How IPA Notation Enhances Voice Cloning Accuracy A Technical Deep-Dive
How IPA Notation Enhances Voice Cloning Accuracy A Technical Deep-Dive - How IPA Transcription Maps Natural Speech Patterns in Neural Networks
The International Phonetic Alphabet (IPA) plays a crucial role in bridging the gap between human speech and neural networks. It acts as a bridge, translating the complexities of spoken language into a format that neural networks can readily process and understand. This translation is vital because human speech perception is a remarkably complex process involving intricate calculations that transform sound waves into meaningful linguistic units. This process engages the entire auditory system, from the initial detection of sound to the higher-level cognitive processing of language.
IPA's ability to capture these fine-grained details of speech empowers neural networks to effectively decode spoken language. We're seeing the benefits of this in areas like voice cloning, where the ability to pinpoint precise phonetic segments is critical for accurate and natural-sounding voice reproductions. Tools like the Mason-Alberta Phonetic Segmenter, a neural network-based system, highlight the potential for automation in analyzing speech and extracting key phonetic features.
However, automatically converting audio into IPA remains a challenging task. Speech is inherently variable, influenced by individual speaking styles, accents, and background noise. Overcoming these obstacles through continued research and development is essential for improving the quality of voice cloning and other speech-related applications. Deep learning methods are making significant strides, promising to refine the accuracy and efficiency of voice cloning and audio production in the future.
The International Phonetic Alphabet (IPA) provides a remarkably detailed system for representing sounds, with over 1,100 distinct symbols. This level of detail is crucial for training neural networks to replicate the intricacies of human speech more accurately. By capturing the subtle variations in sound production, IPA allows neural networks to better grasp the complex interplay of sounds within a word or phrase, a phenomenon known as coarticulation. This leads to a smoother, more natural-sounding voice output, moving away from the robotic quality often associated with earlier speech synthesis models.
Researchers are increasingly finding that the shape of the vocal tract profoundly affects the sound we produce. With the IPA as a guide, voice cloning engineers can effectively translate these vocal tract configurations into parameters that guide the neural networks. This results in a more nuanced simulation of human speech during the cloning process, leading to potentially higher fidelity voice clones.
Furthermore, IPA's ability to capture specific pitch contours offers a valuable tool for mimicking the emotional nuances in human speech. By embedding this information within the neural network's training data, developers can strive to create voice clones that are more expressive, conveying not just the words but also the emotional tone of the original speaker. This is particularly important for applications like audiobook narration or character voice design in podcasts.
We've also seen that IPA's emphasis on features like stress and intonation helps achieve more dynamic and versatile voice cloning outputs. Think of it as providing a detailed roadmap of pronunciation variations that enhance expressiveness in speech. For example, if we're aiming for a voice clone with a distinct regional accent or dialect, using IPA as the training foundation allows the system to learn and accurately reproduce these subtle phonetic features.
One significant advantage of training voice cloning models with IPA transcription is the potential for more efficient use of data. Because each IPA symbol represents a very specific sound, the network can generalize from a smaller dataset and extrapolate with greater confidence to unseen examples. This can be particularly valuable when dealing with less common languages or accents, where the availability of labelled data might be a limiting factor.
Additionally, the inherent precision of IPA seems to lead to cleaner, less distorted speech output in voice cloning systems. The rich detail helps the model generate a more accurate representation of the intended sound, leading to a decrease in artifacts and an increase in intelligibility.
Finally, many believe that the application of IPA transcription to voice cloning systems can be beneficial in real-time scenarios, such as in virtual assistant technologies. The meticulous nature of the phonetic symbols allows the model to quickly adapt to varying user inputs, improving the user experience and making the system more responsive and personalized. This responsiveness, rooted in the clarity of IPA, offers a pathway towards more intuitive and effective voice interactions.
How IPA Notation Enhances Voice Cloning Accuracy A Technical Deep-Dive - Breaking Down Phoneme Recognition Through IPA Documentation
Delving into phoneme recognition using IPA documentation reveals how the International Phonetic Alphabet plays a crucial role in accurately representing and analyzing speech sounds. This system is essential for voice-related technologies because it offers a consistent and universally understood way to describe the sounds of spoken languages, encompassing a wide range of dialects and accents. IPA's detail is vital for voice cloning, where capturing the intricate variations in pronunciation is key to producing realistic and natural-sounding voice reproductions. This enhanced accuracy is beneficial for various applications like audiobook production or crafting characters with distinctive voices in podcasting.
While the automated conversion of audio into IPA remains a challenge due to the inherent variability of speech, ongoing advancements in self-supervised machine learning models, like those used in speech recognition, are progressively improving phoneme identification within the IPA framework. IPA's structured representation of sounds empowers these models to learn and decipher phonetic intricacies more effectively. Further, voice actors find it invaluable for precise control over pronunciation, helping them achieve a higher degree of professionalism in their recordings. This heightened level of articulation directly translates to an enhanced listening experience. The combination of IPA's systematic nature and ongoing technological advancements will continue to open up new possibilities in the areas of voice cloning and related applications.
The International Phonetic Alphabet (IPA) offers a remarkably detailed system for representing sounds, exceeding the capabilities of standard alphabets. This level of detail is particularly valuable for voice cloning technologies, as it allows for the capture of subtle pronunciation nuances that are crucial for achieving a truly authentic sound. This precision is evident in differentiating similar sounds, like the English vowels in "beat" and "bit," which standard orthography might not adequately distinguish.
Because the IPA serves as a universal phonetic language, researchers and engineers worldwide can collaborate effectively on voice cloning projects, minimizing potential communication barriers arising from regional language variations. Furthermore, its ability to capture dialectal variations is key for voice cloning applications that aim to accurately replicate regional accents. This feature is essential for ensuring authentic speech production within different cultural contexts, enhancing the immersive nature of audiobooks or podcasts, for instance.
IPA’s utility extends beyond just capturing sounds; it provides tools for annotating prosodic features such as stress patterns and intonation. Integrating this type of data into voice cloning systems allows for the production of outputs that not only sound correct but also convey the intended emotional context. This is a particularly crucial aspect for applications in audiobook narration or character voice design in podcasts, where conveying emotion is integral to engagement and immersion.
Interestingly, integrating IPA transcriptions into neural network training has revealed that these AI systems demonstrate enhanced generalization across diverse voices. This improved ability to handle variations and produce coherent outputs likely stems from the structured approach that IPA offers for understanding speech. This structured approach helps neural networks to grasp the complex interactions of sounds, such as coarticulation, where adjacent sounds influence each other’s production.
Moreover, the detailed nature of IPA symbols allows voice cloning models to perform well even when training data is limited. This is particularly beneficial for languages or accents with a relatively smaller amount of readily available data. Notably, IPA allows for documenting unique speech sounds across languages, including clicks in certain African languages. By harnessing this detail, voice cloning can push the boundaries of sound replication by capturing and reproducing these highly distinct and culturally significant vocal traits.
Beyond individual sounds, IPA can also capture temporal dynamics like timing and rhythm. This feature is vital for generating natural-sounding voice clones that maintain authentic speech rhythms, significantly impacting listener comfort and relatability. However, despite its significant advantages, automatically converting speech into IPA still faces substantial technical challenges. Overlapping speech, background noise, and variations in speaking styles pose significant hurdles for engineers developing the recognition algorithms necessary to fully leverage IPA's potential in voice cloning technologies. Ongoing research and innovation are crucial for overcoming these hurdles and maximizing the capabilities of IPA in the future of voice cloning.
How IPA Notation Enhances Voice Cloning Accuracy A Technical Deep-Dive - Accurate Voice Replication Through IPA Stress Pattern Analysis
Achieving truly accurate voice replication demands a deep understanding of how stress patterns shape speech. The International Phonetic Alphabet (IPA) provides a powerful tool for analyzing these stress patterns, allowing voice cloning systems to capture the nuances of a speaker's intonation and emotional delivery with greater precision. By meticulously analyzing stress, voice cloning technologies can produce synthetic speech that sounds remarkably natural, mimicking the subtle rises and falls of a speaker's voice. This level of accuracy is particularly valuable for applications like audiobook narration and character voice design in podcasts, where conveying emotions is key to listener engagement.
IPA's role is vital because it offers a structured way to represent both phonetic sounds and prosodic features like stress and intonation. This framework enables voice cloning systems to capture the intricate interplay of these elements, leading to more realistic and compelling voice outputs. However, achieving this level of fidelity in real-time settings presents significant obstacles in speech recognition. Despite these hurdles, ongoing research into stress pattern analysis within the IPA framework holds tremendous promise for pushing the boundaries of voice synthesis and generating increasingly lifelike and versatile synthetic voices in the future.
The International Phonetic Alphabet (IPA) offers a remarkably detailed system for representing sounds, far exceeding the capabilities of standard writing systems. This breadth of phonetic detail is crucial for voice cloning, as it allows us to capture subtle pronunciation nuances that are fundamental to achieving a truly authentic-sounding reproduction. We're talking about capturing the fine distinctions between, for example, the English vowels in "beat" and "bit", where standard spelling doesn't always provide enough clarity.
It's becoming increasingly clear that the shape of a person's vocal tract significantly influences the sound they produce. Using IPA to model these vocal tract configurations helps us build more accurate simulations in voice cloning, potentially leading to more lifelike recreations of the original speaker's voice. This kind of accuracy is particularly important when you consider how nuanced human speech is.
Beyond capturing basic phonemes, IPA also includes tools for annotating prosodic features, such as stress and intonation patterns, which are critical for conveying emotional subtleties in speech. This opens up exciting possibilities for audiobook production, where conveying emotions alongside the story is essential for audience engagement. Similarly, creating characters with distinct emotional traits in podcasting could greatly benefit from these capabilities.
IPA notation includes explicit markers for stress and intonation. This framework can significantly enhance the capabilities of voice cloning systems. By leveraging these markers, we can produce more dynamic speech, potentially enabling the replication of regional accents or dialects with remarkable precision. This has obvious implications for applications where a specific regional flavour or dialect is desired, such as when creating voice clones of certain historical figures or in creating voiceovers for diverse audiences.
One of the interesting aspects of IPA is how it allows voice cloning models to generalize from smaller datasets more effectively. This is because each IPA symbol represents a highly specific sound, enabling the network to grasp the underlying patterns with greater ease and confidence. This attribute is especially valuable for less common languages or regional accents where the availability of labelled data can be a significant constraint. This could even benefit efforts to archive and preserve endangered languages.
When voice cloning systems are trained with IPA transcriptions, they often produce cleaner and less distorted audio outputs. This stems from the detailed nature of IPA, which helps minimize those often-annoying synthetic artifacts that can diminish intelligibility. We get a higher quality, smoother reproduction, and that’s significant for creating listening experiences that are both pleasurable and clear.
Leveraging IPA in voice cloning allows models to quickly adapt to changes in user input. This enhanced adaptability significantly improves the user experience for applications like virtual assistants. This kind of adaptability could be a powerful element for creating more natural, interactive and responsive virtual interfaces in the future.
The universal nature of IPA can help improve collaboration among researchers worldwide. This means we can potentially minimize communication barriers in multi-regional projects. This collaborative potential could significantly accelerate the development of voice cloning technologies tailored to diverse linguistic contexts.
IPA's comprehensive nature extends to documenting unique phonetic features that are not found in the majority of the world's languages, such as click sounds in certain African languages. This allows voice cloning technology to expand its reach by capturing a far wider array of culturally significant sounds.
While IPA is remarkably useful, automatically converting speech into IPA remains a significant technical hurdle. Speech is incredibly variable, affected by individual speaking styles, background noise, and overlapping speech. The variability makes the process of automatically translating speech to IPA challenging. Ongoing research and innovation are essential for refining algorithms and addressing these challenges to unlock the full potential of IPA in future voice cloning systems.
How IPA Notation Enhances Voice Cloning Accuracy A Technical Deep-Dive - IPA Based Language Models for Cross Cultural Voice Adaptation
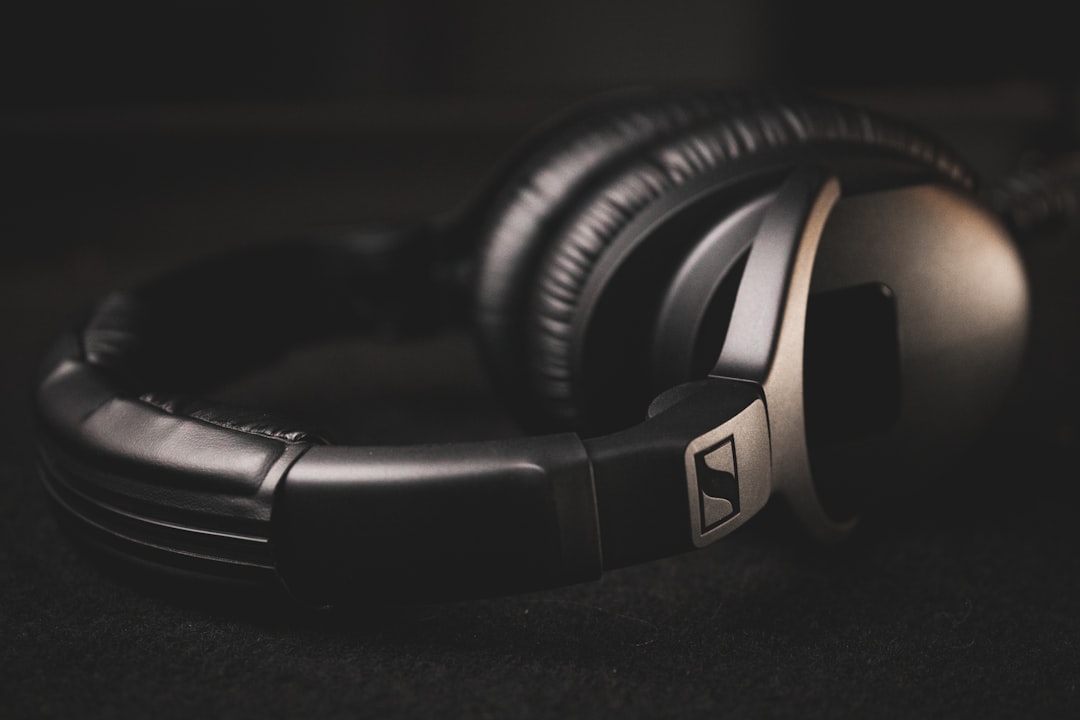
The use of IPA-based language models is becoming increasingly important in the realm of voice cloning, particularly for adapting voices across cultures. These models effectively capture the intricate details of pronunciation across different languages and dialects, resulting in significantly improved accuracy and naturalness when cloning voices. The International Phonetic Alphabet's detailed phonetic structure is key to representing these nuances, allowing for more authentic voice reproductions.
However, there are limitations. Building robust models often requires diverse and comprehensive training data. Simply using datasets with only one speaker per language might not adequately capture the diverse range of pronunciations needed for effective cross-cultural adaptation. Furthermore, the inclusion of suprasegmental features, like stress and intonation patterns, is vital to accurately replicate the emotional nuances present in human speech. This is especially important for applications where emotionality is central to the experience, like audiobooks and character voices in podcasts.
The way we process and incorporate IPA within these language models still requires further development. More refined methodologies are needed to fully realize the potential of IPA and optimize cross-cultural voice interactions. Continued research and improvements in this area are crucial for enhancing the quality and utility of cross-cultural voice adaptation technologies.
The International Phonetic Alphabet (IPA) has become a cornerstone in cross-cultural voice cloning (CVC) within text-to-speech (TTS) systems, acting as a universal language for sound representation. While its importance is recognized, research often underestimates its full potential in cross-lingual TTS applications. Interestingly, how we process IPA and suprasegmental information within these models appears to have a limited effect on CVC performance.
Creating effective CVC models with only one speaker per language in the training data seems insufficient. We're finding that having a diverse set of speakers is key to generalizing well. In the heart of a cross-lingual voice cloning system, the tone color converter leverages the IPA as a core phoneme dictionary, significantly improving performance during training. The power of IPA is also evident in multilingual connectionist temporal classification (CTC) systems, as it allows them to easily expand their output layers to encompass new languages, essentially creating a more universal phoneme set.
Adapting TTS models for new voices or languages can be surprisingly efficient. Small changes to models like Tacotron can lead to good results, even with as little as 20 minutes of new audio data. Achieving effective cross-cultural voice adaptation requires a careful disentanglement of speaker characteristics from the linguistic features within the TTS models themselves. This is an ongoing challenge, but one crucial for success.
Researchers are increasingly recognizing that we need better ways to integrate IPA information into TTS frameworks if we're to improve cross-lingual voice cloning. Current approaches might be missing out on the full potential of IPA's details. The multilingual acoustic models built with CTC architectures have a unique advantage in that they can extend to new languages quite efficiently with the support of IPA. This ability to generalize is exciting for pushing the boundaries of language coverage in voice cloning applications like audiobook production or voice acting in podcasts. While these CTC-based models show promise, it's important to remember that the ultimate goal is a highly accurate voice clone that doesn't simply sound intelligible, but also convincingly captures the unique characteristics of the target speaker and maintains the natural nuances of language. Continued exploration and refinement of methods for leveraging IPA is key to unlocking its potential for making realistic and natural-sounding voice clones that are truly capable of bridging cultural and linguistic divides.
How IPA Notation Enhances Voice Cloning Accuracy A Technical Deep-Dive - Technical Standards in IPA Based Voice Sample Collection
Within the field of voice cloning, establishing technical standards for collecting voice samples using the International Phonetic Alphabet (IPA) is paramount for achieving high-quality, natural-sounding results. IPA's detailed phonetic system allows for capturing subtle speech nuances, but current voice sample collection methods lack standardized procedures. This absence of standardization creates inconsistencies that affect data reliability and hinder its usefulness for AI research. The issue is compounded by the fact that different recording devices introduce varying levels of background noise and inconsistencies in audio characteristics, like base frequency, into the samples. To truly optimize voice cloning, researchers and developers need to implement strict guidelines for collecting and handling voice samples. These guidelines should ensure the samples are phonetically balanced and capture the diversity of human speech patterns. Improvements in these technical standards will not only refine the accuracy and realism of synthetic voices but also enhance the overall listening experience in applications such as audiobook narration and podcast production. The challenge remains in defining these standards broadly enough to allow diverse speech patterns while remaining focused enough to ensure consistent high quality in the results.
The International Phonetic Alphabet (IPA) offers a detailed system for representing sounds, but its nuances, especially regarding voice production, are often underappreciated in voice cloning. For instance, the impact of nasality on vocal characteristics, as captured through IPA's diacritical marks, can significantly affect timbre and speech intelligibility, posing a challenge for accurate replication. Additionally, IPA's representation of vowel length, which can alter word meaning in some languages, highlights the importance of capturing these subtle dynamics for generating contextually appropriate speech in applications like audiobooks.
Furthermore, the coarticulation effect, where sounds influence each other during articulation, is crucial for natural speech. Leveraging this within IPA frameworks is essential for voice cloning models to avoid producing the artificial, robotic quality often associated with early synthetic speech. Consonant clusters, especially in languages with complex sound combinations, also present a hurdle. Successfully training models to smoothly handle these clusters is crucial for a more natural flow in the voice clone's output.
The efficiency of IPA-based phoneme recognition allows for impressive real-time adaptations, particularly relevant for user-facing applications like virtual assistants. However, the relationship between intonation and emotion, both captured within IPA, is often blurred. Effective voice cloning requires carefully distinguishing these aspects during training to produce outputs that reflect not only the words but also the emotional intent of the original speaker.
IPA’s comprehensiveness extends to dialectal variations, making it a powerful tool for creating language learning resources. For example, engineers could utilize IPA to generate training datasets for specific dialects, aiding educational audio content designed for learners of varied accents and pronunciations. Yet, with over 1,100 symbols, IPA can be overwhelming. Successfully training voice cloning models has involved prioritizing common symbols, streamlining the synthesis process and optimizing resource use.
Beyond basic sounds, IPA also represents temporal dynamics, or how sounds change over time, factors like speech rate, pauses, and other timing elements. Incorporating this dynamic representation within voice cloning models generates speech that resonates with listeners more naturally. While IPA’s universality is beneficial, adapting it to various linguistic contexts, particularly tonal languages, presents challenges. Developing techniques to accurately represent pitch variations essential for meaning in tonal languages remains an active area of research within the field of voice cloning.
How IPA Notation Enhances Voice Cloning Accuracy A Technical Deep-Dive - Machine Learning Speech Recognition With IPA Reference Data
Machine learning's application to speech recognition is seeing significant advancements, particularly with the incorporation of the International Phonetic Alphabet (IPA) as a foundational element in training datasets. This use of IPA empowers algorithms to more effectively analyze and replicate the intricate details of human speech, including subtle variations in pronunciation and the melodic aspects of speech like intonation and stress. This heightened focus on phonetics is not only fostering the development of more precise voice cloning techniques but also directly addressing the challenges that come from the inherent variability in how individuals speak, as well as the environmental factors that influence the quality of audio recordings. The anticipated outcome of these improvements in speech recognition is the creation of higher-quality audiobooks and podcast productions, with a more natural and engaging listening experience for the audience. However, the path to achieving perfect voice replication remains complex and riddled with obstacles. Continued research is vital for overcoming these technical challenges and achieving a higher degree of accuracy and fidelity in the results.
Machine learning-based speech recognition, especially within the context of voice cloning, is significantly enhanced by using the International Phonetic Alphabet (IPA) as a reference. IPA's system of representing sounds is remarkably detailed, using over 1,100 distinct symbols to capture the intricate ways humans produce speech. This level of detail is crucial for training machine learning models to understand and accurately reproduce the complexities of human vocalizations.
The link between vocal tract shape and the resulting sound is now becoming a clearer focus in this field. Voice cloning systems are increasingly being designed to integrate IPA phonetic representations along with data about vocal tract configurations. This approach allows them to achieve significantly more accurate and high-fidelity reproductions of the voices they aim to clone.
Another aspect where IPA excels is in capturing features that go beyond individual sounds, like stress and intonation, which are essential for conveying emotional nuance in speech. This capability empowers voice cloning technology to replicate not just the words spoken, but also the underlying emotional tone, a feature of vital importance for applications like audiobook narration where conveying feelings is key to reader engagement.
Moreover, IPA’s universal nature makes it invaluable for capturing regional accents and dialects. It provides a shared framework for voice cloning systems to learn and reproduce the distinctive phonetic characteristics of different regional speech patterns. This is incredibly helpful for ensuring authenticity and cultural relevance across a variety of applications, especially in podcast production or in the creation of voiceovers targeted towards different communities.
IPA's usefulness goes beyond just individual sounds. It can capture aspects like speech rhythm and timing variations, allowing voice cloning systems to generate outputs that exhibit a natural flow, closely mirroring human speech. This focus on dynamic elements is crucial for improving listener comfort and acceptance.
One of the remarkable aspects of IPA is how it allows voice cloning systems to train efficiently on smaller datasets. This efficiency is possible because each IPA symbol represents a highly specific sound. This specificity helps models learn the core patterns of language faster and extrapolate more confidently, making it particularly beneficial for languages with limited available training data.
Beyond improved training, IPA can also enhance the robustness of voice cloning systems against background noise and other variations in recordings. This is achieved through the clarity of the phonetic transcriptions, which allows engineers to develop more sophisticated models that are less susceptible to audio artifacts often introduced by uncontrolled recording conditions.
The shared language that IPA provides for researchers globally has been instrumental in enabling international collaboration within the voice cloning field. With a standardized system, teams from different countries and regions can communicate more effectively and minimize misinterpretations regarding the nuances of phonetics.
However, the sheer breadth of IPA, with its 1,100+ symbols, poses a unique challenge for developers. To effectively train models, developers need to carefully prioritize and select the most relevant symbols for specific dialects without losing essential features. This careful selection helps optimize the training process.
Finally, voice cloning systems incorporating IPA have shown significant promise in enhancing real-time adaptability, particularly in applications like virtual assistants. The capability to quickly adapt to various user inputs ensures smoother and more personalized interaction experiences.
While significant progress has been made in integrating IPA into voice cloning and speech recognition, challenges remain, particularly in how we can most efficiently handle the complexities of dialect representation and achieve increasingly accurate voice cloning within diverse languages. Further research and development in these areas will unlock the full potential of IPA for generating truly natural-sounding synthetic voices that are not only understandable, but also authentic and culturally sensitive.
More Posts from clonemyvoice.io: