Get amazing AI audio voiceovers made for long-form content such as podcasts, presentations and social media. (Get started for free)
Let's Try Something Different Exploring Unconventional Voice Cloning Techniques in 2024
Let's Try Something Different Exploring Unconventional Voice Cloning Techniques in 2024 - Acoustic Metamaterials in Voice Synthesis
Acoustic metamaterials represent a novel approach in voice synthesis, surpassing the limitations of conventional materials in sound manipulation. These materials can precisely control sound waves, leading to improved voice capture, particularly in environments with background noise. This translates into better voice recognition and clarity for applications like voice cloning or podcast production. An interesting aspect is their ability to enhance sound quality while simultaneously absorbing unwanted noise without adding significant weight, a significant advantage in device design. Current research is using data-driven methods to fine-tune these metamaterials, allowing for precise control of sound characteristics. This ability to shape sound with unprecedented accuracy holds immense potential for refining human-machine interactions, especially in demanding audio scenarios. While still a developing field, acoustic metamaterials have the potential to revolutionize voice synthesis methods, potentially leading to new and more sophisticated voice cloning techniques that deliver exceptionally rich and nuanced audio.
Acoustic metamaterials offer a novel approach to sound manipulation, going beyond what conventional materials can achieve. Their unique structures can manipulate sound waves, including bending, absorbing, or redirecting them, which opens up exciting possibilities for more refined control in voice synthesis. For example, they could potentially lead to synthetic voices that not only accurately reproduce the phonetic aspects of a person's speech but also capture the subtleties of their emotional tone.
By carefully controlling the sound field, acoustic metamaterials can selectively amplify or attenuate specific frequencies. This capability is especially valuable in audiobook production where ensuring clarity and emotional expression is paramount. Furthermore, these materials allow for the creation of subwavelength structures, which can generate unique sound patterns not previously possible. This unlocks new avenues in voice cloning that could potentially lead to breakthroughs in replicating voices with greater accuracy and realism.
One of the key advantages of acoustic metamaterials is their ability to finely tune sound insulation to particular frequencies. This is a stark contrast to traditional soundproofing techniques, making them perfect for isolating a voice amidst noisy environments like podcast recording studios. Moreover, their ability to focus sound in specific directions suggests the potential for creating immersive and captivating spatial audio experiences. This could enhance storytelling in audio content by providing a sense of directionality and realism to the soundscape.
Recent research demonstrates the potential for integrating these materials into sound synthesis frameworks to simplify the computational demands of real-time voice manipulation. This advancement has huge implications for live performance technologies and could lead to smoother and more dynamic applications in the future. Furthermore, some researchers are exploring how to engineer these metamaterials to mirror the resonant characteristics of human vocal cords. This fascinating area of research could lead to the creation of more authentic and convincing synthetic voices.
We're also seeing exciting developments in the study of non-linear acoustic metamaterials. These materials offer the potential to manipulate harmonic distortions, leading to novel audio effects within voice-based applications. This exploration holds potential for the creation of new sound textures and the development of increasingly sophisticated sonic environments. This new field of research indicates that utilizing acoustic metamaterials doesn't just improve the quality of synthetic voices, but it also paves the way for more interactive experiences where a cloned voice could respond dynamically to its surroundings.
Let's Try Something Different Exploring Unconventional Voice Cloning Techniques in 2024 - Neural Network-Based Emotional Inflection Mapping
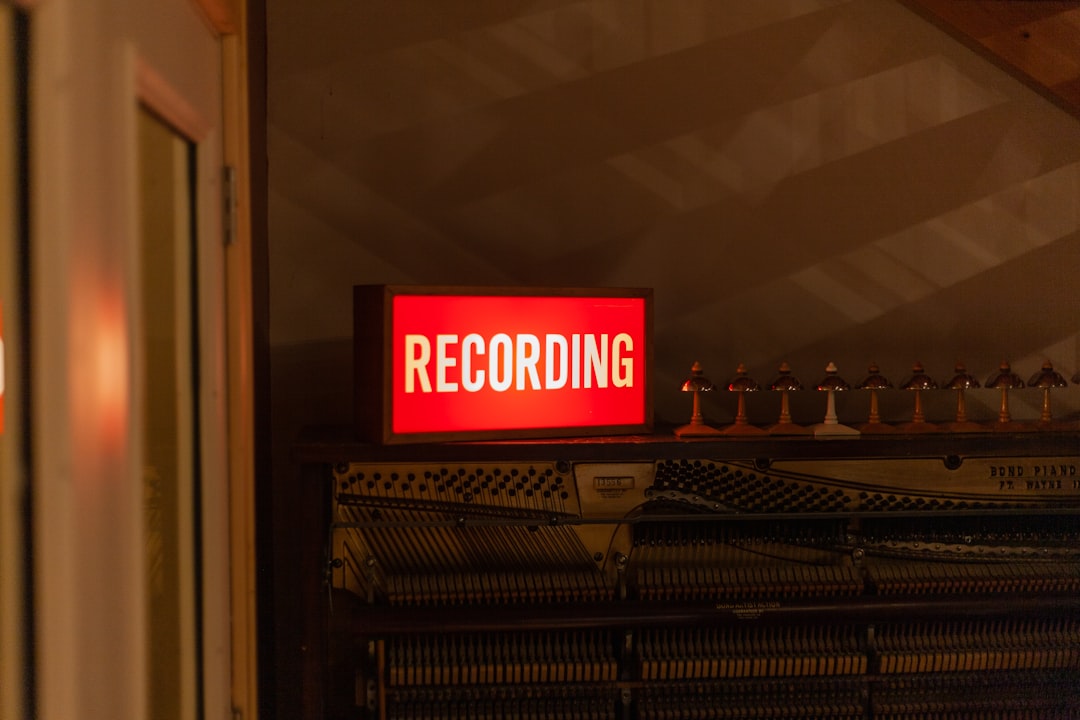
Neural Network-Based Emotional Inflection Mapping is a new approach to voice cloning and audio production, focused on making synthetic voices more emotionally expressive. This technique utilizes advanced neural networks to analyze and replicate the emotional nuances present in human speech. The goal is to create synthetic voices that are not only accurate in their pronunciation but also convey emotions authentically, enriching experiences in areas like audiobooks and podcasting.
By feeding large datasets of emotional speech into deep learning models, researchers are gaining a deeper understanding of how emotions manifest in vocal inflections. The hope is to develop cloned voices that are capable of expressing a wider range of emotions in a way that resonates more deeply with listeners. Integrating this technology into voice synthesis frameworks could lead to more dynamic and immersive audio experiences where cloned voices react in real-time, mimicking natural emotional expression.
While promising, there are challenges to overcome. Balancing technical accuracy with the inherent complexity and subtle nature of emotional communication in audio productions will require further advancements in both the algorithms and datasets used in this technology. It remains to be seen how well these AI-powered methods can truly capture the subtleties of human emotional expression in voice cloning.
Neural networks are increasingly being used to map emotional inflections in speech, allowing synthetic voices to convey a wider range of emotions. This could greatly enhance the experience of listening to audiobooks or podcasts, where conveying emotion is crucial. The networks learn from vast datasets of human speech, identifying patterns in tone, pitch, and rhythm that correlate with emotions like joy, sadness, or anger.
One exciting development is real-time emotional inflection processing, which opens up possibilities for live performances or interactive podcasts. The generated voice can dynamically adjust its emotional expression based on the context of the conversation, potentially creating a more engaging experience for the listener. Interestingly, this approach seems to have potential across different languages, meaning that voice cloning techniques could be adapted to various cultures, leading to more culturally resonant audio experiences.
The level of detail that neural networks are able to capture is fascinating. Even small shifts in pitch or tone can be linked to different emotions. This accuracy is vital for applications like audiobooks and voice acting where subtle emotional cues can bring characters to life in a way that simply mimicking speech patterns cannot.
It's worth considering the potential psychological effects of using these techniques. The ability to carefully craft emotional expressions in a synthetic voice could significantly influence the listener's mood and engagement. This could enhance storytelling in audio content, but it also raises questions about how this power should be used responsibly.
Recent advances have made training these neural networks more efficient. This means less data is needed to produce a convincingly emotional voice, resulting in shorter recording sessions and quicker project turnaround times for voice synthesis projects. Furthermore, combining this with computer vision could allow synthetic voices to react not only to spoken words but also to visual cues. This could pave the way for more authentic and interactive experiences within virtual environments or immersive audio stories.
However, despite progress, achieving perfect authenticity remains a challenge. Humans are quite good at detecting subtle differences between human and synthetic emotional expressions. There's still room for improvement in refining these mapping techniques to make the generated emotions even more convincing.
Beyond entertainment, this technology has the potential for use in therapeutic contexts. A comforting and empathetic synthetic voice could be used in virtual therapy settings, potentially offering support for mental health initiatives. This represents a rather remarkable application of voice synthesis.
Let's Try Something Different Exploring Unconventional Voice Cloning Techniques in 2024 - Quantum Computing Applications for Voice Fingerprinting
Quantum computing presents a new frontier for voice fingerprinting, a field that's crucial for applications like voice cloning. Quantum computers, operating on the principles of quantum mechanics, promise a significant leap in processing audio data and identifying individual vocal characteristics. This enhanced capability could potentially revolutionize how we approach voice cloning, making it even more accurate and refined.
One area of interest is quantum communications, which could dramatically improve the speed and efficiency of transmitting and analyzing audio data used in voice cloning. This could lead to better voice recognition and improved accuracy in replicating voices for audiobook production or podcasting. However, applying these quantum computing principles to voice fingerprinting presents a considerable technical hurdle. The inherent complexity and fragility of quantum systems make it challenging to seamlessly integrate these technologies into existing voice synthesis workflows.
Despite the challenges, the future potential of quantum computing in voice fingerprinting is significant. As the field matures, it could drastically change how we experience sound and personalized audio, leading to more natural and nuanced cloned voices. Whether or not the development of reliable and practical quantum-powered voice fingerprinting occurs within the anticipated timeframe remains to be seen. The ongoing research and development in this area are undoubtedly changing the game for auditory experiences, especially within the ever-expanding world of voice cloning.
Quantum computing's unique capabilities, like superposition and entanglement, might significantly enhance voice fingerprinting techniques. Imagine algorithms exploring multiple voice characteristics simultaneously, leading to much faster and more accurate voice identification. This speed increase could be a game-changer for fields like forensic audio analysis where rapid access to voice databases is crucial.
While classical computers handle voice data sequentially, quantum algorithms could drastically reduce the time needed for certain computations, like searching for unique voice signatures. However, it's important to remember that this is still largely theoretical, as quantum computers are not yet capable of handling complex real-world voice data sets with the needed reliability.
The concept of quantum entanglement, where linked particles share the same fate, could improve the security of voice data transmission. If voice fingerprints were intercepted, the inherent nature of quantum information could make it almost impossible to replicate without detection. This could prove immensely valuable in protecting private audio content and conversations.
Quantum annealers, specifically designed to tackle optimization problems, could help refine voice fingerprinting techniques. This enhanced optimization might focus on identifying very subtle voice characteristics, which could have a big impact on things like personalized audiobook narration, tailoring the audio experience to the listener's preferences.
Quantum computing could also allow researchers to simulate acoustic metamaterials in a way never before possible. This deep-level understanding of these materials could lead to more advanced designs that enhance voice clarity in noisy environments, opening the door to exciting new voice processing capabilities. It's important to emphasize that, in 2024, such research is very much in the exploratory stage.
Quantum computers excel at analyzing high-dimensional data, which is crucial for voice analysis due to the large number of overlapping attributes like pitch, tone, and frequency. This could lead to sophisticated algorithms that can distinguish between different voices, even in noisy environments.
Another promising area is the potential reduction in the amount of training data required for effective voice fingerprinting. This is beneficial when it's difficult to gather a wide variety of voice samples. Robust voice models could potentially be built using fewer examples, making these techniques more accessible.
The speed of quantum processing has the potential to enable real-time voice fingerprinting for live events like podcasts or interactive audio stories. Hosts could incorporate audience interactions more seamlessly and smoothly. However, achieving true real-time functionality on complex audio requires advancements in both hardware and software, making this still somewhat futuristic for 2024.
Quantum computation might also pave the way for more realistic synthetic voices in voice synthesis and cloning applications. By leveraging quantum processes, we might be able to preserve and replicate unique vocal traits of individuals more precisely. This would not only enhance voice cloning but potentially revolutionize audiobook narration, making synthetic narrators sound more like real people. However, this area is still in its early stages, and producing a voice that is indistinguishable from a human voice is a challenging task.
Finally, quantum machine learning could make the process of mapping emotional inflections in speech much more advanced and precise. Analyzing and processing complex datasets more effectively would enable synthetic voices to convey deeper emotional depth, potentially enhancing virtual storytelling and therapeutic applications. While the emotional capabilities of AI are constantly evolving, achieving a truly human-like range of emotion in a synthetic voice remains a major hurdle.
In summary, the application of quantum computing to voice fingerprinting presents a fascinating set of theoretical possibilities. However, the current limitations of quantum computing technology need to be considered. Nevertheless, the potential for improvement and innovation in this emerging field is undeniable.
Let's Try Something Different Exploring Unconventional Voice Cloning Techniques in 2024 - Bioacoustic Modeling for Hyper-Realistic Voice Cloning
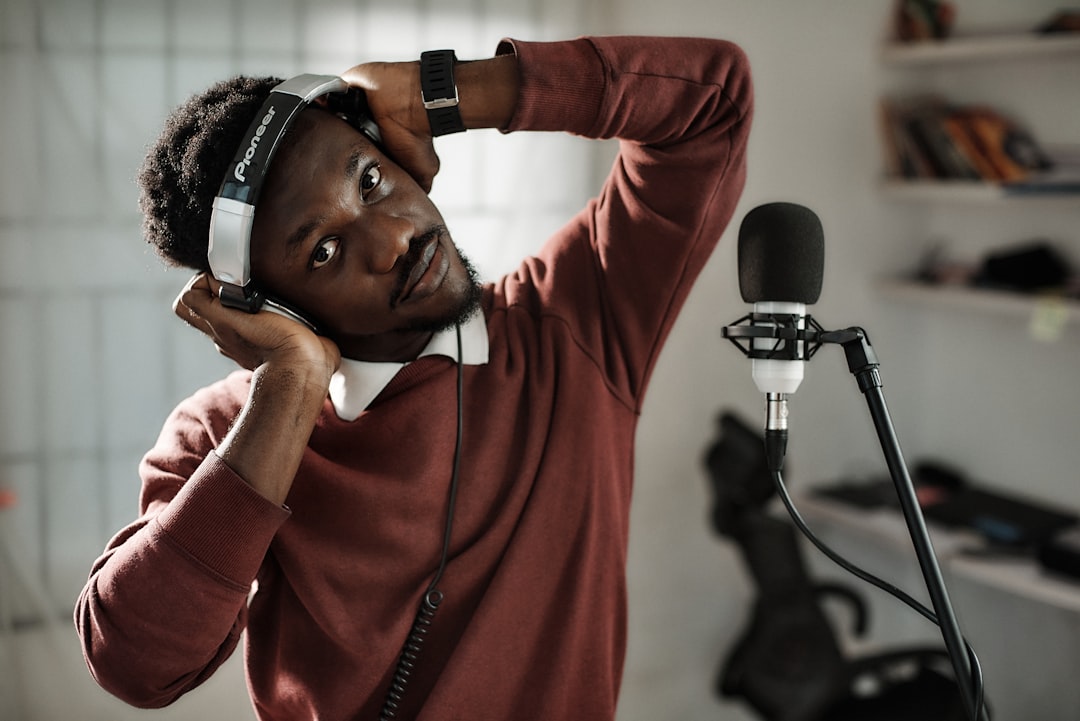
Bioacoustic modeling offers a novel approach to achieving hyper-realistic voice cloning, moving beyond conventional methods by focusing on the intricate sound production mechanisms of the human voice. This method employs sophisticated machine learning algorithms to extract and replicate the unique acoustic characteristics of a person's voice, including subtle variations in pitch, tone, and overall timbre, which are crucial for achieving truly lifelike voice synthesis. As the field of voice cloning progresses, the integration of bioacoustic insights has the potential to generate voices that not only mirror speech patterns but also capture the nuanced emotional depth that defines human communication. While this area of research is still developing, the potential implications for applications like audiobook production, podcasting, and voice-driven content creation are significant, possibly leading to more immersive and engaging listening experiences. However, significant hurdles remain in developing these models to perfectly replicate the complexity and emotional nuance present in authentic human speech. Balancing intricate technical details with the inherent emotional richness of human interaction is a key challenge for the future of bioacoustic voice cloning.
Bioacoustic modeling offers a fascinating approach to voice cloning by focusing on the intricate details of sound production. This technique leverages sophisticated algorithms to analyze the unique resonance and vibration patterns inherent in each individual's voice. By delving into the physical properties of sound generation within different vocal timbres, bioacoustic models strive for more personalized and accurate voice cloning, capturing nuances that might be overlooked by traditional methods.
Researchers are also drawing inspiration from the natural world, particularly animal vocalizations like bird songs and whale calls. Studying these bioacoustic behaviors provides valuable insights into how various sounds are produced and perceived, which can be incorporated into voice cloning to enhance realism. Understanding the underlying principles of sound production in nature can help improve the authenticity of synthetic voices.
A key aspect of bioacoustic modeling is harmonic analysis. This technique essentially dissects a voice into its fundamental frequencies and overtones, allowing for a detailed understanding of the voice's sonic structure. This detailed breakdown aids in the creation of voice clones that retain natural resonances and tonal qualities, leading to a more genuine auditory experience.
Interestingly, emotional states have a noticeable effect on vocal tonality. Bioacoustic modeling incorporates this aspect by analyzing the unique frequency patterns associated with emotions. By integrating this data, synthetic voices can potentially portray a wider range of emotions more authentically, enriching the listening experience in areas like audiobook narration and podcasting.
Beyond entertainment, bioacoustic models show promise in enhancing accessibility for individuals with speech impairments. By mapping specific vocal signatures, these models can generate synthetic voices that closely resemble a person's natural speaking style, fostering a stronger sense of personal expression and communication.
The advancements in bioacoustic modeling are enabling real-time adjustments of synthetic voices based on external audio inputs. For example, a voice clone could adapt its tone in response to background noise levels or even emotional cues detected in a conversation, making the clone more versatile and responsive across various audio contexts. This dynamic adaptability could be particularly useful for immersive audio experiences.
Furthermore, bioacoustic models go beyond simple replication; they incorporate a deeper understanding of phonetics and frequency characteristics specific to different languages and dialects. This nuanced understanding is crucial for creating voice clones that sound authentic across cultural boundaries, a feature that could be beneficial for global podcast production and distribution.
Bioacoustic modeling and artificial intelligence are working in tandem to produce increasingly sophisticated voice cloning techniques. AI excels at analyzing large datasets of voice recordings, while the bioacoustic principles ensure the cloned voice retains its fidelity and expressive qualities. This symbiotic relationship helps create more nuanced and human-like synthetic voices.
Beyond voice cloning, bioacoustic modeling can be applied to study voice fatigue in speakers, analyzing the strain placed on the vocal apparatus during extended periods of speech. Understanding the mechanics of vocal strain could potentially lead to the development of synthetic voices that mimic healthier speaking habits, promoting better audio production practices for audiobook narrators and broadcasters.
Finally, bioacoustic modeling facilitates the creation of multidimensional sound maps that represent voice characteristics spatially. This advanced technology can result in more immersive audio experiences. For instance, a podcast listener might perceive the speaker's location shifting within the soundscape based on the story content. This spatial awareness could significantly enhance listener engagement and immersion in audio-based storytelling.
While still a developing field, bioacoustic modeling presents a compelling set of tools that may lead to more refined and expressive voice cloning techniques in the future. The potential applications are extensive, ranging from improved entertainment experiences to greater accessibility for diverse audiences.
Let's Try Something Different Exploring Unconventional Voice Cloning Techniques in 2024 - Cross-Modal Voice Synthesis Using Visual Cues
Cross-modal voice synthesis, a relatively new approach in voice cloning, leverages visual cues alongside audio to generate more realistic and accurate synthetic voices. This method utilizes facial expressions and lip movements as additional information to guide the voice synthesis process, effectively linking visual and auditory components of speech. This interdisciplinary approach can enhance the quality of voice cloning by producing audio that better aligns with the speaker's visual cues, resulting in more natural and intelligible voices. For example, systems like VISUALVOICE can separate audio and visual components of speech even when dealing with multiple speakers and ambient noise.
While promising, this reliance on visual cues raises questions about the overall authenticity of synthesized voices. It's worth pondering if solely relying on visual information for voice creation can adequately capture the nuances and emotional depth inherent in human speech. The effectiveness of this approach in creating truly engaging audio experiences, particularly for applications like podcasts and audiobook narration, is still to be fully explored and assessed. Future developments will need to carefully weigh the advantages of this cross-modal approach against the need to maintain a sense of emotional depth and authenticity in the synthesized audio to create truly compelling synthetic voices.
Cross-modal voice synthesis, a fascinating area of research, aims to create more realistic synthetic voices by combining audio and visual cues. The core idea is that speech isn't just about the sounds we make, but also the accompanying facial expressions and lip movements. The VISUALVOICE framework, for example, demonstrates this by employing cross-modal consistency. It cleverly uses the relationship between lip movements and facial characteristics to improve the separation of speech from other audio, effectively learning to pick out a voice amidst noise and other speakers.
Interestingly, this method can also improve the quality of voice cloning by leveraging visual information to refine the audio generation process. By tying together visual and auditory data, researchers are getting closer to a more accurate representation of a person's voice, moving beyond simply recreating the phonetic components of speech. This dual approach of analyzing both what we hear and see while speaking has implications for enhancing speaker embedding, the way we digitally represent an individual's unique voice. It effectively utilizes the face as a guide for isolating and cleaning up audio, proving particularly helpful when dealing with recordings from various angles.
Beyond simply separating voices, researchers are also exploring how the physical link between voice production and facial expressions can be leveraged for improved voice synthesis. The idea is that understanding how a person's vocal tract movements translate into facial expressions can be a key to developing more explainable AI systems in voice-related applications, including biometrics. It's remarkable how this technique shows robustness across various real-world scenarios, suggesting the ability to translate to a wide array of use cases.
Moreover, this approach also shines a light on the importance of learning how voices and faces connect. This isn't just about mimicking someone's voice but about understanding the underlying correlations between these two communication channels. Machine learning approaches are being developed that involve both supervised and unsupervised learning, essentially aiming to teach machines the subtle relationship between how a person's face moves and the sounds they produce.
Ultimately, the ability to integrate both visual and auditory modalities allows for a more holistic representation of a speaker, leading to potentially better voice cloning. It highlights the possibility of enhancing the way we perceive and utilize synthesized voices. However, alongside this promise are important ethical considerations. While these techniques might improve the quality of audio content, the potential for manipulating a person's likeness using synthesized voice requires careful thought and responsible implementation. It's a field with great potential but also with challenges that warrant responsible exploration.
Let's Try Something Different Exploring Unconventional Voice Cloning Techniques in 2024 - Neuromorphic Hardware Acceleration in Voice Processing
Neuromorphic computing, inspired by the brain's structure, offers a new approach to voice processing, particularly relevant to voice cloning and synthetic audio generation. It diverges from conventional computer architectures, potentially leading to more efficient and faster processing of intricate audio information. This method hinges on specialized hardware components like artificial synapses and neurons, which significantly accelerate computations within artificial neural networks. This can be beneficial for creating voice cloning applications that react quickly and intelligently to different situations.
The field of neuromorphic engineering is continuously developing, hinting at future advancements in energy efficiency for AI-powered voice systems. Furthermore, it suggests that these systems might learn and adjust to changes in real-time. This capability is particularly advantageous in dynamic audio situations like live podcasting or interactive storytelling, where voices need to be responsive to varied emotional tones and environmental factors. However, incorporating neuromorphic systems seamlessly into current voice processing pipelines is still a challenge. It necessitates ongoing research to fully explore the potential for creating highly realistic and emotionally nuanced voice clones.
Neuromorphic hardware, inspired by the brain's structure, offers a novel approach to voice processing, potentially revolutionizing voice cloning, audiobook production, and podcasting. Unlike traditional computer architectures, neuromorphic systems use an event-driven approach, meaning they react only when there's a change in the audio input, instead of constantly cycling through data. This can dramatically lower power consumption, making it particularly attractive for portable devices where battery life is a concern, such as voice-controlled smartphones.
The ability of these systems to swiftly adapt to different sounds is intriguing. They can quickly learn from varied audio inputs, adjusting voice synthesis in real-time. This makes them ideally suited for scenarios where the cloned voice needs to respond naturally, such as in a virtual assistant or interactive audiobook. Moreover, their design allows them to be remarkably resilient to noise, which can be crucial in environments prone to disruptive sounds, like a busy podcast studio.
Another fascinating characteristic of neuromorphic hardware is its remarkable memory efficiency. By employing memory-saving data-handling methods, they can significantly reduce latency in tasks like voice recognition. This could mean smoother interactions in applications like virtual assistants or interactive audiobooks, as the systems can react quicker to user commands.
Neuromorphic hardware also paves the way for developing generative models that can autonomously improve synthesized voices. They can learn from a limited set of voice samples and create diverse and expressive speech, potentially reducing the need for massive training datasets. This method of processing voice data through spiking, akin to the activity in biological neurons, provides an incredibly precise encoding. This ensures the highest fidelity in voice transmission and reduces unnecessary data, boosting the accuracy of voice cloning efforts.
The inherent energy efficiency of these systems during learning is another attractive quality. This is crucial for voice cloning where ongoing user interactions are central to the process. Being able to learn continuously without massive energy consumption is a significant advancement. Additionally, their ability to capture fine-grained differences in audio signals, especially in the timing of those differences, enables them to potentially replicate the subtle nuances in tone and pitch that convey emotion. This opens exciting possibilities for making synthetic voices more expressive, particularly in audiobooks and podcasts.
The parallel processing abilities of neuromorphic hardware are another feather in its cap. Their architecture can manage multiple audio streams simultaneously, which is incredibly valuable when multiple voices need to be synthesized or recognized simultaneously, as in a group conversation. Moreover, their computational structure allows for real-time interactions, which is fundamental for applications like live podcasts and audiobooks. The capacity of the synthesized voice to instantly adapt to context or feedback ensures a more engaging listening experience for the audience.
However, it's important to acknowledge that neuromorphic computing is still a developing field. While the potential is undeniable, there are technical challenges to overcome before we see truly widespread adoption. Integrating neuromorphic hardware with existing voice synthesis workflows and refining the algorithms to achieve perfectly natural-sounding voices are just two of the obstacles that need to be tackled. Despite these hurdles, the future of neuromorphic hardware acceleration in voice processing looks very promising, and the possibilities for innovation are substantial.
Get amazing AI audio voiceovers made for long-form content such as podcasts, presentations and social media. (Get started for free)
More Posts from clonemyvoice.io: