How Voice Data Analytics Transforms Audio Book Quality 7 Key Metrics to Track
How Voice Data Analytics Transforms Audio Book Quality 7 Key Metrics to Track - Audio Clarity Measurement Through Dynamic Range Analysis in 2024 Production Standards
The year 2024 has seen a surge in the importance of dynamic range analysis for gauging audio clarity, a cornerstone of contemporary production standards. Dynamic range, essentially the difference between the quietest and loudest parts of an audio signal, is crucial for enhancing the perceived clarity and intricate details within audio content. This has become especially relevant in audiobook production where voice data analytics are increasingly used to precisely measure dynamic range. This, in turn, allows producers to fine-tune audio quality and guarantee adherence to industry norms.
Key metrics like signal-to-noise ratio and maximum operating level provide audio engineers with the tools they need to optimize sound and create audio experiences that truly immerse the listener. This renewed focus on dynamic range reflects a wider movement towards superior audio fidelity across the board, as professionals strive to continually improve the listening experience for audiences. However, it's worth noting that the pursuit of higher dynamic range in itself can sometimes be detrimental if not carefully managed, potentially leading to unnatural or jarring shifts in volume. A nuanced approach is needed to maximize its benefits while avoiding any adverse consequences.
In the evolving landscape of audio production, particularly within the audiobook and podcast spheres, the concept of dynamic range has taken center stage. It represents the gap between the softest and loudest parts of an audio signal, measured in decibels (dB). A wider dynamic range, intuitively, provides a more nuanced and detailed sonic landscape, which can translate to a richer listening experience. This is critical for mediums like audiobooks, where clear and engaging audio is paramount.
We’re seeing a subtle but significant shift in the audio industry. Historically, maximizing loudness was often the primary goal. Now, there's a growing appreciation for a balanced approach that prioritizes clarity over sheer volume, especially in spoken-word content. This has led to a shift in professional audio standards, reflected in the increased emphasis on a slightly wider dynamic range.
Furthermore, the increasing availability of A/B testing tools for audio is revolutionizing how engineers refine the dynamic range in their recordings. It enables them to experiment with different dynamic range settings in real-time, allowing for more subtle, informed adjustments. The result can be a more engaging listening experience, where the audio supports and enhances the storytelling aspect of the audiobook.
However, the quest for greater loudness hasn’t always been beneficial. Certain compression techniques can, ironically, negatively impact clarity, especially when applied too aggressively to spoken words. This creates a delicate balancing act for producers, who must carefully consider the trade-offs between perceived loudness and the intelligibility of the audio. There's a sweet spot, somewhere between -12 LUFS and -16 LUFS, where the balance between clear speech reproduction and comfortable listening is optimal.
Yet, despite the advancements in audio measurement tools, surprisingly many audiobook producers continue to rely on less precise, older methods for managing dynamic range. This is a concerning trend as it can lead to suboptimal audio quality. In the realm of audiobooks where listeners often seek a relaxing and engaging experience, the clarity and nuance of the audio play a vital role in keeping listeners engaged.
Fortunately, new technological advancements, particularly in the field of voice cloning, offer exciting possibilities for manipulating dynamic range in audio. The capacity to modify a cloned voice's intensity to align with the desired range of the audiobook presents unique creative potential. Producers can now craft audio that more precisely mirrors the intended emotions and narratives within the story, thereby enhancing the listeners’ immersion.
Interestingly, analyzing popular audiobooks has highlighted a clear relationship between the quality of dynamic range management and audience engagement, indicated by listener ratings. Audiobooks with well-balanced dynamic range tend to receive higher accolades, suggesting that listeners subconsciously value a natural and clear sonic environment.
The dynamic range concept also highlights the importance of specific frequencies within human speech. Most human vocal communication occurs within a certain frequency range. When this is understood and implemented in dynamic range analyses, producers can more readily target those frequencies for clarity and optimal intelligibility, enhancing the audiobook's experience.
The rapid progress in machine learning and related audio analysis software is further changing the field. By automating dynamic range measurements, these tools are enabling engineers to spend less time on manual processes, thereby focusing more on the creative aspects of crafting captivating audio content. This evolution suggests that the future of audio production for spoken-word content, like audiobooks and podcasts, will rely on a deeper understanding and mastery of dynamic range to ensure clear, engaging audio.
How Voice Data Analytics Transforms Audio Book Quality 7 Key Metrics to Track - Voice Consistency Scoring Using Advanced Spectral Analysis Tools
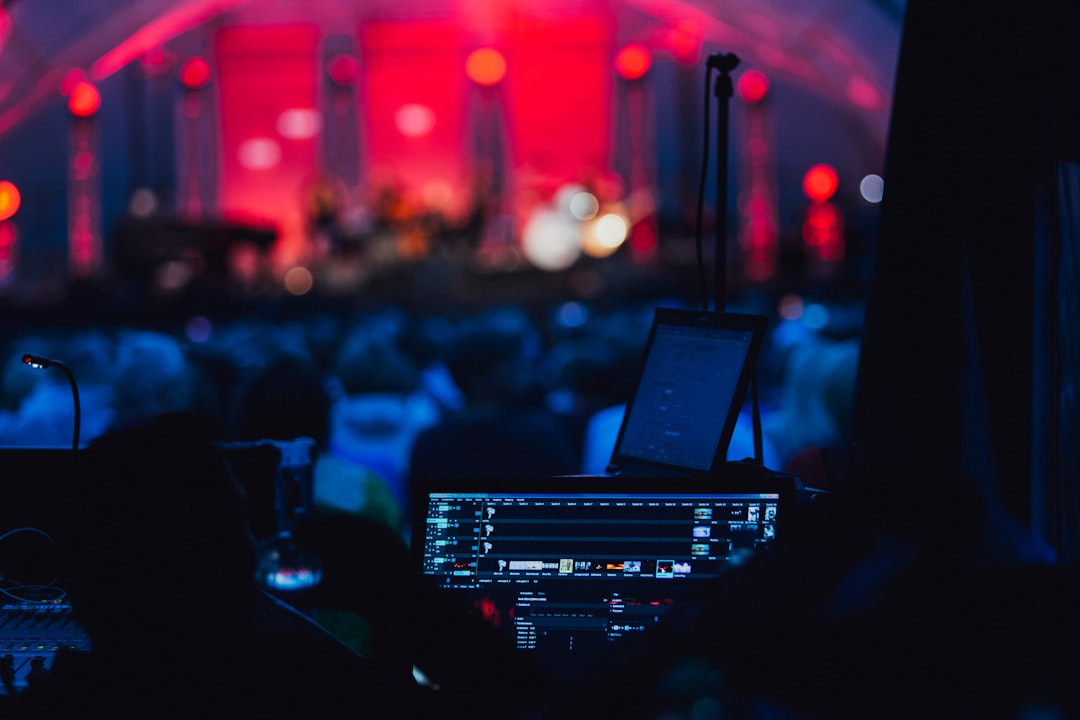
Voice consistency scoring is a new frontier in audio production, particularly relevant for audiobooks and voice cloning. It utilizes sophisticated spectral analysis tools to dissect a voice's characteristics across different dimensions like time, volume, pitch, and spectral makeup. This level of detail allows for a much more nuanced understanding of a voice's performance throughout an audiobook.
Key vocal features like the natural rise and fall of pitch (fundamental frequency contours) and the unique resonances of a speaker (formants) become quantifiable through these analytical tools. Producers gain a much more precise way to judge a voice's consistency and overall quality. This is further enhanced by incorporating multi-faceted evaluation approaches like the Acoustic Voice Quality Index, which can help correlate objective measurements with perceived vocal quality.
There's also an increased push to standardize vocal analysis methods. While still a developing field, standardization helps ensure that the results across different studies and projects can be more easily compared and understood. It is critical to note this standardization is to improve consistency of measures and not to impose a particular style of voice. Ultimately, if producers and voice cloning specialists can improve the consistency of the voice within the audiobook, it will be easier for listeners to maintain focus on the story and not get distracted by the vagaries of the voice. This is especially important in audio books where listening experiences depend on the clarity and flow of the narration. In short, it represents a more scientific and rigorous way to maintain voice consistency and can help make the listener's experience more enjoyable.
Voice consistency scoring leverages sophisticated spectral analysis tools to dissect the intricate frequency patterns within audio signals. By meticulously examining these frequency components, we can gain a deeper understanding of vocal characteristics, which is vital for creating seamless and natural-sounding voice clones in audiobooks. These algorithms not only identify average frequencies but also chart how those frequencies change over time. This temporal analysis allows audio engineers to spot subtle inconsistencies that human listeners might miss, playing a pivotal role in editing and refining audiobook recordings.
Human hearing perceives audio as having a kind of "texture" based on the blend of fundamental frequencies and their associated overtones, what we call the harmonic content. Examining this textural element is instrumental in producing more realistic and believable voice clones. It ensures that the synthetic voice retains the organic and fluid quality of natural speech, enhancing the overall listener experience.
In the world of voice cloning, the distinct spectral fingerprints associated with different emotional states can significantly affect how listeners perceive a story. Research has indicated that a lower frequency spectral envelope in an audio clip is often perceived as more sincere and genuine, which can be a powerful tool for enhancing the impact of storytelling in audiobooks.
The concepts of dynamic range and spectral analysis are tightly interwoven in audiobook production. Advanced audio analysis tools can pinpoint specific frequency bands where dynamic range compression might inadvertently obscure important speech cues. This ensures that every nuance of expression and intonation remains intact, preserving the richness and authenticity of the original voice.
Furthermore, human perception of voice consistency is surprisingly acute. Psychoacoustic studies suggest that we can detect even the slightest abrupt changes in voice consistency, sometimes within just a few milliseconds. Maintaining vocal consistency throughout audiobook narration is thus crucial for preventing listener fatigue and ensuring engagement.
While significant advancements have been made in the realm of voice cloning, occasional inconsistencies in vocal quality can still manifest as subtle artifacts. These artifacts inadvertently reveal the current limitations of the underlying algorithms, highlighting the ongoing need for improvements in voice analysis techniques.
The effectiveness of machine learning models employed for voice consistency analysis relies heavily on large and diverse datasets. However, many audiobook producers still don't leverage the wealth of existing voice data, hindering progress in audio quality. Wider adoption of readily available voice datasets could significantly advance the quality of audiobook production.
The link between voice consistency and the perception of emotions is striking. Listeners often develop a stronger connection with audiobooks when vocal fluctuations are minimized and smoothed. Advanced spectral analysis can quantitatively assess these vocal variations, providing objective measures for scoring and refining the quality of cloned voices.
The democratization of voice cloning technologies is driving a growing demand for sophisticated voice consistency analysis. As this trend continues, it's likely to fuel even greater creative possibilities for audiobook production. Listeners are becoming increasingly discerning, with heightened expectations for natural and immersive audio experiences. This push for quality will likely fuel ongoing innovation within the field.
How Voice Data Analytics Transforms Audio Book Quality 7 Key Metrics to Track - Emotional Expression Detection with Neural Pattern Recognition
Neural pattern recognition has emerged as a powerful tool for detecting emotional expression within audio data. This technology is transforming how we create and perceive audio content, particularly in audiobooks and voice cloning. By analyzing subtle variations in speech, such as pitch, tone, and rhythm, these systems can identify and categorize the emotional states being conveyed by a speaker. This ability to 'read' emotions from a voice is proving invaluable for enhancing the quality and impact of audio productions.
Several approaches, such as deep neural networks and EEG-based emotion recognition, are being employed to dissect and interpret the intricate patterns within audio signals that correspond to different emotional expressions. These techniques can help creators understand how a voice conveys joy, sadness, anger, or other emotions. The hope is this translates into creating audio experiences where emotion is more authentic and immersive.
The potential applications for this technology are quite broad. In audiobook production, it can assist in training voice clones to emulate a wider range of emotional nuances, thereby enhancing storytelling. For podcasts, emotional analysis could be used to adapt the style or pacing of narration based on the intended message or emotion. While still a developing field, the ability to recognize and manipulate emotional expression in audio is poised to significantly influence how we experience and interact with spoken-word content.
It's important to be cautious about this technology, as it can potentially be used to create artificial or overly manipulated emotions. If not applied judiciously, it could undermine the authenticity of a narrator's voice. Nevertheless, the ability to discern emotional content from audio has opened a new frontier in audio production. As these technologies mature and become more sophisticated, we can anticipate them playing an increasingly important role in shaping the quality and depth of audio experiences.
Neural pattern recognition is becoming increasingly adept at deciphering emotional expression from voice data, which is quite valuable in audiobook production. These techniques essentially treat vocal patterns as markers for different emotional states, offering a way to subtly enhance the emotional engagement of a story. Interestingly, emotional expression isn't just about the tone; it affects the very texture of a voice, altering frequency content and how pitch changes over time. We're starting to see AI models that can recognize complex emotions like happiness, sadness, and even anger, not just through words but from the nonverbal aspects of voice. It's pretty amazing that even tiny shifts in speech timing, maybe just a millisecond of delay or speed, can provide clues about what a person is feeling.
There's a fascinating connection between how emotions are expressed in a voice and how hard it is for a listener to understand what's going on. If a narrator conveys emotions genuinely, it seems to reduce the mental effort needed to follow the story, making it easier to grasp complex narratives. Modern neural networks can even analyze emotions in real time while someone's recording. This offers a cool opportunity for narrators to get instant feedback on their emotional delivery and fine-tune their performance on the spot. It's also worth noting that how emotions are shown through voice varies across different cultures. Thankfully, the neural network models being developed are being trained with diverse datasets, hoping to improve the sensitivity of the analysis for different cultures.
Several studies have shown that audiobooks with more emotionally expressive narrators have better listener retention. The theory is that modulating one's voice to convey emotion has a tangible impact on audience engagement. Some researchers are even working on ways to create cloned voices that can express a specific emotion without altering the core aspects of the original voice. This is exciting, opening the door to much more versatility and tailoring within audiobook production. It's not just the ability to identify basic emotions, either; neural networks are showing promising results at recognizing subtle emotional shades, like nostalgia or even irony, which are useful for enhancing narrative complexity. This entire field is still evolving, with new machine learning techniques and neural network architectures continually being integrated to further hone emotional detection abilities from audio. The potential for creating more captivating and emotionally resonant audiobooks is pretty compelling.
How Voice Data Analytics Transforms Audio Book Quality 7 Key Metrics to Track - Pacing and Rhythm Tracking for Optimal Listener Engagement
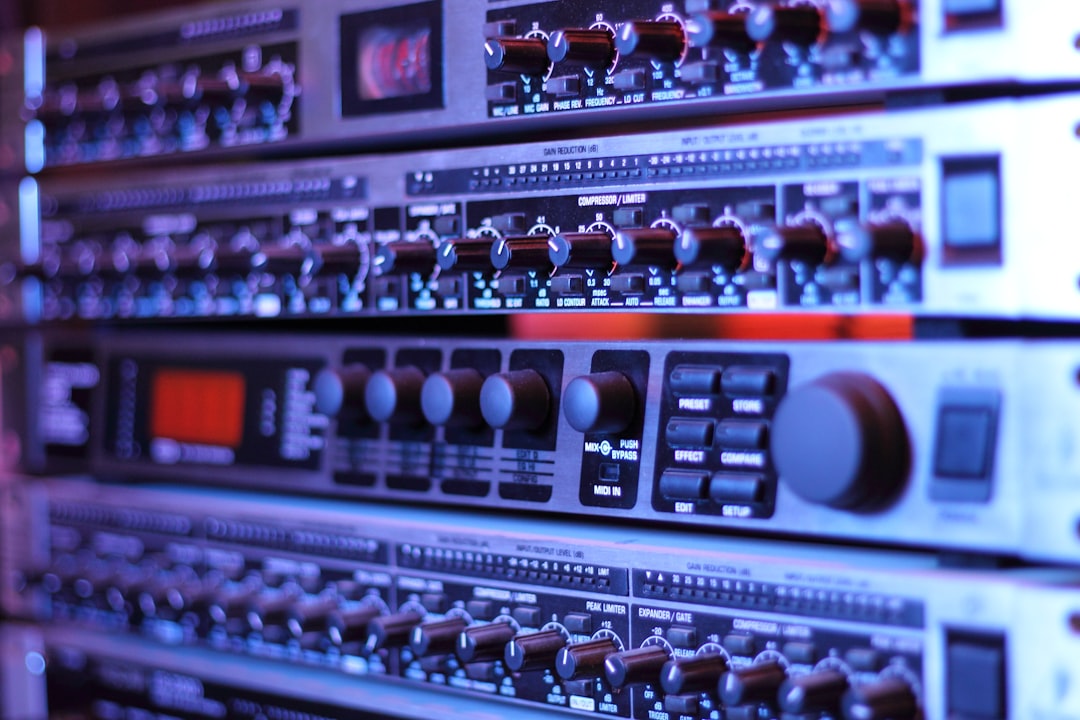
Pacing and rhythm are fundamental aspects of audiobook narration that significantly impact listener engagement. A well-crafted rhythm creates a flow that naturally draws the listener into the story, making it easier for them to follow the narrative. By varying the pace of delivery, narrators can emphasize key plot points, generate suspense, or evoke specific emotions in the listener. The strategic use of rhythmic variations helps to maintain listener interest, guiding their emotional responses and fostering a more immersive experience.
The ability to identify and analyze these patterns through voice data analytics has transformed how audiobook producers approach quality control. It helps them achieve a more consistent and engaging delivery that ultimately enhances the listener's experience. If the pacing is off or the rhythm is inconsistent, it can disrupt the flow of the story and potentially lead to listener fatigue. This is especially true in audiobooks where the listener relies solely on the audio to construct a mental picture of the narrative. Producers and narrators are now better equipped to fine-tune these aspects, allowing them to control and optimize the impact on the listener.
While maintaining a natural and consistent tone is key, some variation is important for an engaging audiobook. The key is to use pacing and rhythm strategically to maximize the impact of the story on the listener, whether that involves creating suspense or enhancing emotional resonance. In the competitive landscape of audiobook production, mastering pacing and rhythm tracking through voice data analytics allows producers to improve the listener's experience and ensure that their audiobooks remain competitive and in demand.
The way a voice moves through a story, its pacing and rhythm, has a profound effect on how listeners engage with the content. Our brains are finely tuned to the timing of speech, noticing even minuscule changes in rhythm as small as a few hundredths of a second. If the pace is inconsistent, it can pull a listener out of the story or cause confusion. This highlights the importance of controlling pacing, especially in audiobooks.
Interestingly, the speed of narration can heavily influence how well people understand what they are hearing, particularly when a story is complex. Slower delivery can actually enhance comprehension, potentially leading to better retention of details. This suggests that audiobook producers should carefully consider the relationship between the story's content and the rhythm used to tell it.
Beyond just clarity, the pacing of speech can also evoke emotions. A faster pace can generate excitement or a sense of urgency, while a slower pace can contribute to a feeling of calm or sorrow. This opens a creative door for audiobook creators to use pace as a storytelling tool to heighten emotional moments within a narrative.
Moreover, the rhythmic structure of speech seems to engage certain parts of the brain, like the auditory cortex. This suggests that a well-structured rhythm isn't just a stylistic choice but a way to potentially encourage deeper processing of the audio content. This leads to a stronger connection between what is heard and the listener.
Even the social dynamics of communication are influenced by pacing. For example, a long pause in speech can communicate thoughtfulness, while rapid speech might show excitement. Audiobooks can leverage these natural tendencies of speech to help listeners feel closer to characters or the narrative's overall tone.
However, pushing the pace too quickly can be a problem. It can overwhelm listeners, creating a kind of cognitive overload that prevents them from easily following the story. This places a burden on producers to find a balance between the right kind of flow and the complexity of the information being presented.
In the not-so-distant future, we will likely see audiobooks that can adapt their pacing in real-time, reacting to how well a listener is engaged. AI algorithms coupled with voice data analysis could allow the narrator's delivery to adjust dynamically. This technology could truly enhance the listening experience by optimizing pacing to retain audience focus.
The rise and fall of a voice, what's called prosody, is heavily impacted by pacing. This modulation, involving changes in pitch and tone, is a significant contributor to maintaining audience interest. It creates an added level of emotional expression that can keep listeners connected to the audio content.
Silence is a surprisingly powerful aspect of rhythm. Pauses, if well-placed, can build tension or allow certain moments to linger more significantly in the listener's mind. It shows that rhythm is not just about speed; it's also about knowing when to be quiet.
Finally, we must also realize that how listeners perceive pacing can differ across cultures. A pace that works perfectly in one part of the world may be off-putting in another. This emphasizes that if an audiobook wants to reach a truly broad audience, then understanding and considering the cultural dimensions of pacing is essential.
While there's still much to learn about exactly how these elements impact listener behavior, we're already starting to see that a better understanding of pacing and rhythm can be a key factor in audiobook creation. By continuing to study how these factors interact with human cognition, it's likely that we will find even more powerful ways to engage listeners in audio content.
How Voice Data Analytics Transforms Audio Book Quality 7 Key Metrics to Track - Background Noise and Audio Artifacts Detection Systems
In the world of audiobooks and podcasting, the ability to identify and remove unwanted sounds is becoming increasingly crucial. Background noise and audio artifacts, like pops, clicks, or hums, can significantly detract from the listening experience, hindering the listener's ability to fully immerse themselves in the narrative. These issues can stem from a variety of sources—the recording environment, equipment limitations, or even subtle flaws in the recording process. The goal of background noise and audio artifacts detection systems is to pinpoint and isolate these problematic elements, improving the overall clarity and quality of the audio.
The development of more sophisticated Voice Activity Detection (VAD) systems, powered by deep neural networks, represents a significant leap forward in this field. These advanced systems can more precisely distinguish between spoken words and various types of background noise, a capability that is essential in environments with a high level of ambient sound. Beyond noise reduction, these systems can also have broader applications. They can play a role in the automation of subtitle generation, for example, by helping to identify precise start and end times for each spoken segment. This improves the synchronicity of the audio and visual content, resulting in a better experience for viewers.
However, as with any powerful audio processing tool, there is a need for caution. An overzealous application of these noise reduction technologies could inadvertently remove subtle variations in voice that contribute to a more natural and engaging listening experience. Striving for complete audio purity should be carefully balanced with the need to preserve the richness and authenticity of the speaker's voice, especially in formats like audiobooks that are heavily reliant on conveying emotion and narrative through tone and inflection. The future of audiobook quality relies on refining these technologies, striking a balance between pristine audio and a voice that feels genuine and human.
Voice activity detection (VAD) is crucial for separating speech from background noise, especially in noisy environments where audiobooks are often recorded. It's becoming increasingly apparent that even subtle background noise can detract from listener experience, potentially causing fatigue and impacting comprehension, making noise reduction a key aspect of producing high-quality audiobooks.
Audio artifacts, often stemming from the digital conversion process, can introduce unwanted distortions like clipping or aliasing. These can be jarring interruptions, particularly within the smooth delivery expected in audiobooks. Producers and engineers are faced with the challenge of minimizing their occurrence to ensure a polished, enjoyable experience for the listener.
Another interesting phenomenon, frequency masking, highlights how louder sounds can obscure softer ones. This can lead to certain vocal frequencies being lost or muffled when background noise is present, making specific words or phrases difficult to distinguish. It's a complex interaction between the different frequency components of a sound that can have a surprising impact on the quality of audiobooks.
Thankfully, advancements in technology have led to the creation of real-time feedback systems that allow for on-the-spot analysis of audio quality during recording. This offers the narrator immediate insight into the quality of their recording, helping them avoid unwanted noise and optimize their delivery, thus improving the final product from the very beginning.
The recording environment itself plays a huge role in the presence and type of background noise. Techniques such as soundproofing and acoustic treatment are critical for ensuring recording quality. The decision of where to record, or the choice of studio, needs careful consideration to produce audio as clean as possible.
It's surprising how sensitive listeners are to background noise. Research indicates that the human auditory system is incredibly adept at picking up changes in background sounds, even if we are not aware of it consciously. This awareness informs the importance of a careful approach to engineering a positive listening experience.
Fortunately, machine learning and other detection algorithms continue to improve their ability to identify background noise and artifacts with speed and accuracy. This translates into improved workflows for editing and refining the audio. The result is a more efficient editing process which helps ensure a polished audiobook is delivered to listeners.
There's also a fascinating interaction between sound quality and the emotional context of the audiobook. A calm and relaxing narrative can be shattered by intrusive noises, highlighting how a proper approach to sound design and noise management are crucial for effectively supporting the emotions of a story. Conversely, a suspenseful scene might benefit from a carefully-chosen ambient soundscape to heighten the tension.
There's a growing interest in crafting specific soundscapes, for example using biophonic elements (natural sounds like birdsong or water). These can create an immersive and nuanced auditory experience that complements a story when used skillfully. However, they can also introduce a distracting element if not carefully managed.
The use of automated noise reduction technologies is now becoming standard practice in audiobook production. These technologies are remarkably good at separating speech from unwanted background noise, generating a more refined audio product that's less distracting for the listener, helping them fully focus on the narrative being delivered. The goal is to create an environment where the story, the words, the voice, are the central point of attention.
How Voice Data Analytics Transforms Audio Book Quality 7 Key Metrics to Track - Cross-Chapter Voice Consistency Analysis Through Machine Learning
Cross-chapter voice consistency analysis, a relatively new area of focus in audiobook production, leverages machine learning to ensure a consistent listening experience across the entire book. It delves into the finer details of vocal performance by scrutinizing attributes like pitch, volume, and the voice's spectral makeup. This allows audiobook producers to pinpoint subtle variations that might otherwise negatively affect audience engagement. Essentially, it enables a more nuanced understanding of the speaker's voice, ultimately refining the overall consistency and quality of the audio.
As the appetite for high-quality audiobook content increases, the use of sophisticated voice analysis tools has become more prominent. These tools play a vital role in crafting immersive listening experiences that keep the audience captivated. However, it's a balancing act. Employing advanced technology needs to be weighed against the delicate art of storytelling, where subtle vocal nuances can be key to expressing emotion and engaging listeners on a deeper level. Striking the right balance will likely remain a challenge as the field continues to evolve.
Cross-chapter voice consistency analysis, powered by machine learning, has become vital in audio productions like audiobooks. By meticulously examining vocal characteristics such as pitch, volume, and spectral patterns, it allows us to understand and quantify the nuances of a voice across different chapters. This is especially important in audiobooks where consistent vocal performance is essential for listener engagement and immersion. We're gaining a more in-depth understanding of how the spectral content of a voice relates to emotional expression. This allows us to fine-tune voice clones for audiobooks to better reflect a character's emotional state in a story. These insights are becoming more actionable as machine learning algorithms enable real-time feedback during audiobook recordings. Audio engineers can now guide a narrator to improve vocal consistency, pacing, and emotional delivery, potentially increasing audience comprehension and retention.
Research on listener behavior suggests that inconsistent pacing can increase cognitive load and make it harder to grasp complex narratives. On the other hand, a consistent pace, especially when paired with well-managed dynamic range, can promote listener engagement by reducing the effort needed to follow a story. It's quite fascinating how perceptive our ears are to inconsistencies in vocal delivery. Studies reveal that even subtle variations in pitch or tone can be detected by listeners within mere milliseconds. This highlights the importance of maintaining a consistent voice, which can prevent listener fatigue and enhance immersion.
We're also seeing how well-managed dynamic range and nuanced emotional expression within audio are linked. Audiobooks that incorporate both elements tend to receive higher listener ratings, suggesting that these qualities contribute to a more natural and engaging listening experience. Thankfully, advances in audio analysis are bringing more automation to quality control. These tools can efficiently assess large quantities of audio data, identifying potential areas where inconsistency or unwanted artifacts negatively impact the listening experience. The influence of cultural norms on how listeners perceive pace and rhythm shouldn't be underestimated either. A delivery that works well in one culture might not translate seamlessly to another, prompting the need for producers to carefully consider the target audience.
The interplay between emotional content, noise, and artifacts is also being explored. Advanced noise reduction technologies are becoming more sophisticated, allowing for the removal of unwanted sounds without negatively affecting a voice's natural expressiveness. Even subtle changes in speech timing, which neural networks can now start to detect and analyze, offer intriguing opportunities to personalize audiobook experiences. As these capabilities improve, audiobook producers will likely have more tools to shape listener emotions, leading to more immersive and engaging narratives. However, there are still concerns about overly manipulating emotions in audio, and it's vital to find a balance between enhancing emotional depth and maintaining authenticity. The future of audiobook production may well rely on a deeper understanding of these subtle interactions between vocal features, emotional context, and listener perception to provide richer and more immersive audio experiences.
How Voice Data Analytics Transforms Audio Book Quality 7 Key Metrics to Track - Word Pronunciation Accuracy Through Phonetic Mapping
"Word Pronunciation Accuracy Through Phonetic Mapping" is a relatively new area of focus in audio production, especially audiobook and podcast creation, that emphasizes the importance of clear and correct pronunciation. This technique utilizes advanced acoustic models to break down speech into its fundamental sounds, or phonemes, allowing for a very detailed assessment of pronunciation accuracy. In the past, this required creating phonetic transcriptions, but more recent approaches can assess pronunciation accuracy at the word level without this step, simplifying the process.
Machine learning plays a vital part in this process, using pronunciation dictionaries and other resources to automatically assess how well a speaker delivers each word. The push for clearer and more understandable spoken content, especially when dealing with audiences from diverse backgrounds, means phonetic mapping is gaining a larger role in audio production. This is important because good pronunciation is key to making sure the audience stays engaged and understands the audio they are listening to. It contributes to an enhanced listening experience by improving clarity and comprehension, which is crucial for ensuring quality in the increasingly competitive audiobook and podcast landscapes.
Phonetic mapping, a technique rooted in the International Phonetic Alphabet (IPA), is increasingly vital in audiobook production. It essentially breaks down speech into its fundamental sounds, known as phonemes, allowing for incredibly detailed analysis of pronunciation accuracy. This capability is key for creating high-quality audiobooks, especially when we want to have voices that sound natural and are easy to understand.
However, the challenge is that not every language is equally well-suited to phonetic mapping. Tonal languages, like Mandarin Chinese, present difficulties due to their reliance on pitch changes to distinguish meaning. Creating effective voice clones for such languages requires specialized phonetic mapping systems, which can be a real hurdle in audiobook production.
Interestingly, studies show a strong link between accurate pronunciation and improved listener comprehension. When audiobooks utilize precise phonetic representations, listeners retain information much better, particularly in educational settings. This connection between clear word pronunciation and effective learning underlines the importance of phonetic accuracy in audiobooks.
It's important to understand that the impact of phonetic mapping extends beyond just word accuracy. It also influences the conveyance of emotion. Tiny changes in the way a sound is made, the subtle phonetic shifts, can alter the feeling of a phrase. This means that narrators can use phonetic mapping to fine-tune the emotional weight of a sentence, leading to a more engaging and nuanced experience for the listener.
Fortunately, the latest phonetic mapping technology often includes real-time feedback during recording. This allows narrators to adjust their pronunciation instantly, minimizing post-production work and increasing efficiency in the production process.
Furthermore, machine learning is starting to make a big impact on the realm of dialects and accents. We're seeing a shift where phonetic mapping systems can now accommodate the distinct features of various speech patterns, allowing producers to generate more realistic and authentic-sounding voices. This feature can be very useful in audiobooks where representing different cultural or regional contexts can increase the story's relatability.
On the other hand, poor pronunciation can significantly increase cognitive load for the listener. This means that understanding speech that is not perfectly clear requires more mental effort. Thankfully, accurate phonetic mapping can reduce this load, enabling listeners to fully focus on the narrative itself, rather than on deciphering poorly-pronounced words.
Phonetic mapping is remarkably effective in distinguishing between similar-sounding words. In stories that have complex and nuanced dialogue, this capability is essential to avoid confusion. The listener's experience is much improved when words are presented clearly and are easily differentiated, even when they sound almost identical.
Recently, improvements in machine learning models have dramatically increased the accuracy of phonetic mapping. These models can analyze vast quantities of voice data, extracting the fine details of how humans speak. This leads to voice clones that are extraordinarily precise and life-like.
Finally, it's important to recognize that cultural norms play a significant role in pronunciation. Certain words may be pronounced slightly differently in different parts of the world. Accounting for these subtleties is a key part of culturally sensitive audiobook production. It ensures a wider audience can engage with and enjoy the content. Ultimately, while phonetic mapping offers a powerful tool for audio creation, ongoing research and development will be crucial in pushing the boundaries of this field and bringing greater precision to audio production.
More Posts from clonemyvoice.io: