Get amazing AI audio voiceovers made for long-form content such as podcasts, presentations and social media. (Get started for free)
How Natural Text-to-Speech Voices Capture Child-like Innocence A Technical Analysis of the Shy Girl Voice
How Natural Text-to-Speech Voices Capture Child-like Innocence A Technical Analysis of the Shy Girl Voice - Voice Pattern Analysis How Neural Networks Map Child Speech Frequencies
Neural networks are increasingly adept at analyzing the unique sound patterns of children's voices. By meticulously mapping the frequencies and durations of phonemes, these networks can recreate the distinctive qualities that characterize children's speech. Techniques like phoneme boundary detection and fundamental frequency modeling are key to capturing the subtle variations that distinguish child-directed speech from adult speech.
This mapping process is vital for the advancement of voice cloning and text-to-speech technologies. The ability to accurately synthesize these youthful vocal characteristics opens exciting avenues in fields like audiobook production and podcasting. For instance, neural vocoders, powered by deep learning, can transform complex acoustic features into realistic audio, allowing for the creation of artificial voices that embody the inherent innocence often associated with children's voices.
Moreover, understanding how children respond to specific speech patterns, like infant-directed speech, offers a new dimension to voice generation. Researchers can leverage these insights to better emulate the playful and engaging qualities that make these speech variations so appealing. The result is a fascinating intersection of technology and linguistic development, revealing the potential for creating more nuanced and expressive artificial voices.
Neural networks are increasingly adept at deciphering the intricacies of child speech by dissecting its frequency components. This allows for the isolation of specific phonetic features that set children's voices apart from adults. The higher fundamental frequency range, commonly between 250 and 300 Hz, is a key identifier that neural networks can exploit to fabricate convincingly child-like voices in voice cloning endeavors.
Children's shorter and more resonant vocal tracts cause formants – the resonant frequencies – to appear at different locations compared to adult voices. Capturing this distinction is essential for producing realistic synthetic voices. The capacity of deep learning models to learn from diverse voice samples improves their ability to generalize to unseen child voice data. This can substantially broaden the application of realistic synthetic speech, particularly in domains like interactive learning or games. However, capturing the rich variations in children's vocal timbral characteristics remains a challenge.
Voice cloning techniques are reliant on accurate models of vocal timbre, but children's voices frequently exhibit greater spectral complexity. This complexity makes it difficult to truly capture the unique personality traits present in each child's voice. Building these algorithms involves analyzing massive datasets of children's speech, creating sophisticated software capable of mirroring the natural inflections and pauses typical of children's conversations.
Furthermore, researchers are finding ways to distinguish emotional nuances in children's speech through machine learning techniques. This holds the potential to imbue synthesized voices with emotions such as excitement or sadness, thereby generating a more resonant connection with listeners. Children exhibit a remarkable ability to learn and produce sounds, with notable vocal changes appearing as early as six months. This early development necessitates the development of adaptive voice synthesis systems that can mirror a child’s voice through different phases of development.
Techniques such as Mel-frequency cepstral coefficients (MFCCs) play a critical role in analyzing children's voice characteristics. These techniques help us isolate the subtle phonetic features in child speech that differentiate it from adult speech. In the realm of podcast production, the ability to modify and understand these distinctive voice characteristics is proving advantageous. Podcast creators can use this knowledge to craft more engaging and relatable narratives specifically targeted towards young audiences, further highlighting the transformative potential of modern voice synthesis technologies.
How Natural Text-to-Speech Voices Capture Child-like Innocence A Technical Analysis of the Shy Girl Voice - Recording Studio Setups The Hardware Behind Child Voice Sampling
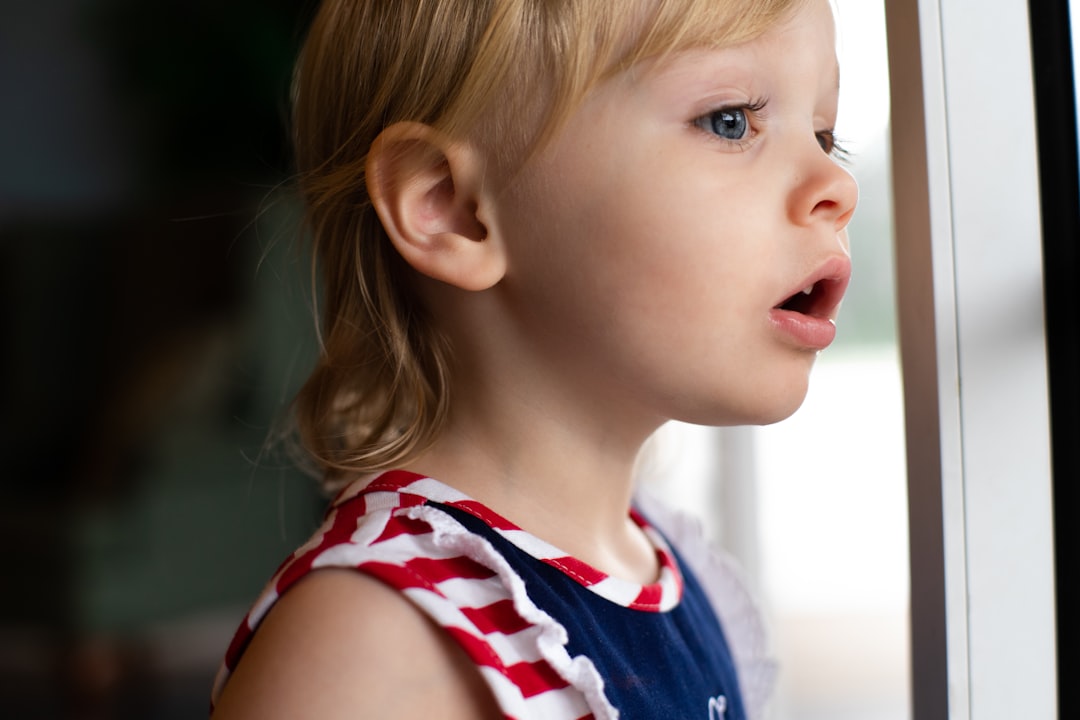
Capturing the natural and innocent qualities of a child's voice for voice cloning and text-to-speech applications demands specialized recording studio setups. The equipment used needs to be sensitive enough to pick up the nuances of children's voices, which often have a higher pitch and different formant frequencies compared to adults. High-quality microphones are essential, as they need to be able to accurately capture the delicate sounds produced by a child's vocal tract without introducing unwanted noise. Using pop filters helps reduce plosive sounds, which are common in children's speech, and improve overall audio clarity.
Creating a suitable recording environment is also vital. It should be quiet and free from distractions to ensure the recordings are clean and pristine. The recording studio should have good acoustics to prevent unwanted reflections and reverberations that can muddy the sound. Additionally, audio interfaces and editing software are necessary for post-production work, such as removing any remaining background noise, adjusting levels, and refining the overall sound quality.
These steps in the recording process are important because they help to retain the authenticity of the child's voice while removing any artifacts that might hinder the ability of voice cloning and text-to-speech systems to faithfully recreate the voice. The goal is to ensure that the resulting artificial voice sounds as natural and childlike as possible, leading to more engaging and effective applications in fields like storytelling, education, and entertainment. While the technology is still developing, these dedicated recording setups represent a crucial step towards creating convincing and emotionally resonant synthetic voices for a variety of purposes.
The creation of convincing child voices, whether for voice cloning or text-to-speech applications, relies on a precise and sophisticated recording setup. Condenser microphones, known for their ability to capture a wide range of frequencies, are often the preferred choice when recording children's voices. This is due to their sensitivity, which allows for the meticulous capture of the subtle nuances and delicate tones characteristic of young voices. Dynamic microphones, on the other hand, tend to focus on mid-range frequencies, potentially missing some of these important sonic details.
The recording environment plays a significant role in ensuring high-quality audio. Carefully designed acoustic treatments are essential for eliminating undesirable reflections and echoes that could muddle the clarity of the child's voice. Sound engineers often use techniques like bass traps and diffusion panels to create a balanced and consistent sound field. This ensures the recorded sound is faithful to the child's voice without being tainted by the surrounding room acoustics.
Children's vocal ranges often extend higher than adults, typically an octave above. Accurately capturing this higher range requires meticulous monitoring and level adjustments during recording. This is to prevent distortion and ensure that the essential qualities of the child's natural voice are preserved.
While the standard sampling rate of 44.1 kHz is sufficient for many audio applications, higher sampling rates like 96 kHz or even 192 kHz can be employed when capturing child voices. These higher rates help capture the detailed, high-frequency components of children's voices without losing valuable harmonic information. This is crucial for recreating a truly realistic child-like sound in synthesized voices.
The dynamic range of a child's voice can be substantial, spanning from gentle whispers to loud, excited exclamations. Compressing techniques, if carefully applied, can help even out these dynamic variations, resulting in a more consistent and less jarring audio experience. Applying compression too aggressively, however, can lead to a dull and lifeless sound.
In post-production, specialized voice processing techniques like formant shifting can be employed to refine the captured voice, further enhancing its child-like characteristics. This allows sound engineers to subtly adjust the spectral components of the recording, giving them finer control over the overall sonic impression.
Due to the often soft nature of children's voices, minimizing background noise is crucial. Extremely quiet recording environments are preferred to ensure that the noise floor is as low as possible. This guarantees that the delicate nuances of children's speech are preserved and remain audible above any ambient noise.
When synthesizing child voices using AI, pitch-shifting algorithms are commonly employed. However, excessive pitch shifting can introduce unnatural artifacts, leading to a robotic or distorted sound that detracts from the voice's authenticity. Sound engineers must use these tools judiciously to maintain a sense of naturalness.
Recording a child's voice using binaural microphones can provide a more immersive and natural listening experience. This technique captures the sound field more realistically, creating the illusion of three-dimensional space. This can contribute significantly to the authenticity and engagement of the synthesized voice.
Real-time feedback mechanisms, such as pitch correction tools, are valuable in both recording and synthetic environments. They provide a way for sound engineers to dynamically adjust the pitch and other characteristics of the voice in real time. This allows for the immediate correction of errors or for capturing spontaneous emotional nuances as they arise in a child's vocalizations. This level of interactive control enhances the accuracy and naturalness of the final synthesized voice.
The ongoing development of these recording and processing techniques, particularly in conjunction with advancements in artificial intelligence, promises ever more realistic and captivating child voices for various applications like audiobook production and voice cloning. The ability to recreate the essence of a child's voice carries with it a responsibility to ensure that these tools are used in ways that are both ethical and beneficial.
How Natural Text-to-Speech Voices Capture Child-like Innocence A Technical Analysis of the Shy Girl Voice - Emotional Mapping in Voice AI Why Children Sound Different Than Adults
In the realm of voice AI, understanding how emotions are expressed through sound – what we call emotional mapping – is becoming increasingly important. Children's voices, with their characteristically high pitch and complex sound profiles, pose a particular challenge when trying to create natural-sounding and emotionally rich text-to-speech (TTS) voices. This isn't simply due to the physical differences in their vocal structures compared to adults; the way children develop emotionally and perceive emotions also plays a big role in how they use their voices.
The goal of the latest AI systems is to capture and reproduce these subtleties in children's vocal expression. This could make interactions in areas like audiobook narration and podcasting much richer and more engaging. The quest for realistic emotional expression in AI-generated voices is tough. It requires researchers to continue refining their techniques to truly capture the full spectrum of emotional expression that naturally occurs in children's voices. It's still an open question how accurately and convincingly AI can convey these emotional nuances.
The field of voice AI has made significant strides in generating natural-sounding speech, but accurately capturing the unique qualities of children's voices remains a challenge. Initially, the focus was on making speech understandable, but now, the goal is to achieve naturalness and even emotional expression. While some progress has been made, achieving fine-grained control over emotional nuances in synthesized speech remains an area of active research.
Children's vocal tracts are physically different from adults', resulting in distinct formant frequencies. These formants, essentially resonant frequencies, impact how the sounds of speech are shaped. Consequently, recreating realistic child-like voices requires careful modeling of these unique formant shifts. Also important is the higher fundamental frequency range in children, usually between 250 and 300 Hz, which distinguishes them from adults. Neural networks and machine learning models are being used to analyze these features and translate them into synthetic voices.
However, a child's voice isn't just about pitch. The spectral complexity of children's voices – the richness of their overtones and harmonics – adds another layer of difficulty for voice cloning. It's more than just mimicking a simple tone; it's about capturing the individual personality that each voice embodies.
Adding another layer of complexity is that children's voices change as they grow. The developmental trajectory of a child's vocal ability means any AI model must be adaptable. It's not just about pitch shifts, it also involves the ongoing maturation of emotional expression. An AI voice needs to reflect these changes accurately if it's to capture the essence of a child's evolving voice.
Several techniques are being used to better model these complex vocal characteristics. Mel-frequency cepstral coefficients (MFCCs), for instance, have become standard tools for analyzing speech. They're used to isolate those fine phonetic details that vary across ages. These are valuable for creating more accurate synthetic voices, especially as children's language skills develop.
Another challenge is the recording environment. Sound engineers work hard to create spaces that minimize reflections and ensure a clean audio recording. Sound can bounce around a room in unpredictable ways, and for recording subtle vocal details from a child, the environment matters a great deal. It's particularly critical for capturing the dynamic range of a child's voice, from the quietest whispers to the loudest excited bursts of sound. Synthesized voices need to be able to represent these dynamic variations to feel natural.
There's also experimentation with binaural recording techniques, which attempt to replicate the natural spatial experience of sound. This can improve the perceived naturalness of synthetic voices, enhancing the listening experience, especially for content aimed at children.
Interestingly, children's speech production undergoes rapid change in the first few months of life, with significant improvements in the clarity of sounds by around six months old. These early developmental steps challenge researchers to create models that can capture these rapid changes in speech patterns.
Finally, there's a growing reliance on real-time feedback tools during recording. These tools allow for instant adjustments to pitch and tone, allowing engineers to capture the spontaneity and nuanced emotional variations present in children's speech. This helps ensure a high level of authenticity in the synthetically generated voices.
While advancements in voice AI continue, replicating the full range of expressive human qualities, especially in dynamic situations, remains a formidable challenge. Capturing the rich and constantly evolving nature of children's voices is particularly demanding but promises exciting potential for the future of applications like audiobook production, voice cloning, and interactive storytelling.
How Natural Text-to-Speech Voices Capture Child-like Innocence A Technical Analysis of the Shy Girl Voice - Speech Rate Variations Natural Pauses in Child Voice Production
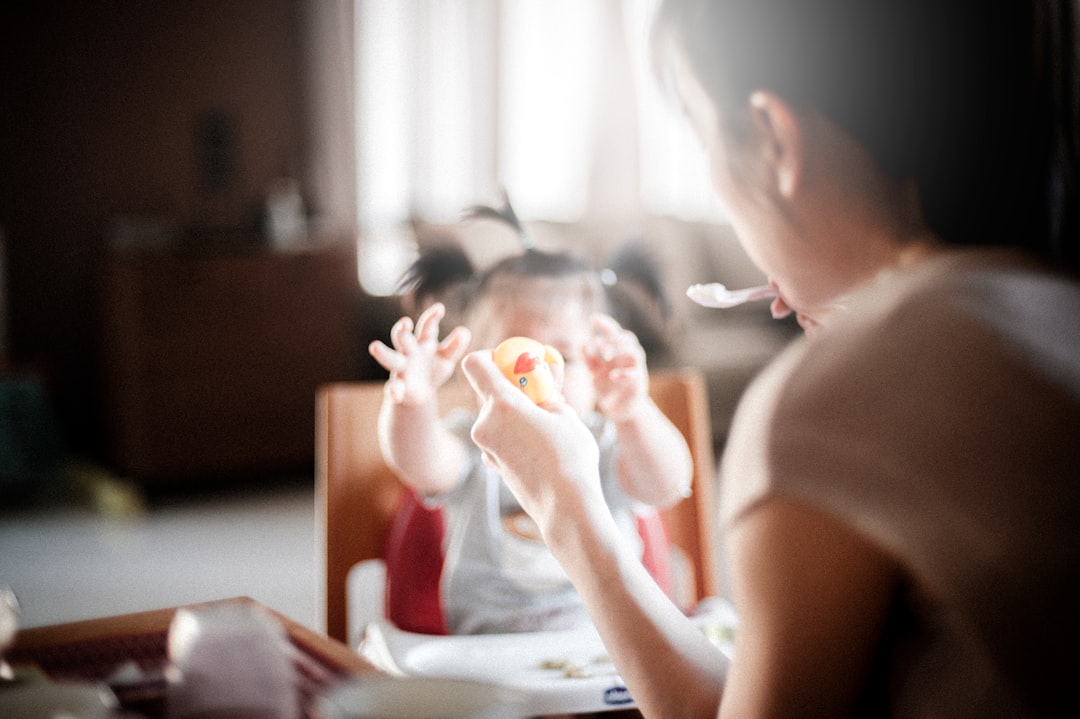
When exploring how children speak, a significant aspect is the way they vary their speaking speed and naturally pause. These elements are crucial for making synthesized voices sound authentic. Kids naturally change how fast they talk, often with sudden pauses that show their emotions or what they're talking about. These shifts make a child's voice more expressive, but they also create challenges for the technologies that try to copy voices. To capture these subtleties, complex algorithms are needed to analyze and replicate the unique characteristics of how children speak. The goal is to make sure synthetic voices have the innocence and spontaneity that children's voices are known for. Ongoing research in neural text-to-speech aims to develop systems that not just imitate the sounds of children but also capture the captivating and nuanced aspects of how they naturally communicate. While there have been advancements in mimicking the sound of children, there are still obstacles when it comes to completely replicating the emotional nuances and natural patterns of child speech. This field is continually evolving, and as it does, we can expect to see more advanced techniques emerge, allowing for more compelling and lifelike child voice synthesis.
The clarity of children's speech significantly improves by around six months of age, highlighting the need for age-specific recording methods in audio capture. Synthetic voice models need to adapt to these developmental changes if they're to produce truly accurate representations. Failing to account for this rapid developmental progression in speech could lead to inaccurate and less-than-natural sounding synthetic voices.
Children's voices possess a broad dynamic range, encompassing both hushed whispers and excited shouts. To create convincingly authentic synthetic voices, recording techniques must meticulously capture both ends of this spectrum. If the full dynamic range isn't adequately captured, the resulting synthetic voices might lack the genuine emotional depth that makes them relatable.
The link between a child's emotional state and their vocal characteristics is complex. Things like pitch and tone of voice shift with a child's feelings. This intricate relationship poses a significant challenge for AI researchers seeking to replicate genuine emotional expressions in synthesized voices. It's a tough problem that requires continued refinement of modeling techniques to capture the full range of emotions that naturally appear in children's voices.
The recording environment greatly impacts the quality of a child's voice capture. Poor acoustics can introduce unwanted reflections and echoes, muddling the finer details in their speech. These reflections can pose a serious hurdle for efforts to accurately clone children's voices. The resulting AI voice might not represent the nuances of their speech as intended.
Because a child's voice changes with age, AI voice models must exhibit flexibility and adaptability. It's not enough to simply adjust pitch. The evolving patterns of emotional expression during a child's growth also need to be considered. AI models that fail to accommodate these evolving dynamics produce static voice profiles that don't connect with listeners over time.
While neural networks can analyze frequency patterns with great accuracy, capturing the intricate complexity of children's voices continues to be a challenge. These networks sometimes struggle with the delicate overtones that give each child's voice a unique identity. This inherent limitation requires researchers to find alternative or complementary techniques to ensure the synthesized voices are truly unique.
Mel-frequency cepstral coefficients (MFCCs) play a critical role in recognizing and isolating subtle phonetic details present in children's voices. By pinpointing these features, they significantly enhance the modeling process for generating synthesized speech. MFCCs ultimately contribute to making the resulting synthetic voice more relatable to a child audience.
Binaural recording techniques mimic human hearing, providing a three-dimensional sound experience. When applied to recordings of children's voices, it enhances the perceived authenticity of the synthesized output. This approach helps create a more immersive auditory experience that makes children feel more engaged and connected with the AI-generated content.
Real-time feedback systems are incredibly helpful for capturing spontaneous emotional nuances in children's speech. This level of real-time control can be challenging to replicate after the recording has finished. Therefore, the immediacy of real-time feedback improves the overall naturalness and authenticity of synthesized child voices.
High-quality condenser microphones are favored for recording children's voices because of their ability to pick up higher frequencies. This sensitivity to higher frequencies ensures that the clarity and vibrancy of a child's vocal output is preserved during the synthesis process. This careful choice of microphone is necessary to maintain the essential characteristics of their speech that distinguish their voices from adults.
How Natural Text-to-Speech Voices Capture Child-like Innocence A Technical Analysis of the Shy Girl Voice - Pitch Control Methods Creating Age Specific Voice Authenticity
Controlling pitch is a key aspect of creating realistic, age-specific voices in audio, especially for children. Modern text-to-speech (TTS) systems are becoming quite good at fine-tuning the pitch of synthesized voices. This lets them accurately reproduce the higher-pitched nature of children's voices. This is important for maintaining the playful and emotional qualities naturally expressed in younger voices. Techniques such as Mel-frequency cepstral coefficients (MFCCs) help analyze and identify subtle details in children's voices. Real-time feedback during recording further helps capture the dynamic range of these voices, making for more natural-sounding recordings. Despite progress, there's still work to be done to capture all the emotional complexities of children's voices to make them truly sound like human children. This involves a constant back-and-forth between technology and the human artistic element in audio production. Ideally, this will lead to more engaging audio experiences in podcasts, audiobooks, and voice cloning applications.
The creation of convincingly child-like voices in synthetic speech technologies hinges on understanding and replicating the unique acoustic properties of children's vocal tracts. The smaller size of a child's vocal tract compared to an adult's leads to distinct formant frequencies, which are crucial for shaping the sound of speech. Accurately modeling these formant shifts is essential for crafting realistic artificial voices.
Beyond the physical differences, children's voices also possess a greater spectral complexity compared to adult voices. The multitude of overtones and harmonics that contribute to their speech are crucial for capturing the individual characteristics of a child's voice when cloning it. This intricate interplay of frequencies is a challenge for voice cloning systems, making the reproduction of truly individualistic and natural-sounding child voices difficult.
Current recording technologies are improving to capture the subtleties of a child's emotional expression through speech. Implementing real-time feedback during recording allows sound engineers to make adjustments to pitch and other vocal aspects on the fly. This immediate modification capability allows for the dynamic incorporation of emotional nuances, resulting in synthesized voices with a higher degree of authenticity.
Children's voices exhibit a broad dynamic range. From hushed whispers to excited exclamations, the full range must be captured. Neglecting to capture the entire range can result in synthetic voices that are emotionally flat. This dynamic capture is crucial for achieving the emotional depth and resonance we associate with children's voices.
The rapid developmental changes that occur in a child's speech, particularly within the first few years of life, pose a formidable challenge for voice synthesis systems. Maintaining accuracy across these developmental stages necessitates continuously adapting the speech models to ensure they remain effective. Failing to adapt could lead to producing a static and inaccurate depiction of a child's voice.
Creating a suitable recording environment is critical for the success of any voice cloning effort. Unwanted echoes or reverberations from a poorly designed studio space can muddle the finer details in a child's voice, potentially hindering the accuracy of the cloning process. The result can be less-than-engaging synthetic voices.
Mel-frequency cepstral coefficients (MFCCs) have proven to be invaluable in the analysis of children's speech. MFCCs excel at extracting those subtle phonetic variations that uniquely characterize children's voices. Leveraging MFCCs enhances the accuracy of synthetic voice generation, contributing to a more believable and relatable artificial voice, especially when targeted at children.
Children exhibit spontaneous, often unpredictable interruptions in their speech. Capturing these natural breaks and pauses is critical for crafting a realistic and engaging synthesized voice. Simply replicating the sounds of a child's voice isn't sufficient; the overall speech patterns must be mirrored to convey authenticity.
Using binaural microphones provides a compelling auditory experience when recreating a child's voice. This recording method more closely emulates human hearing, leading to a more immersive and realistic listening experience. For content aimed at children, the ability to create a sense of three-dimensional sound adds to the sense of engagement.
The importance of tailoring voice cloning to specific ages cannot be overstated. Children's voices evolve dramatically during development, demanding constant updates to voice models to stay effective. Failing to consider this evolution will result in voice clones that do not resonate over time with their intended audience.
In conclusion, creating genuinely convincing synthetic child voices remains a captivating but challenging endeavor. These complex aspects of speech production, coupled with the rapid developmental changes that occur in children, present ongoing challenges for researchers in this domain. However, as these technologies mature, we can anticipate further improvements in the capacity of AI to not only produce speech that sounds like a child but also to capture the unique emotional depth and nuanced expressions of these fascinating human voices.
How Natural Text-to-Speech Voices Capture Child-like Innocence A Technical Analysis of the Shy Girl Voice - Audio Post Production Steps Converting Raw Voice Data to Natural Speech
The journey from raw voice recordings to natural-sounding speech, especially when aiming for child-like qualities, heavily relies on audio post-production techniques. Initially, the raw audio undergoes a detailed examination of the text content to understand the underlying structure and meaning. This process transforms the written text into a format suitable for speech synthesis, which involves converting it into speech features.
Sophisticated techniques like Mel-frequency cepstral coefficients (MFCCs) are crucial in this stage, analyzing the intricate phonetic details of children's voices to isolate their distinctive qualities. These techniques help create accurate models of how children's vocal tracts shape sounds.
To capture the inherent emotional expression of children, post-production also leverages real-time feedback during recording. This allows for immediate adjustments to pitch and other vocal qualities, helping to replicate the wide range of emotions children display in their voices. This is particularly important in creating an authentic sound that replicates the unique way children naturally vary their speech rate and pauses.
As artificial intelligence and audio processing techniques continue to improve, these post-production methods will contribute to a more realistic experience in applications like audiobooks and podcasting. The ultimate goal is to create synthetic voices that not only sound like children but also convey the engaging, natural communication style we associate with their voices. This is particularly important for creating a meaningful and engaging experience for children, both in entertainment and educational settings. While the field of voice cloning and AI-generated speech continues to advance, achieving perfectly natural-sounding children's voices remains a challenging, and perhaps never fully achieved, endeavor.
1. **The Challenge of Dynamic Range:** Children's voices cover a wide range of volume, from quiet whispers to loud exclamations. Accurately capturing this full range is critical. If not properly managed, synthetic voices might sound emotionally flat and lack the natural depth we associate with children.
2. **Formant Frequencies and Vocal Tract Size:** The smaller size of a child's vocal tract results in unique formant frequencies—the resonant frequencies that shape the sound of speech—compared to adults. Modeling these differences is essential for creating convincingly age-appropriate synthetic voices.
3. **Capturing Emotional Nuances in Real-Time:** Modern audio production relies on real-time feedback during recordings. This allows sound engineers to instantly adjust the pitch and other vocal elements, capturing the spontaneous emotional changes that occur naturally in a child's voice.
4. **Spectral Complexity and Individuality:** The richness of a child's voice, with its many overtones and harmonics, makes it spectrally complex. This makes it challenging for voice cloning systems to create truly authentic voices, as it's not just about sound, but also about individual personality. Analyzing the specific details of each voice is crucial.
5. **Pitch Shifting – A Double-Edged Sword:** Current TTS systems are better at adjusting the pitch of a synthesized voice, crucial for mimicking the naturally higher pitch of children. However, too much manipulation can lead to an unnatural, robotic sound that undermines authenticity.
6. **The Importance of Mel-Frequency Cepstral Coefficients:** This technique is invaluable for isolating the tiny differences in how children pronounce sounds. These subtle features are essential for improving the models used to create synthesized speech, especially when designing content specifically for children.
7. **The Ever-Changing Child's Voice:** Children's voices change rapidly, especially in early childhood. AI voice models need to adapt constantly to reflect these changes to be effective. Failing to do this can result in a voice that sounds artificial and doesn't resonate with listeners as the child ages.
8. **Binaural Recording for Enhanced Immersion:** Using binaural microphones mimics the way humans hear, making the recorded sound more realistic and three-dimensional. This is particularly useful for children's content, creating a more engaging experience.
9. **The Role of Pauses and Natural Speech Patterns:** Children naturally pause during speech. Capturing these pauses is vital for creating believable synthetic voices. Simply imitating a child's sounds isn't enough; their overall conversational patterns must be considered for authentic-sounding results.
10. **Controlling the Recording Environment:** The acoustics of the recording studio are crucial. Unwanted echoes and reflections can obscure the details of a child's voice, making it more difficult to create accurate voice clones. A carefully treated space ensures a cleaner, more precise recording for optimal results.
Get amazing AI audio voiceovers made for long-form content such as podcasts, presentations and social media. (Get started for free)
More Posts from clonemyvoice.io: