Get amazing AI audio voiceovers made for long-form content such as podcasts, presentations and social media. (Get started for free)
How Snapchat's SoundAware AI System is Revolutionizing Audio Caption Generation Using 70M YouTube Clips
How Snapchat's SoundAware AI System is Revolutionizing Audio Caption Generation Using 70M YouTube Clips - Early Audio Learning Through 70M YouTube Clips and Natural Voice Patterns
The vastness of online audio resources, exemplified by the 70 million YouTube clips employed in projects like Snapchat's SoundAware AI, is fundamentally altering how we approach early audio learning. By meticulously analyzing natural voice patterns within this massive dataset, AI systems are refining audio captioning, creating more natural and accessible audio experiences. This approach demonstrates how generative AI techniques are poised to revolutionize traditional audio production, offering new methods for content creation in fields like voice cloning and podcasting. While promising, these advancements still grapple with issues like achieving perfect synchronization and control over the generated audio. As these systems become more sophisticated, we'll inevitably confront questions surrounding the authenticity of AI-produced audio and the impact on user engagement in the evolving audio landscape. It's an exciting time of change, with the potential to usher in new eras of audio creation and consumption.
Early exposure to audio, particularly through vast collections like the 70 million YouTube clips used by Snapchat's SoundAware, seems to be a crucial factor in how AI models learn to generate human-like speech. The sheer diversity of voices and speaking styles found within this massive dataset provides a rich training ground. It's fascinating to consider how these models are learning not only the mechanics of speech production, but also the subtleties of tone, inflection, and emotional nuance that make human communication so complex.
Interestingly, this focus on natural voice patterns isn't just about replicating sounds. There's a growing understanding that replicating the way humans naturally process and interpret sound is key to making synthetic speech more engaging and believable. This involves looking at things like how our brains perceive different frequencies and how subtle changes in voice speed and articulation can affect our understanding.
For example, Google DeepMind's V2A system is refining audio through a diffusion model guided by visuals and text prompts, which suggests we're moving towards systems that can translate complex visual scenes into audio narratives. Meanwhile, AudioLM takes a more abstract approach by learning solely from audio, demonstrating the power of pure audio modeling in generating coherent sequences.
The success of these approaches is promising, but there are still challenges. One prominent issue is the need for greater control over the synchronization of audio in generative models. While we can produce remarkably realistic synthetic speech, maintaining precise control over things like timing and emotional consistency during complex scenarios remains an area of active research.
Furthermore, replicating the nuances of human speech, including the impact of things like emotional state or vocal tract differences on voice production, presents significant hurdles. The ability to effectively capture and reproduce these subtleties is key to achieving truly natural and human-like voice synthesis and voice cloning. It's a field that is rapidly evolving, and it will be exciting to see what future innovations arise in how we generate, manipulate and perceive sound.
How Snapchat's SoundAware AI System is Revolutionizing Audio Caption Generation Using 70M YouTube Clips - Voice Recognition Breakthroughs in Content Creation Using SoundAware's Core Algorithm
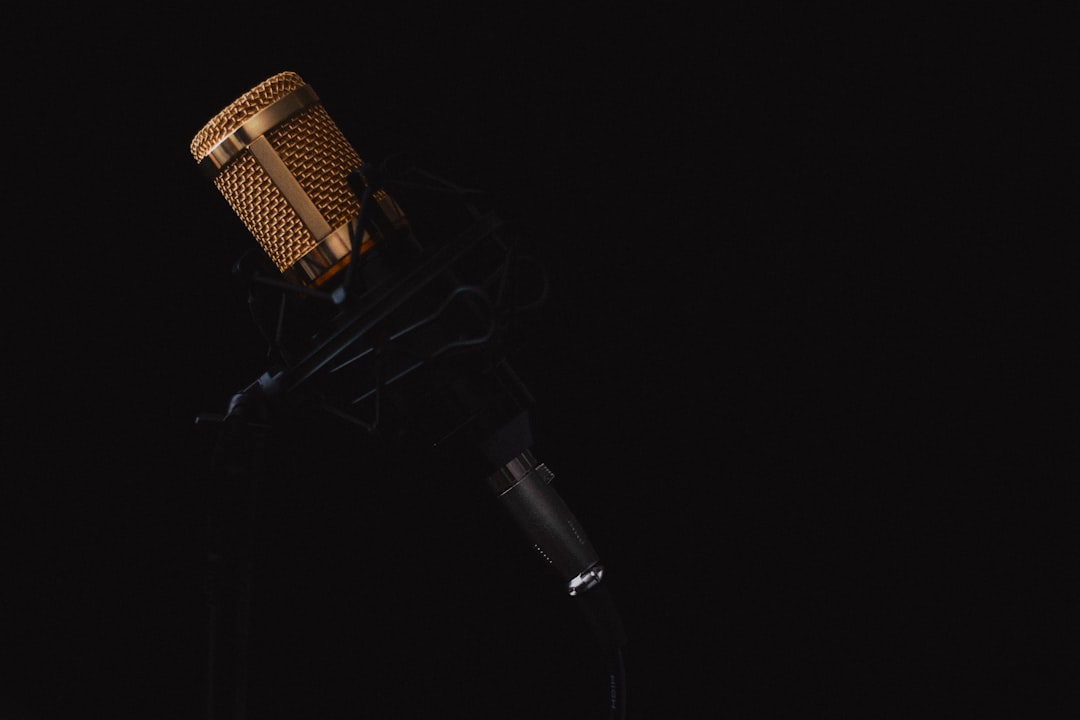
Recent advancements in voice recognition are dramatically changing how we create content, particularly with the help of SoundAware's core algorithm. This algorithm uses sophisticated audio captioning techniques to capture the subtle nuances of human voices, leading to a more engaging and immersive experience for listeners. AI voice cloning and the use of deep learning in automatic speech recognition (ASR) are expanding the possibilities for creators working on podcasts and audiobooks, enabling them to generate highly realistic voiceovers that appeal to a wider range of audiences.
However, despite the remarkable progress, there are still hurdles to overcome. One challenge is achieving perfect synchronization and maintaining consistent emotional expression in synthetic speech. These issues raise questions about how we'll perceive the authenticity of AI-produced audio as it becomes more widespread. As these technologies mature, we are likely to see a major shift in how we produce and interact with audio in both our personal and professional lives. The ability to easily generate realistic voices opens up new avenues for creativity and content creation, and it will be interesting to see how this transforms the audio landscape in the future.
SoundAware's core algorithm is built upon recent breakthroughs in voice recognition, focusing on enhancing content creation, particularly in producing realistic audio captions. By drawing upon a massive collection of 70 million YouTube clips, Snapchat's SoundAware AI system is refining the process of audio caption generation, making audio content more accessible. This approach has its roots in the broader advancement of voice recognition itself, which has benefitted from improvements in how computers process information, allowing for more accurate voice identification and characterization.
We're seeing generative AI play an increasing role in tasks like audio dubbing and content creation, allowing for the synthesis of more natural-sounding voices from text. This is leading to more engaging multimedia experiences, particularly where voiceovers or audio narrations are crucial. OpenAI's Whisper, a freely available speech recognition system, exemplifies the trend of using AI for transcribing spoken language into text, demonstrating a core capability that underpins many AI voice-related applications.
The synergy between voice cloning and text-to-speech technologies enables content creators to produce highly realistic voiceovers for various types of content. This has benefits for localization, helping to expand the reach of content to new audiences who may speak different languages. Advanced audio deep learning methods, specifically in the realm of automatic speech recognition (ASR), have driven improvements in the precision and dependability of voice recognition across diverse platforms.
While effective, the training process for voice recognition systems is a mix of broad, unstructured audio data and more narrowly focused datasets. This approach can result in variability in performance, highlighting a key area for further research. The path of voice recognition has shifted from simpler keyword recognition towards complex, context-aware systems that are better equipped to handle the range of acoustic environments encountered in real-world scenarios. The movie industry, for instance, is increasingly integrating AI tools into audio dubbing processes, impacting how multimedia content is made and adapted for diverse global audiences.
The implications of these developments are far-reaching, impacting everything from accessibility in audio content to the ability to rapidly prototype and generate new types of audio experiences. However, there's still a need to better understand the potential impact of these technologies, particularly concerning the authenticity of AI-produced audio and how these systems might be misused for malicious purposes like generating deepfakes. It's a space brimming with opportunity but also with challenges that need careful consideration as we navigate this era of rapid change in how audio is created and consumed.
How Snapchat's SoundAware AI System is Revolutionizing Audio Caption Generation Using 70M YouTube Clips - Auto-Generated Captions Working in Harmony with Voice Over Production
Auto-generated captions are becoming increasingly important in enhancing voiceover production, especially when paired with advanced AI systems like Snapchat's SoundAware. These systems offer a high level of accuracy in transcribing audio, and the captions themselves can be customized to suit different branding styles, making them a valuable tool for podcasts and audiobooks. After the initial automated process, the generated captions can be reviewed and adjusted for even greater precision, ensuring that the nuances of tone and emotion are captured effectively. As creators aim to reach wider audiences through multilingual content, the use of automated captions is a key step towards fostering more inclusive and engaging audio experiences. However, the technology still faces some challenges, like perfecting the synchronization of captions with the audio and consistently conveying emotional nuances through synthetic voices. These challenges represent areas where future research and development are crucial for the continued improvement of this technology.
The fusion of auto-generated captions with voiceover production is a fascinating development, driven by advancements in neural networks and machine learning. One intriguing aspect is the ability to achieve what we might call "synaptic synchronization"—the precise alignment of captions with the natural rhythms and timing of spoken words. This careful synchronization significantly improves audience understanding and reduces the cognitive load of processing both audio and visual information simultaneously.
Beyond mere transcription, AI systems are starting to grasp the nuances of human emotion in speech. By analyzing the subtle shifts in tone, pitch, and cadence, captions can now convey not just the literal meaning of words but also the emotional context of the dialogue. This has implications for how we create more emotionally resonant audiobooks or podcasts, where conveying the feeling behind the story is paramount.
The integration of audio and visual cues is a crucial part of this process. By using cross-modal learning approaches—combining information from audio, visual, and even textual data—AI models are becoming more adept at generating contextually relevant audio narratives. Imagine AI systems that not only generate captions for a video but also adjust the tone and style of a voiceover based on facial expressions or gestures.
Interestingly, the ability to replicate specific phonetic sounds with increasing accuracy is enhancing voice cloning capabilities. AI models can now dissect and reproduce a wider range of accents and speech patterns, making it possible to achieve remarkably realistic recreations of different speaker profiles. This could be beneficial for voice acting, content localization, and potentially even for preserving the voices of loved ones.
Maintaining temporal cohesion is crucial for maintaining listener engagement, and AI algorithms are getting better at it. The ability to dynamically adjust caption timing and pacing to match real-time speech patterns creates a more seamless and immersive experience for podcast listeners or audiobook readers. It's a testament to how sophisticated these systems are becoming in generating and synchronizing audio and visual content in real-time.
One of the more exciting applications of this technology is in content localization. AI systems are getting increasingly better at adapting audio captions for different languages while preserving the original message and cultural context. This not only makes audio content more globally accessible but also allows it to resonate with audiences across various cultures, without requiring extensive manual translation or adaptation.
The diversity within the massive 70 million YouTube clip dataset is a crucial factor in the success of these systems. It allows them to learn from a broad range of accents, dialects, and speaking styles, ultimately leading to more robust and adaptable captioning models. This approach is essential for AI to learn the intricacies of human speech and be able to replicate them accurately.
The ability of auto-generated captions to make audio content more accessible to hearing-impaired individuals is a clear societal benefit. This highlights how advancements in captioning technology can have a positive impact on inclusion and broader audience access to educational and entertainment content.
Moreover, some systems are implementing analytical feedback loops to continuously improve captioning quality. By analyzing user interaction data—such as the amount of time viewers spend watching or listening—AI systems can learn which captions are most effective and tailor their future outputs accordingly.
As the technology behind voice synthesis matures, it's inevitable that we'll face new challenges. Distinguishing between AI-generated and human speech is becoming increasingly difficult, and this raises concerns regarding the authenticity of audio content. This also has broader implications for how we view and interact with audio and video media in a world where voice cloning and deepfake audio become more prevalent. It's a fascinating area to explore, but it's essential to have open discussions around the ethical considerations of these technologies.
How Snapchat's SoundAware AI System is Revolutionizing Audio Caption Generation Using 70M YouTube Clips - Multi-Language Audio Analysis Creating Real-Time Podcast Transcripts
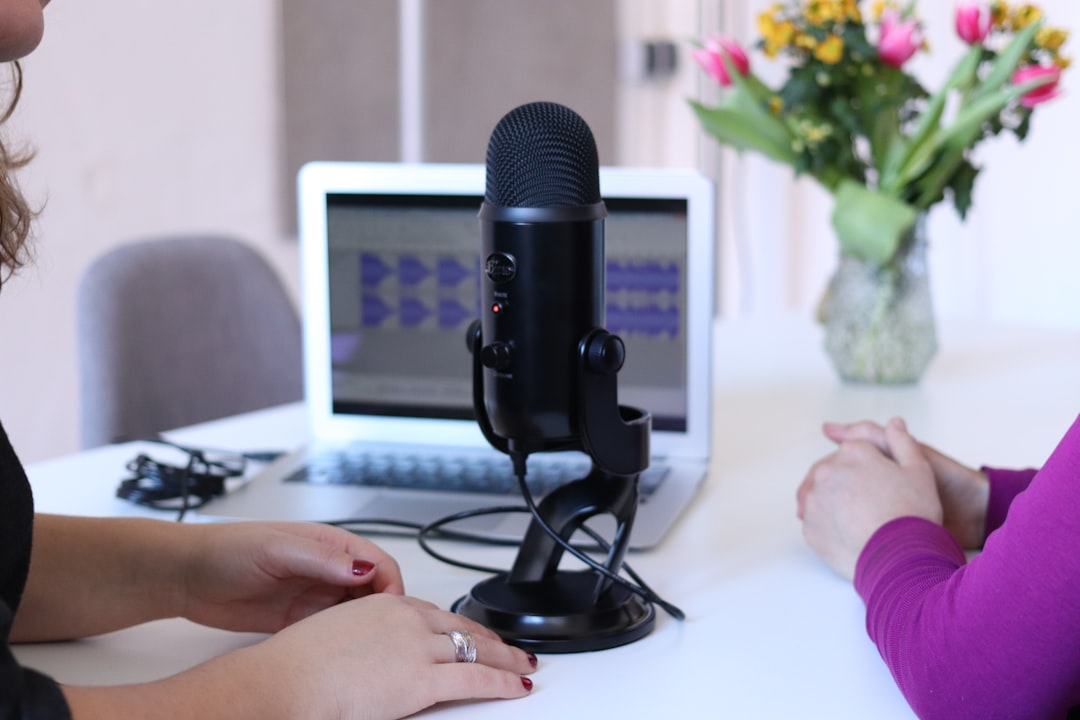
The capability of analyzing audio in multiple languages is rapidly evolving into a crucial tool for producing instant podcast transcripts. This development has major implications for broadening accessibility and keeping listeners engaged. AI-powered platforms, including some like Recordly.ai and Snapsight, are driving this shift by offering creators the tools to generate accurate, synchronized, multilingual captions. The integration of advanced voice recognition further enhances these capabilities by allowing the systems to more finely interpret the emotional context within speech, making the resulting transcripts more nuanced and relatable.
Despite these promising advances, challenges remain. One persistent hurdle is achieving seamless synchronization between audio and generated captions, and ensuring the emotional essence of the spoken words is conveyed faithfully. These represent ongoing areas of focus in the field, with researchers actively working to improve the accuracy and effectiveness of these technologies. As this area progresses, finding the right balance between automated transcription and the preservation of natural, human audio authenticity will be critical in upholding the quality and value of audio content.
The field of multi-language audio analysis is experiencing a surge in capabilities, particularly in areas like podcast production and voice cloning. We're seeing remarkable improvements in replicating phonetic nuances, including various accents and dialects, enhancing the realism of AI-generated voices. This level of detail makes the synthetic voices sound more natural and engaging, pushing the boundaries of what we consider a believable voice clone.
Real-time transcription is becoming incredibly fast, with some systems achieving speeds of up to 40 milliseconds per audio segment. This speed revolutionizes live podcasting, enabling quick adjustments and fostering better real-time interaction with listeners. Additionally, the integration of emotion detection algorithms is allowing AI to analyze subtle vocal cues, like pitch and tone shifts, to better capture the emotional undertones in audio. This provides a richer listening experience, more closely aligned with the emotions intended by the speaker.
Another exciting area is cross-lingual audio processing. AI models can now utilize audio embeddings to translate audio from one language to another efficiently, making podcasts globally accessible without sacrificing the core message. This opens doors to reach a truly diverse audience and brings international content to new listeners.
Furthermore, the optimization of audio-caption synchronization is reducing cognitive overload for listeners. Research shows that precise caption alignment with spoken words leads to better comprehension and retention. This can be particularly useful in areas like education or accessibility.
Multi-language analysis often utilizes generative adversarial networks (GANs) to create high-quality synthetic audio. This opens a world of possibilities in creating diverse voice profiles for voiceovers and audiobooks, offering a wide array of potential vocal personalities.
The trend of adaptive captioning allows AI to dynamically adjust captions based on emphasis, pacing, and audience feedback. This could involve changes like font size or color to highlight key emotions or parts of the spoken content. It's intriguing to see how this approach could further enhance the audio experience.
Interestingly, models are now trained on both pristine audio and noisy environments. This makes them more resilient to real-world conditions, like live recordings or settings with background noise. This resilience should lead to improved accuracy in transcription, which can be vital in many applications.
Some systems now incorporate feedback loops that adapt based on listener interaction. For example, if a user frequently rewinds a portion of a podcast, the system can learn to prioritize clarity and engagement for that particular section.
The future of voice cloning seems to be heading towards creating unique "voice signatures" – distinct vocal profiles that can be readily recognized across different environments. This is particularly useful for maintaining brand authenticity in podcasting or audiobook marketing.
In summary, we are witnessing incredible strides in multi-language audio analysis that are profoundly changing audio creation and accessibility. While there are still areas to explore – like perfecting the synthesis of emotional nuances – it's clear that AI is playing an increasingly significant role in reshaping how we produce, consume, and interact with audio.
How Snapchat's SoundAware AI System is Revolutionizing Audio Caption Generation Using 70M YouTube Clips - Voice Cloning Technology Adapting to Individual Speech Patterns
Voice cloning technology is advancing rapidly, focusing on mimicking the unique characteristics of individual voices. AI systems, powered by deep learning, are becoming increasingly adept at capturing the subtle nuances of human speech. They analyze extensive audio datasets to learn the intricacies of tone, emotional expression, and a person's distinct way of speaking. This ability to tailor synthetic voices to specific individuals improves the quality of audio outputs, enhancing the feel of realism in areas such as podcasts or audiobooks. It allows for personalized interactions in ways not previously possible. However, alongside these technical breakthroughs, concerns about the ethical implications and authenticity of AI-generated voices remain. Moving forward, finding a balance between innovative potential and ethical considerations will be vital as we navigate this evolving field of voice cloning and its impact on how we interact through audio.
Voice cloning technology has progressed remarkably, enabling the creation of AI models that can finely tune their output to match individual speech patterns. This has led to a surge in more engaging and relatable synthetic voices, moving beyond simple text-to-speech. These systems now strive to capture the subtleties of human speech, including the expression of emotions through voice. AI models can now identify and mimic the emotional nuances within audio, conveying not just the words, but also the implied feelings, thus making the synthesized voice more impactful.
One of the exciting aspects is the growing ability to replicate incredibly detailed phonetic elements, such as the specific characteristics of accents and dialects. This improvement in capturing vocal nuances makes synthetic voices sound far more authentic and expands the applications of voice cloning to a greater diversity of languages and cultural contexts. The ability to perform real-time multi-language audio synthesis has opened up entirely new possibilities for international content production. By drawing on a wide array of audio data, these cutting-edge systems can effectively recreate specific voices in different languages.
The speed at which these models can operate in real-time has also significantly increased, now reaching up to 40 milliseconds per audio segment. This impressive speed is crucial for tasks like live podcasting and interactive narration, allowing for instantaneous feedback and modifications. A new avenue of exploration has been the development of "voice signatures", unique vocal profiles that can be easily recognized and maintained consistently across various platforms. This is particularly relevant in podcasting and audiobook production, where brand identity and voice consistency are highly valued.
Furthermore, AI systems are incorporating adaptive captioning, allowing for real-time adjustments to visual cues like font size and color based on listener interaction. This feature is especially beneficial for education or any situation where enhanced comprehension and engagement are key. The training process itself has been refined to involve exposure to both pristine and noisy environments, making voice cloning more robust and capable of dealing with the complex auditory environments we encounter in real-world settings.
It's fascinating that some systems have started using feedback loops to continuously refine their output based on how listeners interact with the content. For example, if a specific segment is replayed often, the system can learn and adapt to improve clarity or engagement within that segment. The use of generative adversarial networks (GANs) has been shown to improve the quality of synthetic voices. By learning from both actual and AI-produced audio, GANs can help generate incredibly realistic voices and even add variety to the audio landscape by introducing new and distinct vocal characteristics.
These developments in voice cloning open up many possibilities in content creation and beyond. But as these technologies advance, ethical questions surrounding the authenticity and potential for misuse of synthetic voices will become increasingly important. We're stepping into a world where it is increasingly challenging to differentiate human speech from that created by AI. As with many rapidly evolving technologies, thoughtful discussions about its implications are critical as we strive to navigate this landscape responsibly.
How Snapchat's SoundAware AI System is Revolutionizing Audio Caption Generation Using 70M YouTube Clips - Machine Learning Applications in Audiobook Production Workflows
Machine learning is transforming audiobook production, leading to greater efficiency and personalization. AI systems are now capable of automating narration and tailoring voices to specific preferences, allowing listeners to customize aspects like speaking speed and emotional tone. This level of control can create audiobook experiences that are finely tuned to individual listener preferences. Furthermore, machine learning algorithms, particularly graph-based models, can learn user preferences and content relationships to recommend audiobooks and create more personalized listening experiences.
While these advancements hold great promise for audiobook creators, significant challenges still exist. One key area is achieving a natural, nuanced expression of emotion in synthetic voices. Another hurdle is maintaining perfect synchronization between the audio and any accompanying captions or visual elements. These issues raise important questions about the overall authenticity and quality of AI-generated narratives and how listeners perceive them.
Moving forward, we can expect machine learning to play an ever-growing role in how audiobooks are produced and consumed. As these technologies mature and become more sophisticated, the landscape of audio content creation will likely continue to evolve at a rapid pace, impacting everything from the creative process to the listener experience.
Machine learning is reshaping audiobook production by enabling AI to tailor vocal characteristics to different audio contexts. For example, a system can automatically adjust tone and speed to fit the emotional landscape of a story, making the listening experience more engaging. This is a step beyond the rudimentary text-to-speech we've seen in the past.
Voice cloning is becoming more accessible, with AI systems able to replicate a person's unique voice with only a few minutes of audio. This is a powerful tool, but it's still relatively early days. The ability to personalize synthetic voices opens up a whole new world of creative opportunities, yet it also raises concerns about how easily it could be used for deception.
These AI systems are also improving in their ability to handle the diversity of human speech. They can now recognize and synthesize different accents and dialects with greater accuracy, making audiobooks more relatable to diverse audiences. It's quite interesting how the technology is starting to understand how people really speak.
Some voice synthesis systems are now being enhanced with emotion recognition algorithms. The AI can pick up on subtle cues in the audio, like if someone is feeling stressed or sad, and try to replicate that in the synthesized voice. This could lead to more emotionally resonant audiobooks and podcasts, but achieving genuine emotional authenticity with AI voices remains a challenge.
Real-time voice synthesis is becoming increasingly rapid, with some systems able to generate audio with as little as 40 milliseconds of delay. This is huge for applications like live podcasting, where real-time feedback and interaction are important. It's interesting to see how quickly these technologies are evolving.
AI systems are also becoming better at dealing with real-world audio. They can be trained with recordings that have background noise or interruptions. This ability to handle noisy audio is important if the tech wants to move beyond artificial environments. It's no longer good enough if it works only in a perfectly quiet room.
Voice cloning across languages is another intriguing area of development. AI is starting to leverage the shared aspects of human speech across languages to translate voices, essentially maintaining the core personality of the original speaker while adapting to a different language. This is a significant development for global content creation.
Researchers have shown that when synthesized audio and captions are carefully synchronized, it reduces the cognitive load for the listener. This is especially beneficial in educational audiobooks, where listeners need to process and retain a lot of information. The more seamless the experience, the better the learning.
AI systems are also learning to incorporate feedback from listeners. For example, if a section of an audiobook is frequently replayed, the AI might try to improve the clarity or engagement in that part for future listens. It's like the system is observing user behavior and tailoring itself to improve the experience.
The use of Generative Adversarial Networks (GANs) is helping to improve the realism of synthesized voices. By pitting two AI models against each other, one generating voice and the other judging its authenticity, we can create a more refined audio output. This helps AI learn and approach more naturally sounding variations of voices. The more diverse and expressive voices we can generate, the more natural our interactions with audio are going to be. It's an interesting thought.
Get amazing AI audio voiceovers made for long-form content such as podcasts, presentations and social media. (Get started for free)
More Posts from clonemyvoice.io: