Get amazing AI audio voiceovers made for long-form content such as podcasts, presentations and social media. (Get started for free)
How Voice Sample Length Affects AI Cloning Accuracy A Technical Analysis
How Voice Sample Length Affects AI Cloning Accuracy A Technical Analysis - Impact of Background Noise On Voice Sample Quality
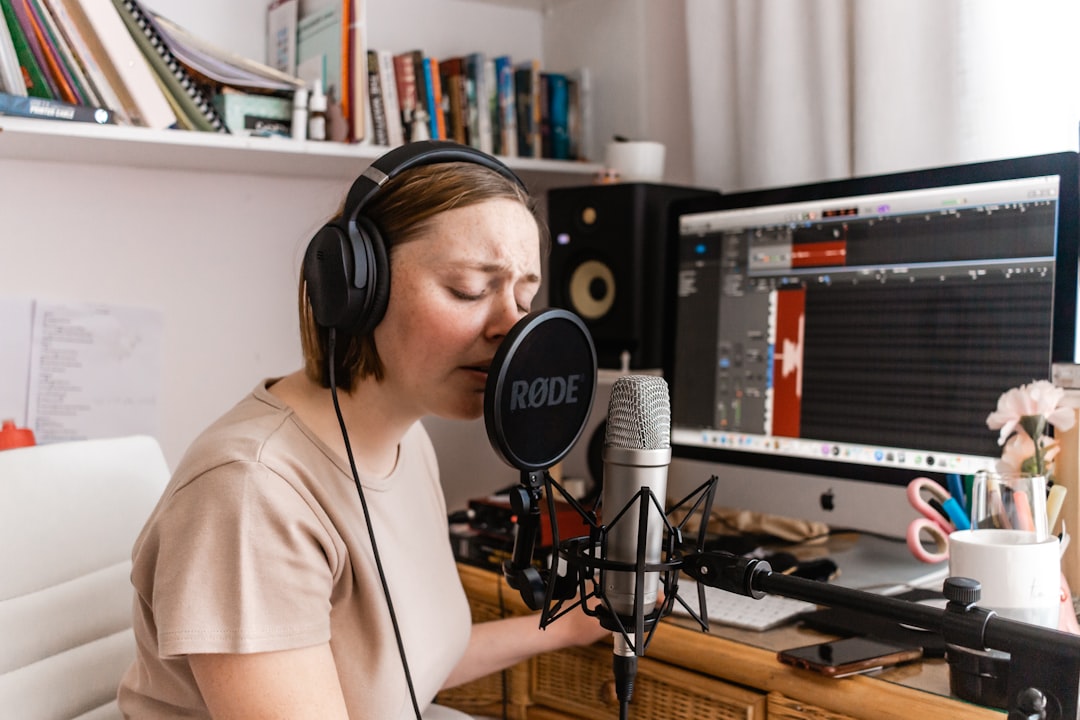
The presence of background noise can significantly compromise the quality of voice samples, impacting their clarity and making them harder to understand. This is a particular concern for applications reliant on accurate voice analysis, like voice cloning for AI models or producing high-quality audio books and podcasts. Extraneous sounds can mask subtle changes in pitch and tone, making it challenging for techniques like the Acoustic Voice Quality Index (AVQI) to accurately assess the vocal characteristics. While techniques like Variational Autoencoders are showing promise in reducing the impact of noise, the subjective nature of human perception adds complexity. The perceived quality of a voice sample can vary depending on individual listeners, the recording environment, and the overall structure of the recording itself. As a result, obtaining pristine audio recordings free from unwanted ambient sounds is paramount for achieving accurate voice replication in applications like AI voice cloning. The ability to minimize background noise during the recording process is thus crucial for enhancing the quality of the source material and optimizing the performance of voice cloning algorithms.
1. **Noise's Frequency Interference**: Background noise encompasses a wide range of frequencies, potentially overlapping with the core frequencies of the voice itself. This overlap creates distortion, obscuring subtle vocal details and making it tough for voice cloning algorithms to accurately capture the speaker's unique voice characteristics.
2. **Audio Compression's Impact**: In audio production, background noise often necessitates the use of dynamic range compression. While this can reduce the noise, it unfortunately flattens the audio signal. This flattening can lead to less expressive voice samples, making it harder for the voice cloning model to replicate emotional nuances and subtle shifts in tone.
3. **Signal Strength vs. Noise**: The signal-to-noise ratio (SNR) is a crucial factor in voice sample quality. If the SNR falls below 20 dB, the performance of voice recognition and cloning technology can plummet significantly. The models may struggle to distinguish the desired voice from the background noise.
4. **The Masking Effect of Louder Sounds**: Human hearing is affected by a phenomenon called frequency masking, where louder sounds can effectively mask quieter ones. This means that essential components of a voice sample can be obscured by background noise, severely affecting the quality of data used to train voice cloning algorithms.
5. **Counterintuitive Noise Addition**: Interestingly, some researchers have begun to use the opposite approach: artificially adding tailored noise that mimics various environments to voice samples during training. The idea is that this helps the algorithms develop a better understanding of noise patterns, so they become more capable of filtering it out in the real world, potentially leading to more accurate voice clones.
6. **Room Acoustics Matter**: The acoustic properties of the recording space heavily influence the quality of the voice sample. Hard surfaces in a room can cause reverberation that interacts negatively with background noise, leading to echoes and a muddy sound. Isolating the voice from these complications is harder during the processing phase.
7. **Training for Noise**: Voice cloning algorithms frequently incorporate conditioning techniques that involve adding environmental background noise to the training data. This aims to make the algorithms more resilient to various recording conditions encountered in the real world. However, it can also introduce potential challenges in maintaining the purity of the voice sample during the cloning process.
8. **Sampling Rate Effects**: The choice of sampling rate can significantly impact the way background noise affects voice samples. Lower sampling rates might not capture crucial high-frequency elements of speech, which are often the first targets of interference from background noise.
9. **Phoneme Problems**: Research suggests that background noise can have a disproportionate effect on the accurate recognition of certain sounds, particularly fricatives and plosives. These are the sounds that involve air rushing out, like 'f' or 'p'. This issue makes it harder for the cloning process to accurately model those sounds. It underscores the importance of high-quality, noise-free samples for achieving a precise phonetic representation.
10. **Time-Based Masking**: Noise isn't just a frequency issue. It also affects us over time, which is called temporal masking. Sounds that follow a louder noise can be harder to perceive. This can lead to incomplete voice samples lacking crucial temporal details, which are essential for producing high-fidelity voice clones.
How Voice Sample Length Affects AI Cloning Accuracy A Technical Analysis - Audio Frequency Range Analysis In Voice Copying
The standard microphone utilized in voice cloning applications typically captures a frequency range spanning from 20 Hz to 20,000 Hz, with a relatively flat response across the frequencies most relevant to human speech. This microphone's dynamic range is about 114 dB, capable of handling sound pressure levels as high as 120 dB, roughly equivalent to the noise produced by a chainsaw.
The goal of voice cloning is to produce artificial speech that closely mimics the voice of a specific individual. Current techniques are being honed to enhance the quality of the synthetic voice, particularly when working with lower quality datasets.
The duration of a voice sample impacts how the voice is perceived and the acoustic analysis results. Some studies have shown that voice samples as brief as three seconds are sufficient for making a rough determination of vocal characteristics and for estimating how accurate a clone will be.
The effect of voice sample length on the precision of AI voice cloning is a critical area of ongoing research. It's evident that determining the optimal duration for recording a sample is vital for achieving high-fidelity results.
Real-time voice cloning technologies rely on the power of deep learning to extract detailed acoustic data from human speech. This extracted data is combined with a text-based input to produce a natural-sounding synthetic voice.
With the growth of crowd-sourced audio data, we're now able to collect speech data in a variety of environments. The implications for voice analysis research are significant since these recordings reflect individuals speaking in their normal surroundings.
Voice cloning has advanced with the development of 'few-shot' generative models. These models enable us to clone a speaker's voice using only a few audio recordings, taking advantage of cutting-edge deep learning methods.
The rapid improvement of speech synthesis techniques is having a direct impact on the quality and accuracy of synthetic voice generation.
The rise of smartphones and wearable technology makes it possible to collect voice data on a massive scale. This presents exciting opportunities for conducting more in-depth analyses of vocal characteristics and for gaining a better understanding of how human voices work.
How Voice Sample Length Affects AI Cloning Accuracy A Technical Analysis - Voice Sample Consistency Testing Across Multiple Takes
Voice sample consistency testing across multiple takes is a critical aspect of voice cloning, particularly when aiming for high fidelity and accuracy. Human voices naturally vary across different recordings, and these variations can be quite impactful for AI models trying to replicate them. For instance, a person's voice might sound slightly different due to fatigue, a change in mood, or simply because of subtle alterations in their vocal production. These differences can introduce inconsistencies that challenge the ability of voice cloning systems to generate accurate and seamless replicas.
One of the most noticeable areas of inconsistency is in how a person articulates words. The same phrase recorded multiple times might have slightly different pronunciations, emphasis, and even vocal tone. These seemingly small details can lead to difficulties for AI models, potentially resulting in cloned voices that sound slightly unnatural or struggle to maintain perfect intelligibility. Additionally, factors like cognitive load on the speaker can impact pitch, tempo, and even emotional inflections, adding another layer of complexity to the consistency issue.
Microphone positioning plays a significant role in how the voice is captured. Even slight changes in distance or angle can drastically affect the tonal quality of the recording. This means ensuring consistently optimal microphone placement is essential for generating consistent voice samples – something that might be overlooked in some voice cloning experiments and audio recording scenarios.
Phonemes themselves are susceptible to changes across recordings, too. A phoneme's characteristics can change if a speaker's pitch or tempo shifts slightly. Since phonemes are the basic building blocks of words and sentences, inconsistency in their representation can lead to distortions in the cloned voice's pronunciation or even identity. This highlights the need for rigorous attention to detail when working with voice samples for cloning purposes.
Beyond phonetic variations, the temporal aspects of speech—like the rhythm, pace, and pauses—can shift as well. This leads to variations in the timing information extracted from the samples, which can affect how the cloning algorithm synthesizes speech. In essence, a model trained on inconsistent samples might struggle to faithfully replicate the natural flow and pacing of the original voice.
Interestingly, even how listeners perceive subtle variations in voice quality plays a role. Because our experiences and perceptions of speech are subjective, what might seem like an insignificant variation to one person could be noticeable to another. This underscores the complexity of achieving a voice clone that consistently meets a listener's expectations, as it's influenced by both technical accuracy and individual interpretation.
Harmonic structures, the foundation of a voice's timbre, can also be affected by inconsistencies between recordings. Changes in these harmonic structures can hinder the AI model's ability to accurately reproduce the original speaker's frequency characteristics. This can manifest as undesirable sonic artifacts in the cloned speech, impacting its naturalness.
Another factor is the role of vocal warm-ups. Individuals who use their voice frequently might perform different warm-up routines before recording sessions, and this can result in differences in their voice's consistency across samples. The impact of these practices underscores the need for standardized warm-up protocols or the recording of a warm-up period to build a better profile of the individual's voice across states.
Furthermore, the technology used during recording can affect how voice samples are captured. Different recording devices, microphone settings, or audio processing techniques might capture distinct aspects of a voice, introducing inconsistencies between different takes. Consequently, standardizing recording equipment and procedures across multiple takes becomes important for optimization and accurate replication of the target voice.
Finally, it's crucial to recognize that ongoing research in this field is striving to improve the robustness and accuracy of voice cloning techniques. This includes developing sophisticated methods to account for inherent variations in vocal performance and the effects of recording conditions. Future innovations are likely to address these challenges, leading to even more natural and high-fidelity voice clones.
Get amazing AI audio voiceovers made for long-form content such as podcasts, presentations and social media. (Get started for free)
More Posts from clonemyvoice.io: