How Voice Samples Length Affects AI Voice Cloning Quality A Data-Driven Analysis
How Voice Samples Length Affects AI Voice Cloning Quality A Data-Driven Analysis - Minimum Sample Duration The Science Behind Voice Training
The duration of a voice sample is a cornerstone in the science underpinning voice training and, by extension, the success of voice cloning. The length of the sample profoundly impacts the quality of AI-driven voice synthesis. Longer samples offer a more comprehensive acoustic landscape for analysis, leading to a more accurate representation of the individual's voice characteristics. In the realm of voice cloning, capturing the intricacies of a specific voice requires a sufficiently long audio segment to encompass the nuances and variations present in natural speech. While recent advancements in neural voice cloning strive to reduce the need for extensive sample lengths, the efficacy of these methods remains strongly linked to the quality and duration of the input audio. Ultimately, the judicious selection of sample length influences the fidelity of the cloned voice and the overall listening experience, ensuring the synthetic voice closely mirrors the original. Achieving optimal voice cloning relies not simply on sample quantity but on ensuring that the chosen duration provides enough information for the AI to effectively learn and replicate the desired vocal patterns.
Regarding the duration of audio samples used for voice training, a critical threshold seems to exist. Researchers are finding that around 30 seconds of audio provides enough phonetic variety and nuanced vocal characteristics to generate more accurate voice clones. Shorter samples, particularly those under a second, may struggle to capture the full range of vowel and consonant sounds needed. Each phoneme has specific acoustic features that require a minimum time frame, usually between 0.5 to 1 second, for proper representation.
Additionally, short audio clips can lead to misinterpretations during the voice synthesis process. Without sufficient context like intonation or emotional nuances, AI models might struggle to accurately understand the speaker's intended message, resulting in robotic-sounding speech. The quality of the surrounding environment also plays a role; shorter samples are more vulnerable to noise interference. In comparison, longer samples give AI models a better opportunity to identify and filter out background noise, resulting in cleaner and more natural-sounding synthetic voices.
Furthermore, we see that training data from longer samples helps maintain a consistent voice across various speaking styles and paces. This ability to generalize is valuable for voice cloning. Interestingly, the language being used can also influence the best sample duration. Tonal languages, for instance, may require longer samples to fully capture the subtleties of tonal shifts, while languages that rely more on stress may benefit from a variety of sample durations to accurately represent rhythm.
We've also observed that longer recordings are better at capturing emotional content within speech. These subtle emotional cues can be lost in short recordings. This is particularly important in applications like audiobooks and podcasts, where maintaining listener engagement depends on conveying emotion through the voice. AI models trained with longer samples also adapt better to various voice qualities and speech patterns, potentially resulting in more versatile voice outputs.
However, it's important to note that while longer samples generally offer improved outcomes, there can be diminishing returns if the data becomes excessively long. There's likely an optimal window that maximizes performance without being overly redundant. Finally, the temporal dynamics of speech, things like pauses and breathing, are crucial for natural-sounding speech, and these are captured more completely in longer recordings. This feature is particularly important for applications like storytelling and narrations where the human element of delivery is critical.
How Voice Samples Length Affects AI Voice Cloning Quality A Data-Driven Analysis - Audio Processing Techniques in Short vs Long Voice Recordings
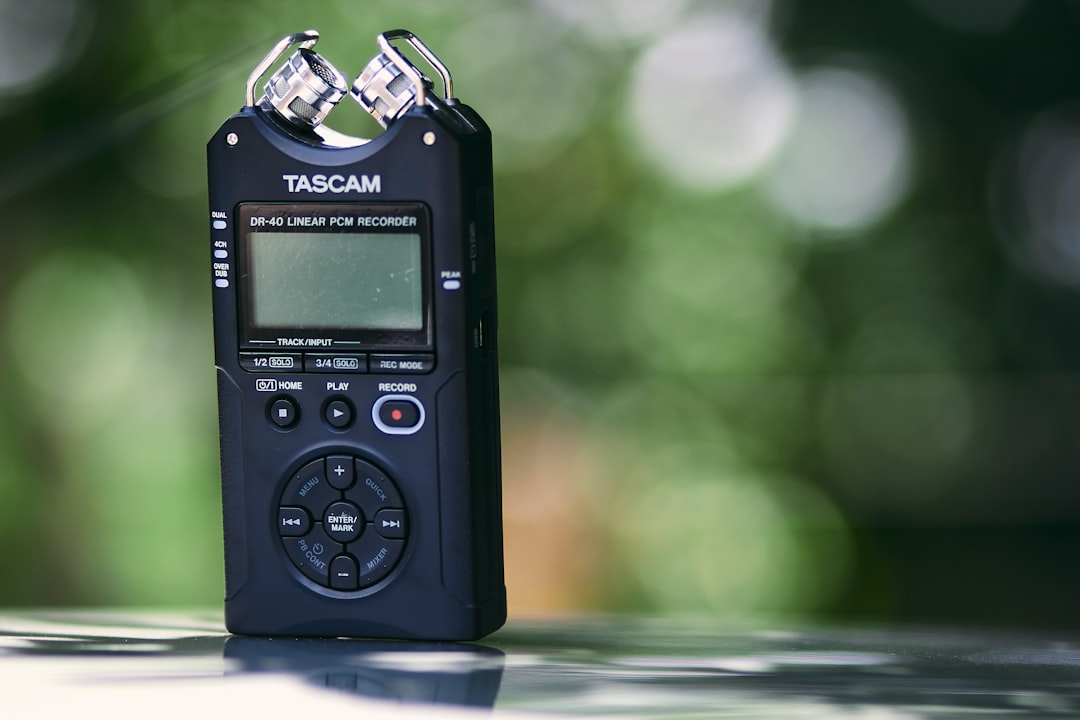
The way we process audio data significantly impacts the success of voice cloning, particularly when dealing with varying lengths of recordings. Shorter voice clips often pose difficulties for AI systems because they may lack the diverse phonetic information and contextual clues required for accurate speech synthesis. This can result in a less natural-sounding output, particularly in terms of capturing the speaker's emotional nuances and the subtle variations in their voice. Longer recordings, on the other hand, give AI algorithms a more robust dataset to work with. This abundance of data enables a more comprehensive understanding of the speaker's vocal characteristics, leading to improved cloning accuracy. Furthermore, longer samples provide a more stable platform for noise reduction, potentially leading to a cleaner and more desirable output. However, simply having longer recordings isn't always ideal. There can be a point of diminishing returns, and excessively long samples may add unnecessary data that does not improve the cloned voice quality. The balance between the chosen processing techniques and the length of the input voice sample is critical for attaining a high-fidelity synthetic voice. This is particularly crucial in areas like audiobook production and podcasting, where preserving emotional expression is a priority. The techniques used to process the sound, whether it's a simple podcast interview or a sophisticated audiobook narrative, are vital in achieving a convincing and engaging AI voice.
When it comes to voice cloning, the length of the audio sample plays a pivotal role in the quality of the synthesized voice. Longer recordings generally offer a wider range of sounds, which is crucial for accurately replicating the nuances of a speaker's voice. This is particularly true for capturing subtle variations in phonemes, leading to a more natural-sounding clone.
Shorter recordings, unfortunately, are more prone to being tainted by background noise. This noise can interfere with the clarity of the synthetic voice. However, with longer recordings, algorithms designed for noise reduction have a larger window of opportunity to effectively filter out extraneous sounds, improving the output voice's overall cleanliness.
The way we speak, with its distinct rhythms, pauses, and changes in tone, is better captured by longer recordings. These timing aspects, or temporal dynamics, are vital for creating a voice that sounds natural and engaging. For applications that rely on an authentic delivery, such as podcasts and audiobooks, these aspects are especially significant.
Based on research, it seems a minimum of roughly 30 seconds of audio data provides the optimal starting point for effectively cloning a voice. This duration encompasses enough phonetic variation and nuanced vocal characteristics to create a reliable model. Shorter samples can sometimes struggle to capture the full range of sounds needed.
The emotional aspects present in speech are better preserved in longer samples. These subtle emotional cues, which enhance listener engagement, are easily lost in brief clips. Applications like storytelling and podcasting, where emotion can be crucial for audience connection, greatly benefit from this advantage.
Furthermore, AI models trained on longer audio samples demonstrate an improved ability to understand the context of the speech. Sarcasm, emphasis, and other contextual subtleties are more readily interpreted, avoiding the mechanical-sounding output common with short recordings.
While longer samples are beneficial, extending the recordings indefinitely isn't necessarily a recipe for success. Overly long recordings can introduce unnecessary redundancies that can slow down training and hinder efficiency. Striking a balance is key.
Interestingly, the language itself can influence the best sample length for cloning. Languages with a heavy reliance on tone might require longer samples to accurately reproduce those shifts. Conversely, languages that rely more on stress might perform adequately with a wider range of sample durations.
The versatility of a cloned voice is significantly improved when training is done with longer audio recordings. This is because AI models can better learn to generalize across various speaking styles, ranging from casual to formal, with appropriate emotional nuances, enhancing the overall usability of the synthetic voice.
Ultimately, the delicate interplay between the timing of speech and the ability of a model to synthesize natural-sounding voice is heavily reliant on the length of the voice samples used. By using longer recordings, we unlock opportunities for creating truly authentic, natural-sounding voices, which is essential in a wide range of applications, from voice assistants to interactive narratives.
How Voice Samples Length Affects AI Voice Cloning Quality A Data-Driven Analysis - Studio Recording Requirements for Clean Voice Samples
For achieving optimal results in AI voice cloning, especially in applications like audiobook production and podcasting, the quality of the voice samples is paramount. Studio recordings are ideal as they allow for control over the environment and equipment, ultimately leading to cleaner audio. High-quality microphones and audio interfaces are essential tools to capture the voice with precision and eliminate unwanted noise. It's imperative that the recordings feature a single, clear speaker without any distracting background noise. This ensures that the AI model focuses solely on learning the unique characteristics of the target voice, creating a more accurate and realistic clone.
Moreover, post-recording processing is crucial. Techniques like equalization (EQ) to adjust the frequency balance and noise reduction through techniques like noise gating can refine the raw recordings and enhance their clarity. These steps are important for optimizing the sample for voice cloning algorithms. While some voice cloning methods can produce decent results even with less-than-perfect recordings, the competitive landscape favors meticulously prepared, clean audio samples. These meticulously crafted samples lead to more accurate and natural-sounding cloned voices, making them a key factor in successful voice cloning projects. The impact of these pre-processing steps, even simple ones, is evident when comparing the difference in quality between recordings made with minimal effort and studio-quality productions.
To obtain clean voice samples suitable for AI voice cloning, several factors in the recording process are critical. The choice of microphone significantly impacts the final outcome. Condenser microphones, known for their sensitivity, are often favored over dynamic microphones because they capture a broader range of frequencies, thus preserving subtle vocal nuances that are crucial for accurate voice representation. However, the environment's acoustics are just as important. A room with treated surfaces to minimize reflections and echoes creates a cleaner recording with less unwanted noise, making the AI's job easier.
The length of the recording also affects the processing requirements. Shorter recordings can be challenging for AI as they may lack the phonetic variety and context longer samples naturally provide. This means more intensive processing is sometimes necessary for shorter samples, which can potentially lead to a less natural-sounding clone. Higher sample rates and bit depths during recording capture more detail and allow for a richer voice representation, leading to a more refined cloned voice.
Dynamic range compression can be a useful tool during recording. It helps manage the variations in loudness, ensuring that all parts of the vocal range are well-represented without being overly dynamic for the AI model. Furthermore, maintaining a consistent distance between the speaker and microphone reduces variability in the bass response (the proximity effect), ensuring the character of the voice is stable. This consistency is critical for creating an accurate voice clone.
Background noise poses a greater threat to shorter samples. Directional microphones or recording in noise-isolated spaces are essential for minimizing this problem. The management of breath sounds during recording is another aspect to consider. While capturing some breath can add authenticity, excessive amounts can necessitate extra editing effort.
It's also essential to consider the speaker's physical condition. Extended recording sessions can lead to vocal fatigue, affecting the consistency of the sample. Scheduling breaks and paying attention to the speaker's well-being can significantly improve vocal quality and consistency. The selection of the script is equally important for ensuring a wide variety of phonetic sounds and speaking patterns are represented. The richer and more diverse the script, the better the AI model will learn from the samples and improve the clone's quality.
In conclusion, it's a collaborative process of recording techniques, environmental control, and thoughtful recording practices that leads to the ideal voice samples for voice cloning. Attention to detail in the recording process, such as microphone selection, room acoustics, sample rate, and even the scripting, leads to significantly better results for AI models seeking to clone a voice. While some technologies can adapt to less than optimal audio, attention to detail results in better cloned voices overall.
How Voice Samples Length Affects AI Voice Cloning Quality A Data-Driven Analysis - Background Noise Impact on Voice Cloning Results
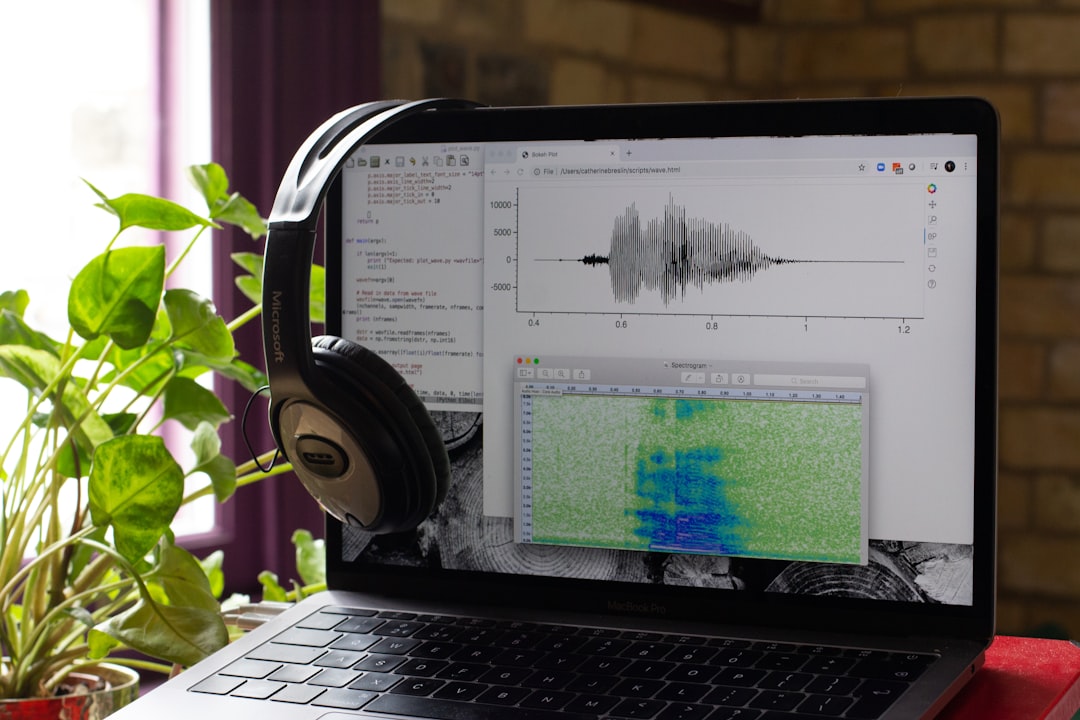
**Background Noise Impact on Voice Cloning Results**
The quality of voice cloning heavily depends on the clarity of the training data. Unfortunately, recordings often happen in environments with background noise, which can significantly impact the AI's ability to accurately capture and reproduce a person's voice. Noise, whether it's traffic, machinery, or even a room's ambient sounds, muddies the audio signal, making it difficult for the AI to isolate the specific vocal characteristics it needs to learn. This interference can lead to synthetic voices that sound artificial, lacking the natural nuances and emotional expressiveness that are vital in applications like audiobooks or podcasting.
To combat the negative effects of noise, AI researchers are developing more sophisticated noise reduction methods. However, even with these improvements, the best approach is to record the initial voice samples in quiet, controlled environments whenever possible. Ultimately, the challenge remains to develop innovative voice cloning techniques that can produce high-quality synthetic speech even from audio recordings made in less-than-ideal, noisy conditions. This ongoing need for research and development emphasizes the importance of having clean recordings to achieve truly believable and engaging cloned voices.
The presence of background noise during voice recordings can significantly impact the outcome of AI voice cloning. Noise can obscure crucial voice characteristics, making it harder for AI models to accurately capture the speaker's unique vocal qualities. This often leads to synthesized voices that sound less authentic and more robotic, hindering the overall quality of the cloned voice.
For applications that depend on conveying emotional nuances, like audiobooks, the impact of noise becomes even more critical. Longer recordings offer AI models a better understanding of the context in which words are spoken, allowing them to pick up on subtle emotional inflections and vocal dynamics that might be lost in shorter, noisier clips. Essentially, the temporal features inherent in human speech, including pauses and the natural flow of breath, are better captured in longer recordings. This allows cloning algorithms to create more natural-sounding synthetic speech patterns, which are important for a seamless listening experience.
When training AI models with clean voice samples, they exhibit increased adaptability to diverse speaking styles. This means the cloned voice can be used more effectively in various situations, from formal presentations to informal conversations. Conversely, background noise can limit the range of phonetic information available in shorter recordings, potentially missing subtle sounds like breathy phonemes or sibilance. This can have a negative effect on the resulting clone, making it difficult to achieve a high level of fidelity.
Advanced noise reduction techniques are more effective when applied to longer recordings. This is because algorithms have a wider scope of audio data to work with, allowing them to better differentiate between the speaker's voice and the noise itself. However, extended recording sessions, particularly those in environments with fluctuating noise levels, can introduce variations in tone and energy due to voice fatigue. This inconsistency can lead to a less reliable AI model that might not accurately represent the original speaker's voice.
Background noise can introduce inconsistencies in the perceived vocal attributes of a speaker in short audio samples. To maintain a high degree of accuracy in voice cloning, a consistent recording environment is necessary. Noise can also impact the frequency response of a recorded voice. For example, low-frequency rumble, common in certain environments, might mask higher-frequency elements vital for speech nuances, ultimately affecting the naturalness of the clone. The script itself, during recording, can play a role in mitigating the noise's influence. A diverse script that encompasses a variety of phonetic contexts can help capture essential sounds even in noisy conditions, which is beneficial for training the AI model and, ultimately, improving the final cloned voice's quality. It's interesting to consider that even with advanced noise reduction methods, having cleaner voice recordings from the outset is vital for achieving the best possible results in AI voice cloning.
How Voice Samples Length Affects AI Voice Cloning Quality A Data-Driven Analysis - Emotional Range Detection in Voice Sample Length
The ability of AI to recognize emotional nuances within a voice sample is fundamental to the success of voice cloning. Especially in scenarios like audiobook production or podcasting, where the emotional tone of the narrator can significantly impact listener engagement, capturing a wide range of emotional expression is critical. However, the methods currently used to collect voice data often fall short of effectively capturing the full spectrum of human emotion. This limitation is especially pronounced in shorter audio clips, where subtle emotional cues can easily get lost without a sufficient amount of contextual information. Because of this, the quality of AI voice cloning models is restricted. The importance of properly incorporating diverse emotional content into training data, specifically through the careful selection of longer voice samples, is paramount. Moving forward, improving how AI detects emotional range in voice samples will undoubtedly lead to a significant increase in the naturalness and accuracy of voice clones across various applications.
The length of a voice sample significantly influences how well AI can capture and replicate the nuances of a speaker's voice, particularly in areas like emotional expression and natural speech patterns. Longer samples are generally preferred because they contain a richer array of acoustic features and contextual information.
For instance, capturing a wider range of emotions in a voice sample – like excitement, sadness, or surprise – is easier with longer recordings. These emotional cues, which are tied to how we change our tone and pace, help AI understand the speaker's emotional landscape. This knowledge is then used to create more expressive and engaging synthetic voices, which is beneficial for applications like audiobook production where listeners appreciate a narrator who can express a character's emotional journey.
Furthermore, the intricate timing of speech — like the use of pauses and how a person naturally breathes during conversation — are crucial for a natural-sounding voice. Unfortunately, these temporal aspects are often lost in shorter recordings, leading to AI-generated voices that sound a little stilted or robotic. Longer recordings, however, give AI more opportunities to learn these rhythms, creating a much smoother and more natural conversational feel.
Another benefit of longer voice samples is the improved ability to capture the diversity of phonemes. The broader range of sounds captured in longer samples allows AI to effectively model how people speak, leading to improved accuracy in replicating pronunciation and various speaking styles. This is particularly important when attempting to replicate a speaker's distinct accent or speaking patterns.
When considering speaker consistency, longer samples allow AI to capture more examples of a speaker's voice under varying conditions. This is beneficial for tasks like audiobook narration, where a consistent vocal quality across a wide range of scenarios (i.e., scenes of excitement, sadness, or even suspense) is needed to create an immersive experience for listeners.
Beyond phonetics and emotion, longer samples offer a richer context for AI models. It's amazing how well AI can pick up on subtle cues, such as sarcasm or emphasis, when trained on a longer duration of voice data. This enhanced contextual understanding improves the ability to generate voices that sound less robotic and more like genuine human speech.
Moreover, longer voice samples are invaluable when it comes to noise reduction. AI algorithms can more effectively isolate and remove background noise from a longer sample, resulting in a cleaner final voice. This is especially useful in scenarios where the recording environment was less than ideal.
However, it's worth considering that each phoneme has an inherent duration needed for correct recognition. Longer samples give AI enough time to capture these crucial phonetic elements, minimizing potential errors that can arise from too-short samples.
Further, the dynamic range – the variations in loudness within a recording – is another area where longer samples prove advantageous. The extended length enables better control over dynamic range compression during training, ensuring that variations in loudness are handled effectively without losing subtle details in the voice.
Ultimately, the choice of script for a voice sample has a notable effect on the effectiveness of voice cloning. Longer samples with varied scripts allow for a greater range of phonetic and emotional expressions to be captured, offering a more robust dataset for the AI.
While 30 seconds appears to be a good starting point for capturing adequate data, the optimal duration for different applications is an area of ongoing exploration. There's likely a sweet spot that captures enough information without redundancy. Researchers are constantly seeking that balance to optimize efficiency and effectiveness in voice cloning techniques.
How Voice Samples Length Affects AI Voice Cloning Quality A Data-Driven Analysis - Multiple Language Support Based on Sample Duration
The ability of AI to clone voices across multiple languages is significantly impacted by the length of the audio samples used for training. Newer voice cloning techniques demonstrate a remarkable ability to create high-quality synthetic voices using very short samples, in some cases, as short as 6 seconds. This capability has led to a surge in tools that support over a dozen languages. Such a development opens up possibilities for producing multilingual content like audiobooks and podcasts, where authentic emotional conveyance and the unique tonal qualities of the original speaker's voice are crucial. However, shorter samples can inherently lack the detailed range of emotional expression and acoustic variation that longer recordings capture. There is a trade-off to be aware of; shorter samples may be more convenient, but the AI might not be able to replicate the original voice with the same level of accuracy or versatility, which is particularly noticeable in applications demanding emotional depth and linguistic diversity. Ultimately, a proper balance between sample length and the desired output quality is vital for successfully using AI to produce believable and engaging multilingual voice cloning outputs. The ability to achieve this balance and effectively mitigate the potential drawbacks of using short samples is a crucial aspect of AI voice cloning research.
The duration of a voice sample significantly influences how effectively AI voice cloning can capture the nuances of a speaker's voice, especially when considering different languages. For example, tonal languages like Mandarin require longer recordings to properly capture the subtle shifts in pitch that are essential to the language. In contrast, stress-based languages like English might produce satisfactory results with shorter audio snippets.
Each individual sound in a language, or phoneme, has a minimum duration needed for accurate recognition. Research suggests that certain phonemes need at least half a second to be reliably identified. This underscores the crucial role sample length plays in achieving a high-fidelity synthetic voice.
Longer voice samples give AI models a better understanding of the speaker's timing. This includes pauses, rhythm, and even natural breathing patterns, all crucial for sounding human. Shorter audio clips tend to miss these temporal nuances, leading to more robotic-sounding synthetic speech.
Furthermore, emotional context is more apparent in longer recordings. AI models trained on such samples are better able to decipher nuances like sarcasm and emphasis, which significantly impact the listener's experience in applications like podcasts and audiobooks.
Longer samples also allow for more robust noise reduction. AI algorithms can more effectively separate the speaker's voice from background noise when provided with a larger audio context. This capability results in a clearer, more natural-sounding cloned voice, which is essential for a high-quality listening experience.
The dynamic range, or the variation in loudness during a recording, can be more effectively managed with longer samples. AI can learn to handle changes in volume more naturally when there's a wider range of audio to analyze, resulting in less mechanical-sounding cloned voices.
Maintaining consistency in a cloned voice is easier when trained on longer samples. AI models can capture consistent vocal characteristics across various emotional states, making it simpler to maintain quality during diverse types of narration or voice acting.
Similarly, emotional detection algorithms tend to perform better with longer recordings. A broader range of emotional expression allows the AI to learn and replicate a more diverse emotional palette, which is critical for creating believable characters in narrative applications.
Finding a sweet spot in terms of sample duration is also important. Excessively long samples lead to redundant data, which slows down training without a significant gain in accuracy. Striking a balance optimizes data processing efficiency for voice cloning.
Finally, the diversity of the recording script plays a vital role. A more complex script with varied vocabulary leads to a richer dataset. This allows the AI to learn a wider array of phonetic and emotional expressions, enhancing the overall effectiveness of the voice cloning process. While 30 seconds seems like a good starting point for capturing sufficient voice data, the optimal duration is still an area of ongoing exploration. Researchers continue to strive to find the ideal balance between the amount of data and the quality of cloned voices.
More Posts from clonemyvoice.io: