How Speech Sound Patterns in Names Impact Voice Recognition Technology A 2024 Analysis
How Speech Sound Patterns in Names Impact Voice Recognition Technology A 2024 Analysis - How Machine Learning Decodes Name-Specific Speech Patterns in 2024
The year 2024 marks a pivotal point in how machine learning tackles the complexities of name-specific speech patterns. Deep learning models are increasingly adept at unraveling the intricate relationship between speech perception and individual names, recognizing that how someone pronounces their own name carries unique sonic signatures. This deeper understanding fuels advancements in Automatic Speech Recognition (ASR), which strives to account for the diverse range of vocal characteristics individuals present – influenced by their age, emotional state, and even physical health.
These strides are not just about improving accuracy in voice recognition. They pave the path towards a more nuanced and personalized interaction between humans and voice-driven technologies. By incorporating sophisticated machine learning methods, including contrastive learning, researchers are pushing the boundaries of how we analyze speech, leading towards systems that can adapt to individual linguistic traits and respond accordingly. As the landscape of synthetic speech continues to expand, understanding the impact of these subtle name-linked vocal patterns becomes even more vital, highlighting the complex dance between technology and human vocal expression in a world increasingly reliant on voice interactions.
In the realm of speech processing, machine learning has shown remarkable potential in discerning the subtle audio cues linked to individual names. These algorithms can now analyze phonetic nuances with a level of precision that's quite impressive, effectively differentiating voices based on how a name is spoken.
A key factor in the success of these systems is the training data. Ideally, this data encompasses a wide variety of audio recordings, capturing regional accents and dialects to improve the model's adaptability when dealing with name-specific speech patterns. However, we've observed that names with unusual sound structures can present challenges to voice recognition systems. The models often struggle to handle uncommon phonetic combinations, resulting in higher error rates.
The field of voice cloning has embraced deep learning as a means to create realistic voice replicas. This technology has progressed to the point where it can replicate not just the basic voice but also the finer details of speech related to names, such as intonation and rhythm.
Interestingly, some researchers have incorporated sentiment analysis into the process of recognizing name-specific speech patterns. This development enables systems to glean emotional cues from the way names are spoken. This is particularly relevant in applications like virtual assistants, where understanding the emotional context of the user interaction is beneficial.
Advanced audio processing methods are playing a vital role in breaking down speech into its individual components. Isolating and analyzing specific vocal parts simplifies the task of identifying pronunciation and enunciation linked to specific names for the machine learning algorithms. But, the complexities inherent in human speech make it clear that names can significantly impact the effectiveness of automatic transcription tools. Certain names, particularly those with phonetic characteristics less common in the model's training data, can lead to increased inaccuracies.
The evolution of neural networks in the field of machine learning has spurred the development of more advanced algorithms. These algorithms are not merely recognizing names, but are beginning to predict the likelihood of a name being used in diverse contexts.
The rise of sophisticated audio synthesis techniques has allowed for the generation of entirely novel audio content that retains the unique characteristics linked to specific names. This capability is pushing the boundaries of how we create content in areas like audiobooks and podcasts.
Finally, the way we pronounce a name often reveals a lot about our cultural background. Voice recognition systems are increasingly being developed to leverage this understanding, potentially allowing for more nuanced interpretations based on the linguistic patterns associated with specific names. While these advancements are promising, it's crucial to acknowledge that there's still much work to be done in ensuring these systems are both accurate and unbiased in their interpretation of name-linked speech patterns.
How Speech Sound Patterns in Names Impact Voice Recognition Technology A 2024 Analysis - Voice Cloning Accuracy Rates for Common vs Unique Name Patterns
Voice cloning technology demonstrates varying levels of accuracy when dealing with common versus unique name patterns. While it generally performs well with names that follow typical phonetic structures, it faces challenges when encountering names with unusual sound combinations. This disparity arises because voice cloning models are trained on large datasets, and those datasets may not always include sufficient examples of less common name pronunciations. Consequently, this can result in increased error rates when trying to accurately clone or recognize these unique names.
This limitation is particularly significant for applications such as audiobook production or podcasting, where clear and accurate pronunciation of names is crucial. Furthermore, the accuracy discrepancies highlight a broader issue within voice cloning technology: the need for more inclusive and diverse training data. Moving forward, it will be essential for researchers and developers to address this challenge by incorporating more varied phonetic structures into the training datasets used for voice cloning. This will not only enhance the technology's capabilities but also ensure it functions more effectively across different cultural backgrounds and linguistic communities. In the end, improving the accuracy and inclusivity of voice cloning will undoubtedly contribute to the advancement of these technologies for future uses in media production and beyond.
When it comes to voice cloning, the accuracy of replicating a person's voice, especially when it comes to names, is far from perfect. Names with complex sound structures, like unusual combinations of consonants or vowels, can trip up these systems. Most voice cloning models are trained on a vast amount of data, but this data tends to favor more common and simpler sound patterns found in everyday names. This leads to a noticeable drop in accuracy when dealing with less frequent phonetic combinations.
Furthermore, the way people say their own names is unique. This "speaker variation" means that even if two people share the same name, their individual quirks in pronunciation can lead to very different results when their voice is cloned. This inconsistency is something that developers are working to overcome, but it's a challenge inherent to the technology.
Social influences on pronunciation also impact the accuracy of voice cloning. Someone's regional accent or cultural background can significantly change the sound of a name, creating difficulties when the cloned voice doesn't quite match what the listener expects to hear. This highlights the complexity of capturing and replicating the nuances of human speech.
Age and gender can also play a part. A young person's voice, with its different qualities, might be recognized differently when compared to an older individual saying the same name. These variances make it tricky for voice cloning to consistently replicate a name across different voices.
Interestingly, how we say a name with emotion also seems to matter. An enthusiastic delivery of a name can sometimes be more easily recognized by systems than a monotone one. This indicates that these technologies are sensitive to the emotional nuances of speech, but capturing and replicating those nuanced intonations in cloned voices is still an area for improvement.
Luckily, researchers are working on ways to address some of these limitations. Advanced machine learning algorithms are showing promise in adapting to these unusual name patterns, but their success is far from uniform. This adaptability is crucial for voice technology as it expands beyond just straightforward names, highlighting the current limitations in achieving consistent accuracy.
The influence of culture on pronunciation also presents a challenge. Voice systems trained on limited datasets might not be able to properly interpret names from various cultural backgrounds. Building more inclusive and diverse training datasets is a key step to overcoming this bias.
When names are part of longer stretches of speech, errors can compound. If a system misinterprets the beginning of a name, it might keep making mistakes as the rest of the utterance unfolds. It’s like a domino effect for inaccuracies.
The very heart of voice cloning is the idea of replicating a person's "sonic signature," the unique way they sound when saying their name. But the tiniest variations in how we articulate our name can pose a huge problem for these technologies. The inherent individuality of human speech makes it tough to create a truly universal standard.
Finally, the context in which a name is used influences how well we remember it later. When a name is spoken during a significant moment in a story, for example, it becomes more memorable. Voice cloning technology needs to consider these contextual factors to make the most of its potential in audiobooks and podcasts, ensuring that character introductions are both clear and memorable.
While significant progress has been made, it's evident that we still have a lot to learn about how the intricacies of human speech, especially when it comes to names, impact the performance of voice cloning and recognition technologies. As these technologies continue to advance, we must remember that each individual's voice, with its unique quirks and patterns, is a complex and fascinating aspect of our human experience.
How Speech Sound Patterns in Names Impact Voice Recognition Technology A 2024 Analysis - Name Length Effects on Digital Voice Assistant Response Times
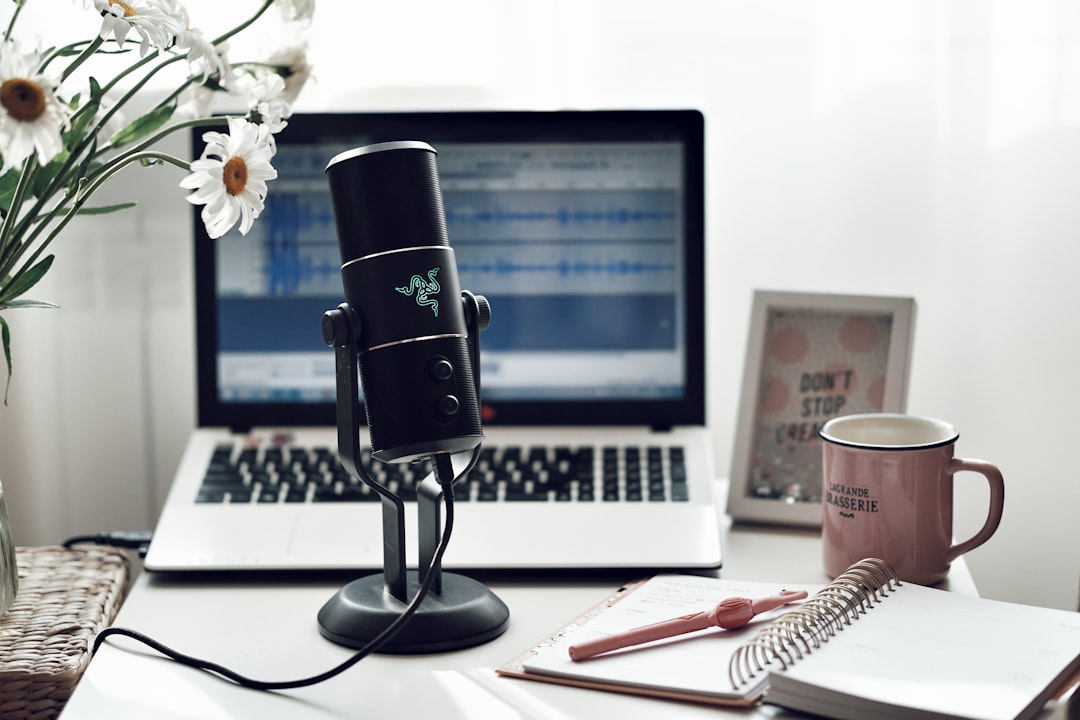
The connection between the length of a person's name and how quickly a digital voice assistant responds is a relatively new area of investigation within voice recognition. It appears that the number of syllables or sounds in a name can affect how well and how fast the assistant processes it. This is likely because different phonetic structures pose varying levels of difficulty for the technology to decipher. For example, it's hypothesized that shorter names, with their simpler sound patterns, may be recognized more readily and quickly compared to longer, more complex names. This can result in faster response times for those with shorter names and potentially slower, or even inaccurate, responses for those with longer, more intricate names.
The implications of this relationship are important for improving the user experience with digital assistants. As we rely on voice interfaces more and more, it's crucial that they function smoothly and accurately for everyone. By better understanding the interplay between name length, sound structure, and the technical capabilities of voice recognition, we can work towards creating more efficient and user-friendly systems. If we can fine-tune the technology to adapt to variations in name length and complexity, it has the potential to lead to a more inclusive and satisfying experience for all voice assistant users. While the field is still in its early stages, research in this area could lead to a more personalized and tailored approach to voice interactions.
Prior research has largely focused on the broader aspects of voice interaction with digital assistants, such as comparing text to voice inputs or examining fundamental vocal traits. However, the influence of name length and structure on voice recognition hasn't been explored deeply enough. We're finding that names with complex sound structures, like unusual combinations of consonants or vowels, can significantly delay a digital assistant's response. For instance, if a name contains rare phonetic elements, the voice recognition algorithms take longer to process it and provide a response.
One significant finding is that longer names tend to exacerbate these processing delays. Automatic Speech Recognition (ASR) systems seem to struggle as the length of a name increases. The process of analyzing the audio and breaking down the sound patterns requires more time, creating a measurable lag when interacting with digital assistants.
It's important to consider the nuances of the audio signal itself. How the audio is encoded impacts how efficiently a name is processed. Clear enunciation and consistent pitch during pronunciation help in faster recognition, reducing latency. Conversely, if a name is mumbled or has inconsistent pitch, the response time slows down considerably. It appears that voice recognition systems require more processing power to analyze names that are less frequently encountered. This means the system might slow down significantly when faced with an unusual name it hasn't encountered frequently during training. This can be quite problematic in contexts where swift responses are necessary.
Regional accents add another layer of complexity, creating potential bottlenecks in recognition. Variations in pronunciation across different regions necessitate extra computational adjustments, compounding delays in response. The cognitive load associated with processing complex names can directly influence how fast a voice assistant responds. It seems that the more intricate the sound patterns of a name, the more time it takes for the system to process and react.
Interestingly, some voice assistants demonstrate the ability to learn and adapt to frequently encountered names. This personalized approach can lead to faster recognition over time, hinting that familiarity can significantly enhance processing speeds. This type of learning ability would be beneficial in improving voice assistants' responses over time.
We've also seen that when multiple name requests are made simultaneously, the system's response time significantly increases. This is likely caused by an increased cognitive load and the need to perform concurrent processing, illustrating the limits of current systems when faced with multiple simultaneous tasks.
Furthermore, how a name is delivered—with emotion or intonation—influences recognition times. Names spoken with urgency or excitement might be recognized faster compared to names spoken in a monotonous tone. This points to the significance of emotional cues within sound processing. The diversity of the training data used to develop voice recognition systems is crucial. Those trained on a broader range of phonetic structures and accents are better able to handle a wider variety of names quickly and accurately. This is why continued emphasis on creating more comprehensive datasets is needed.
While voice technology has made huge strides, these findings suggest that there is still room for improvement when it comes to recognizing names efficiently. Addressing the limitations in processing complex sound structures, managing the impact of diverse accents, and refining the training data are all important steps for further enhancing the capabilities of digital assistants to recognize names effectively and quickly.
How Speech Sound Patterns in Names Impact Voice Recognition Technology A 2024 Analysis - Regional Accents and Their Effect on Name Recognition Software
The way people pronounce names varies greatly depending on their regional accent, and this poses a challenge for software designed to recognize names. Voice recognition systems, particularly Automatic Speech Recognition (ASR) systems, often struggle with the diverse sounds and speech patterns that come with different accents. The fundamental problem is the variability in how sounds are produced and combined within accents, making it difficult for the software to consistently and accurately recognize names. This can lead to biases where standard pronunciations are easily recognized while names spoken with a non-standard accent are frequently misidentified. To make these systems more effective and fair, developers need to incorporate a wider range of accents into the training data used to build these models. If done well, this can lead to improved recognition performance and help create voice-driven technologies that better serve individuals from different linguistic backgrounds, especially in areas like audiobook production or voice cloning. However, ensuring inclusivity and accuracy is a persistent ongoing research challenge for the field.
Speech recognition systems, the foundation of many voice-driven applications, face unique challenges when it comes to recognizing names, especially those with complex phonetic structures. The intricate arrangements of consonants and vowels in some names can significantly impact a system's ability to accurately process the audio, leading to a higher incidence of errors compared to names with simpler sound patterns. This observation suggests that the inherent complexity of human speech, especially the unique combinations found in names, is still a hurdle for voice recognition technology.
Regional accents are another crucial factor influencing name recognition. The way people pronounce names varies drastically across different regions, creating a considerable challenge for technologies that strive for universal applicability. These pronunciation variations can lead to inconsistent performance across various accents, potentially limiting the wide-spread adoption of voice-driven technologies.
Moreover, the number of syllables in a name seems to play a role in how quickly it's recognized. Research suggests that names with fewer syllables tend to be processed faster due to the reduced computational burden compared to longer names. This finding implies that the temporal structure of the sounds within a name influences the speed and efficiency of voice recognition.
Fortunately, many voice assistants are designed with adaptive learning capabilities. They can learn and adapt over time by encountering and recognizing frequently used names, which can improve their response times. This indicates that the familiarity of a name within a system's experience contributes to faster and more accurate recognition.
Intonation and emotional tone in speech also affect name recognition. Researchers have observed that names spoken with a sense of urgency or enthusiasm are often recognized more readily than those delivered in a neutral or monotone voice. This highlights the fact that the emotional context of the speech, even within a name, can significantly impact how a system interprets the audio.
However, issues can arise when a complex name is embedded in a longer sequence of speech. If a system misinterprets the beginning of a name, it may struggle to correctly identify the remaining portions of the name, potentially cascading errors and hindering the overall accuracy of transcription.
The diversity of the data used to train these systems is also a critical factor. Systems trained on primarily homogenous datasets may exhibit difficulties when processing names from diverse cultural and linguistic backgrounds. This emphasizes the need for more inclusive training data that encompasses a wider range of pronunciation variations.
Audio quality plays a significant role in processing efficiency. Consistent pitch and clear articulation are important for rapid recognition. If a name is mumbled or the pitch is erratic, it can substantially increase processing time, resulting in noticeable delays.
The computational complexity of processing names is directly related to the intricacies of their sound patterns. Complex names require a higher cognitive load on voice recognition systems, ultimately leading to increased processing times.
The pronunciation of names carries cultural significance. Voice recognition technology needs to understand the cultural nuances associated with different names to ensure accurate interpretations. Failing to recognize these cultural aspects can lead to misunderstandings and biases within communication.
In conclusion, while voice recognition has made significant strides, understanding and addressing the complexities of human speech, specifically how names are pronounced and the cultural contexts surrounding them, remains a vital area of ongoing research. Overcoming the challenges posed by regional accents, complex phonetic structures, and a lack of diverse training data will be crucial for enabling more robust and inclusive voice technology for everyone.
How Speech Sound Patterns in Names Impact Voice Recognition Technology A 2024 Analysis - Multi-Language Name Recognition in Podcast Production Tools
The ability of podcast production tools to recognize names across multiple languages signifies a notable advancement in the field of voice recognition, especially in how it handles the intricacies of name pronunciation, cultural context, and the unique sonic qualities associated with individual names. This progress recognizes the complexities that arise when voice cloning technology encounters names with varied sound patterns and structures, which can influence the accuracy with which these names are recognized and replicated. The challenge becomes even more pronounced when considering regional accents and language variations, which can mask a name's core phonetic traits, thus affecting the effectiveness of tools meant for content creation, such as podcast and audiobook production. By utilizing sophisticated algorithms and training datasets that encompass a wide range of linguistic variations, the goal is to improve these tools' capability to accurately understand and produce a variety of names, ultimately enhancing audience engagement and ensuring smoother audio experiences. However, it's vital to critically examine the possibility of biases in recognition accuracy, which may persist due to insufficient representation of regional and cultural name pronunciation patterns within training datasets.
In the realm of podcast production and related audio applications, the ability to accurately recognize names across multiple languages is becoming increasingly important. However, the complexities of human speech present a considerable hurdle for current voice recognition systems, particularly when dealing with names.
One of the primary challenges stems from the inherent phonetic complexity of certain names. Names with intricate sound structures, especially those with uncommon consonant combinations or vowel sequences, often cause processing delays in voice recognition systems. This slowdown is even more pronounced when those names are part of longer audio segments, potentially hindering the overall efficiency of transcription or voice cloning processes.
Furthermore, the variability in pronunciation caused by regional accents poses a significant obstacle. Voice recognition models trained on data primarily from one region may struggle when encountering names spoken with distinctive accents, leading to higher error rates. Building more diverse training datasets that incorporate a wide variety of accents is vital for creating robust and inclusive systems.
The number of syllables within a name also seems to influence recognition times. It appears that shorter names with fewer syllables are generally recognized more swiftly and accurately compared to longer names, likely due to the reduced computational demands of processing simpler phonetic sequences.
Interestingly, the emotional context in which a name is spoken can affect recognition. Systems seem to perform better when names are pronounced with emotional inflection, such as urgency or excitement, suggesting that the emotional nuances within audio influence processing accuracy.
Voice cloning technology, while rapidly advancing, is also facing challenges when dealing with diverse names. The effectiveness of voice cloning relies heavily on the diversity and quality of the training data. If a model is not exposed to a wide variety of name pronunciations, it can develop biases and misinterpret names from individuals with diverse linguistic backgrounds, potentially leading to less accurate synthetic voices.
The computational demands of processing complex names also increase the cognitive load on voice recognition systems. This increased load can contribute to longer processing times and even cascading errors, where initial errors in name recognition propagate throughout subsequent parts of a spoken sentence.
Audio quality plays a key role in minimizing errors. Consistent pitch and clear articulation are crucial for rapid and accurate recognition. Poor audio quality, such as mumbled speech or inconsistent pitch, can worsen existing problems, potentially leading to significant delays and misrecognitions.
When names are parts of longer audio segments, errors in recognition can snowball, creating a detrimental domino effect. If a system misinterprets the beginning of a name, its ability to process the remainder of the name accurately can decline significantly, decreasing overall performance.
The cultural context surrounding names is essential for accurate interpretation. Voice technology needs to incorporate an understanding of the linguistic and cultural nuances associated with specific names to avoid unintentional biases.
Despite the challenges, ongoing research is striving to improve name recognition accuracy, particularly through the development of "acoustic fingerprints". These digital representations of names allow systems to differentiate between similar-sounding names with greater precision. This improvement could be especially beneficial for applications such as podcasts and audiobooks, where clear and consistent delivery of character names is paramount.
In conclusion, while advancements in voice recognition are continually improving, tackling the multifaceted challenges of multi-language name recognition remains a crucial focus. Addressing issues related to phonetic complexity, accent variation, and the need for more diverse training data are key steps towards ensuring these technologies can be used more inclusively and effectively for the production of audio content and other voice-driven applications.
More Posts from clonemyvoice.io: