form content such as podcasts, presentations and social media. (Get started for free)
More Posts from clonemyvoice.io: