Get amazing AI audio voiceovers made for long-form content such as podcasts, presentations and social media. (Get started for free)
Acoustic Analysis Exploring Voice Cloning's Impact on Podcast Production in 2024
Acoustic Analysis Exploring Voice Cloning's Impact on Podcast Production in 2024 - Real-time Voice Cloning Advances in Podcast Creation
The landscape of podcast creation is rapidly evolving with the emergence of sophisticated real-time voice cloning systems. These systems, powered by deep learning methods, can now capture the essence of a speaker's voice from a relatively short audio snippet. This means that podcasters have a new tool to explore – the ability to generate speech in multiple languages and to experiment with a range of vocal styles with relative ease. The speed of the cloning process itself has improved to the point where voice generation can happen almost instantaneously, surpassing the actual duration of the cloned speech in some cases. This capability holds exciting potential to boost the creative process and to broaden accessibility for podcast creators who may have voice limitations.
However, these advancements don't come without potential drawbacks. The ease of replicating voices can create fresh opportunities for the malicious use of the technology for misrepresentation or disinformation, requiring careful consideration of the ethical implications of this emerging technology. The development and deployment of voice cloning in podcasts are undeniably headed for greater sophistication, prompting discussions on the responsible utilization of this power within the podcasting community.
1. Real-time voice cloning offers podcasters a new way to create audio quickly, streamlining editing and significantly reducing the overall production time needed to get a podcast episode ready. This can potentially reduce the pressure on podcasters who are pressed for time.
2. The latest voice cloning techniques don't just mimic a person's voice, they're able to capture subtle aspects like emotional tone, the rise and fall of pitch, and individual speaking styles. This makes the cloned voices sound very human, almost indistinguishable from the real thing at times.
3. Research shows that people often have trouble telling the difference between a synthesized voice and a real one. This raises some concerns about how this might affect the trust and authenticity that is usually associated with podcasts.
4. The increasing sophistication of voice synthesis software has allowed for the development of custom voice avatars, giving listeners a choice of who "reads" their audiobooks or podcasts. This is a new and interesting avenue for podcast production.
5. Researchers have learned that voice cloning's accuracy can be boosted by using acoustic characteristics from a combination of recordings. This leads to a richer and more believable listening experience for the audience.
6. Unlike the early versions of voice cloning, which required a vast amount of training data, the new models can create unique voices with only short snippets of audio. This makes it easier for podcast creators to experiment with a range of voices for their shows.
7. Some of the latest voice cloning tools can adjust to things like background noise and different microphone qualities in real-time. This keeps the audio clear and cuts down on the work needed to edit the audio in post-production.
8. While voice cloning certainly makes podcasting easier, it also presents some ethical questions, especially concerning obtaining permission and the risk of creating misleading or false audio material.
9. As the neural networks powering voice cloning become increasingly advanced, there's a growing interest in combining it with interactive audio features. This could open up possibilities for podcasts to become more engaging, such as incorporating personalized storytelling for listeners.
10. The quality of synthesized voices has improved dramatically thanks to progress in signal processing. This results in more robust and satisfying audio for listeners, potentially leading to better engagement with podcast content.
Acoustic Analysis Exploring Voice Cloning's Impact on Podcast Production in 2024 - Emotion Control Breakthroughs in Synthesized Speech
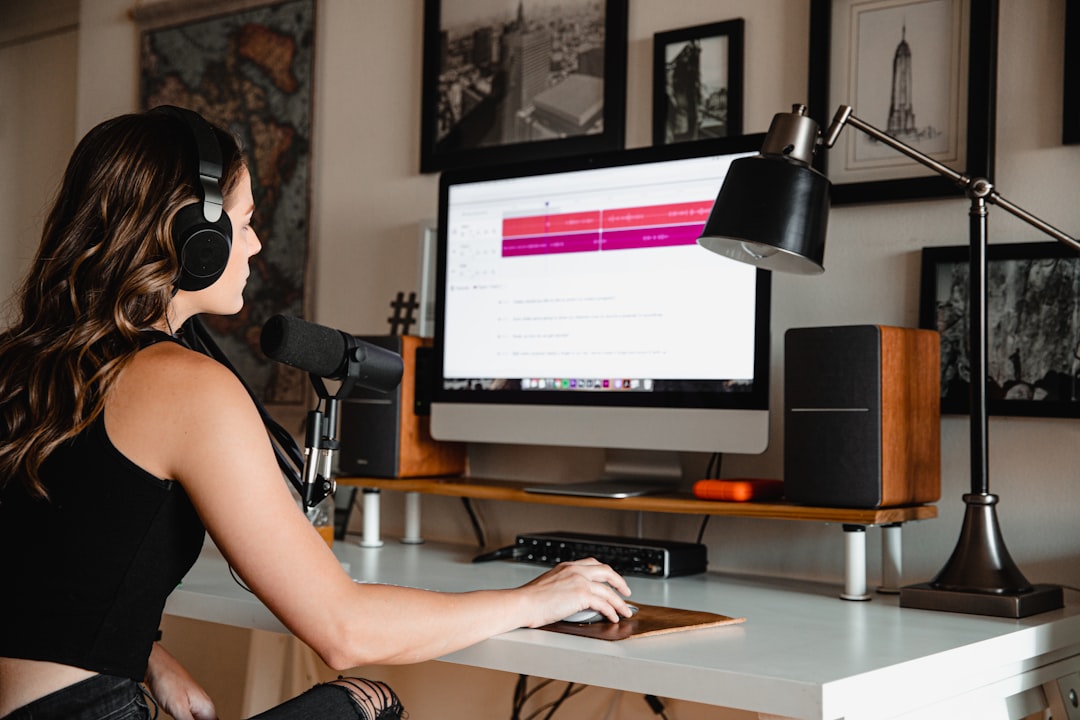
The field of synthesized speech has seen significant advancements in the area of emotion control, impacting various audio production sectors, including podcasting and audiobook creation. These improvements stem from innovative algorithms that analyze acoustic features like pitch and volume to both recognize and replicate human emotions. This newfound capability to inject emotional depth into synthetic voices has led to a more compelling and immersive listening experience. In some instances, these synthetic voices have become nearly indistinguishable from human speakers, blurring the lines between artificial and natural speech.
Furthermore, the rise of controllable expressive speech synthesis systems has opened exciting new avenues for personalized audio. By integrating emotion analysis with speech generation, content creators can now craft audio experiences designed to evoke specific emotions, greatly enhancing storytelling within podcasts and audiobooks. However, with this progress comes the crucial need to address ethical considerations. The ability to manipulate emotions in synthesized speech carries with it a risk of misrepresentation and deception, demanding a careful examination of authenticity and responsible content creation within audio mediums.
The field of synthesized speech has seen significant advancements in its ability to convey emotions, raising intriguing questions about the future of podcasting and audiobook production. Research shows that synthesized voices can now trigger emotional responses similar to those elicited by human speakers, suggesting that voice cloning can effectively replicate not just sound, but also intricate emotional states.
These breakthroughs leverage algorithms capable of analyzing acoustic features like speech tempo, intonation, and volume changes, allowing for nuanced performances in synthesized speech. Techniques such as prosody modeling have shown a positive impact on listener engagement, enriching the synthesized voice with emotional depth and narrative urgency. It's fascinating to note that the emotional impact of a synthesized voice can be influenced by the surrounding audio elements, suggesting that the overall listening experience shapes how listeners perceive the synthetic voice.
Furthermore, the effectiveness of voice cloning can vary across cultures, highlighting the need for cultural awareness when creating synthesized voices aimed at a global audience. Some machine learning models are even able to learn from limited datasets using techniques like Transfer Learning, allowing for a faster adaptation to new emotional tones without the need for extensive training data. These advancements have found a practical application in audiobooks, where they allow narrators to fluidly switch between characters with varying emotional states, enhancing the storytelling experience.
Interestingly, research suggests that subtle acoustic variations in synthesized speech can influence the audience's psychological response. For example, higher-pitched voices may be perceived as more trustworthy, while lower-pitched voices can convey authority, impacting how the audience connects with the podcast content. The goal of injecting emotional cues into synthesized speech is moving towards real-time adaptation, where the voice dynamically responds to live listener feedback during a podcast.
Perhaps surprisingly, acoustic studies have shown that listeners often prefer synthesized speech with slight imperfections, like breath sounds and minor hesitations, creating a more natural and relatable listening experience. This counterintuitive finding highlights the delicate balance between synthetic and human-like speech and underscores the complexity of human perception of audio signals. The field of synthesized speech continues to develop, holding the potential for creating richer and more immersive experiences in the world of audio content.
Acoustic Analysis Exploring Voice Cloning's Impact on Podcast Production in 2024 - Multilingual Content Production Streamlined by AI
AI is dramatically altering how multilingual audio content is created, especially within the podcast and audiobook spheres. Voice cloning technologies are now able to generate high-quality audio in multiple languages, significantly speeding up the production process and making it more efficient. New tools that clone voices quickly using just a short audio sample empower creators to experiment with a variety of voices and styles, improving engagement and making their work more accessible to a wider audience. While this streamlines production, it also prompts us to consider how this might impact the trustworthiness of the audio we consume. The potential for cloned voices to be used to spread misinformation or deceive listeners necessitates a thoughtful discussion around the ethical use of this technology as it continues to become more advanced. This change isn't merely about convenience; it's about how we tell stories and communicate across languages within the growing world of multimedia content.
The ability to incorporate multiple languages into voice cloning opens up new possibilities for podcast creators to reach a wider global audience without needing a separate voice actor for each language. This reduces the time and effort required for producing content in diverse languages, significantly streamlining the workflow and potentially making podcasting more accessible internationally.
Moreover, recent advancements in voice synthesis are enabling real-time translation. This implies that podcasters could theoretically deliver episodes simultaneously in multiple languages, potentially breaking down communication barriers and fostering direct audience interaction across diverse linguistic communities. This could revolutionize the podcasting experience, creating a more interconnected and responsive medium.
Some cutting-edge neural network models use audio input to fine-tune the tonal characteristics of synthesized speech while also retaining the specific nuances of each language. This helps to ensure a more natural and authentic-sounding output, leading to a more enjoyable listening experience in different languages. It's exciting to consider the implications this has for bridging cultural divides through shared audio experiences.
However, it appears that the emotional response a synthesized voice elicits can be impacted by the language being used. For example, listeners might find a synthesized voice speaking a foreign language more stimulating or intriguing than one speaking a familiar language. This could influence how podcasters approach audience engagement based on language and culture.
This ability to generate multilingual voice characters can be useful for audio dramas and storytelling. Podcasters could potentially design unique scenarios without being limited by finding actors for every character and language needed. This would open up a greater range of storytelling possibilities within the podcast format itself.
The acoustic design of synthesized multilingual voices has become increasingly sophisticated, incorporating aspects of cultural phonetics. This means the generated voices can be tweaked to reflect specific regional accents and pronunciation styles, which in turn, potentially increases audience engagement and relatability. It is a subtle but potentially impactful change that can create a deeper connection between listener and content.
These advances in voice synthesis allow creators to dynamically integrate sound effects and background audio within the context of multilingual content. This opens the door for creating richer and more intricate audio experiences. This layered narrative approach holds potential for captivating listeners within varied linguistic environments.
Voice cloning platforms are starting to develop the ability to adjust their speech patterns based on listener feedback and analytics. This interactive capability allows podcasters to refine the tone and style of their podcasts in real time, potentially catering to different audience segments and demographics within various language groups.
It's worth noting that how believable a synthesized voice sounds can depend on the language being used. Synthesized voices speaking English may face a more stringent level of scrutiny for naturalness compared to voices in other languages. This highlights that listeners' perceptions of authenticity can be influenced by a wide variety of factors, including cultural expectations of different languages.
The increasing sophistication of multilingual voice cloning also requires us to consider how to better evaluate the quality of the generated voices. Listeners likely won't tolerate awkward phrasing or poor pronunciation in synthetic speech. This necessitates the development of rigorous quality control systems and context-aware learning models for producing high-quality and natural-sounding multilingual audio content.
Acoustic Analysis Exploring Voice Cloning's Impact on Podcast Production in 2024 - Open Science Boosting Acoustic Analysis Research
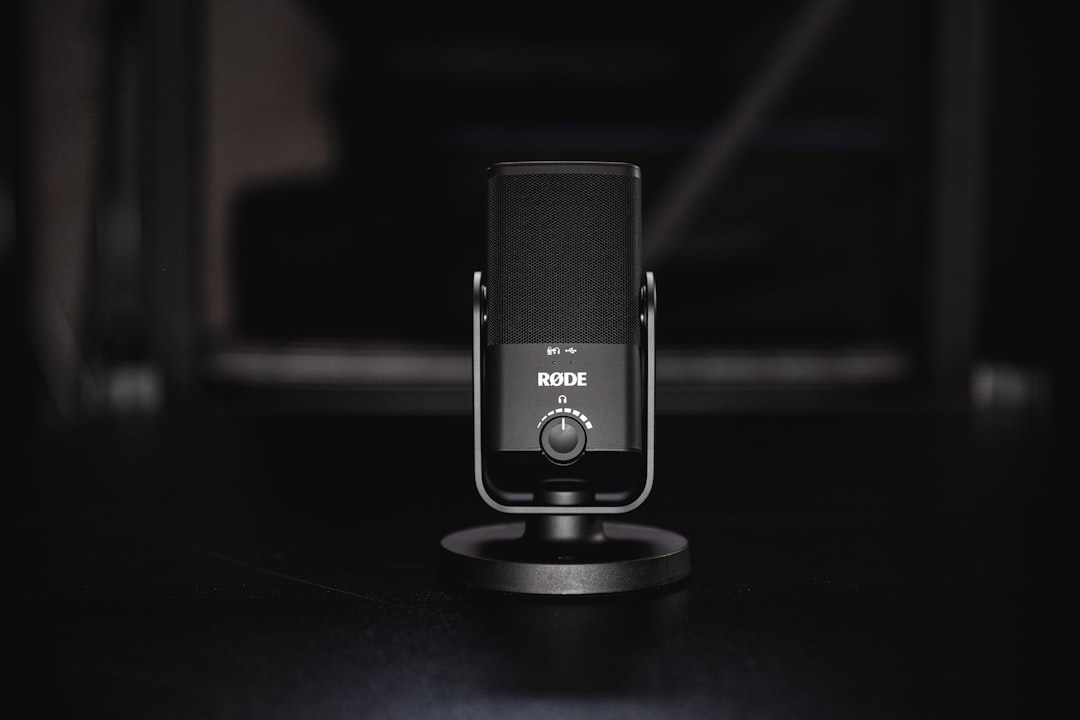
Open science principles are significantly impacting acoustic analysis research, particularly in areas like voice cloning. The increased availability of speech datasets, thanks to open practices, is fueling more advanced analysis and encouraging cross-disciplinary collaborations. This opens doors to fresh research questions and insights into how voice technology functions. However, the analysis of these datasets often requires a lot of manual or automated work, which can be a barrier for researchers, especially given the pace of developments in voice technology. Furthermore, the way we currently evaluate voice quality is still under scrutiny, whether we are talking about how it sounds to a person, how we measure it acoustically, or even how the vocal system works in the body in both medical settings and research. These are important areas of discussion in this fast-changing field where the use of synthesized voices in podcasts and audio media is ever evolving.
Open science initiatives have significantly broadened access to speech datasets, a boon for researchers exploring acoustic analysis in areas like voice cloning. Analyzing these datasets often requires manual or semi-automated methods, a process that's both time-consuming and potentially necessitates laboratory support. Voice analysis is a critical component across a range of research and commercial applications, indicating a strong need for researchers from diverse backgrounds to collaborate and explore fresh research avenues.
Currently, evaluating voice quality presents some significant hurdles, particularly when combining auditory perception, objective acoustic measurement, and aerodynamic assessments – challenges relevant in both clinical and research settings. Researchers are exploring innovative approaches to teaching voice acoustics, including leveraging online focus groups to understand the most effective ways to present these topics in graduate-level courses focused on vocal disorders.
The impressive development of voice technology and natural language processing tools has helped researchers identify vocal biomarkers that could improve clinical diagnostics and patient monitoring. Research on voice acoustics has shown that coaching sessions can lead to a change in vocal habits, often involving tenser phonation, suggesting alterations in how the larynx muscles are being used.
Acoustic measures are indispensable tools for differentiating between healthy and abnormal voice qualities. They're used to help distinguish between possible causes of voice issues and to evaluate the effectiveness of treatment in speech therapy. Tutorials on acoustic analysis methods illustrate their practical applications in both clinical and research contexts, emphasizing their ability to track changes in voice therapy interventions.
The software tool, Praat, has become a standard for analyzing and visualizing speech acoustics. It aids researchers in understanding how articulatory motions connect to acoustic filters and sources. While this tool is helpful, it is important to remember that limitations in technology and methodology can result in gaps in our understanding of the complexities of the voice. The goal is always to develop tools that better reflect the intricacies of the human voice.
Acoustic Analysis Exploring Voice Cloning's Impact on Podcast Production in 2024 - Machine Learning Approaches in Audio Categorization
Machine learning techniques are becoming increasingly important for sorting and categorizing audio, especially within the context of voice cloning and podcast creation in 2024. The use of deep learning has enabled more sophisticated acoustic modeling, improving the ability to pinpoint and classify different sounds and surrounding environments. This allows algorithms to differentiate between various audio elements, like speech and background noise, improving the overall quality and structure of podcasts. Furthermore, deep semantic learning methods are furthering our understanding of the context within which audio is produced. This is vital for creators trying to create captivating stories while simultaneously considering the ethical concerns related to the increasingly lifelike nature of synthetic voices. As these technologies mature, it becomes more important to consider the long-term impact on the perceived authenticity of audio, the degree to which it can be trusted, and the overall experience of the listener. These are complex issues that require ongoing, careful consideration.
Machine learning approaches to audio categorization are proving increasingly useful in sound production, including podcasting and audiobook creation. They can differentiate between various audio elements like music genres, distinct voices, and background noise, all of which can contribute to better sound design and content curation within podcasts.
These methods go beyond basic analysis like pitch and volume by utilizing techniques like MFCCs and spectrograms to detect intricate patterns within audio. This allows for a deeper understanding of the speaker's characteristics and their emotional state, valuable information for enhancing the overall quality of synthetic voices used in voice cloning applications.
Additionally, real-time audio categorization provides instantaneous feedback during podcast recording, enabling podcasters to fine-tune their audio on the spot. This can potentially save time and effort in the post-production phase. Unsupervised learning techniques are gaining traction in audio categorization, allowing for the classification of novel sounds without extensive human-labeled datasets. This is particularly beneficial when experimenting with less conventional audio elements within a podcast.
Furthermore, advanced audio categorization systems can categorize sounds based on unique qualities like timbre and rhythm, offering podcast creators a wider range of tools for sound design. They can integrate these elements seamlessly with dialogue, leading to more creative and unique sonic landscapes.
Machine learning has also enabled automatic tagging and keyword recognition, dramatically improving the podcast workflow. It streamlines searchability and content referencing, making the editing process considerably smoother. This capability is especially crucial when dealing with a large quantity of audio recordings and manipulating synthesized voices.
The application of audio feature learning has further implications for voice cloning. By recognizing subtle variations in vocal delivery, these algorithms can generate more refined and nuanced synthetic voices that closely mirror a speaker's unique vocal patterns.
It's important to acknowledge that the effectiveness of machine learning in audio categorization can be susceptible to environmental influences, like background noise and microphone quality. Researchers need to continually refine and improve these algorithms to ensure their accuracy in real-world podcasting conditions.
Interestingly, machine learning models analyzing vast audio libraries are revealing trends in listener preferences for certain sound characteristics. This knowledge can guide podcasters in optimizing their audio design to enhance emotional impact and improve listener retention.
As these methods continue to develop, there's a growing ability to isolate and analyze individual sound events, independent of their surrounding audio environment. This is critical for achieving clarity in synthesized speech within podcasts, especially in situations where distracting background sounds might hinder the listener's experience. The capacity to filter out these unwanted sounds can significantly enhance the polish of the final audio product.
Acoustic Analysis Exploring Voice Cloning's Impact on Podcast Production in 2024 - Ethical Considerations of AI Voice Technology in Media
The increasing sophistication of AI voice technology, especially in podcasting, compels us to confront a complex set of ethical dilemmas. The ability to replicate a person's voice with just a short audio snippet, through voice cloning, raises questions about whether this is a responsible use of technology, particularly regarding informed consent and the potential for invasion of privacy. In the world of podcasts, where building trust with listeners is paramount, the emergence of synthesized voices that are nearly indistinguishable from human speakers introduces a challenge to the authenticity listeners expect. As the technology becomes more readily available, concerns arise about the possibility of malicious use for spreading false information or creating deceptive content. These concerns necessitate the development and adherence to ethical guidelines that safeguard individuals and uphold the integrity of the audio medium. Open discussions within the podcasting and broader media landscape are essential to address the potential harms and benefits of this rapidly developing technology, ensuring its responsible and beneficial integration into media production.
AI voice technology, while offering transformative potential for media, presents a complex ethical landscape. Research suggests a considerable portion of the public feels apprehensive about the authenticity of synthesized voices, potentially leading to a decrease in trust when consuming audio content. This is especially relevant for podcasting, where authenticity plays a crucial role in establishing and maintaining a connection with listeners.
Voice cloning technologies are now capable of generating audio that's remarkably similar to real human speech. However, studies reveal that even subtle deviations in synthesized voices can trigger suspicion in listeners. This suggests that podcast creators face a delicate balancing act – leveraging the benefits of the technology while upholding audience trust.
When AI systems introduce emotional nuance into synthetic voices, listeners might find it challenging to differentiate between genuine human emotion and a cloned rendition. This raises critical questions around the ethical implications of emotional manipulation and the authenticity of expressed sentiments within media.
Beyond impersonation, voice cloning technology possesses the potential for misuse in harmful scenarios like identity theft and deceptive media practices. Researchers are raising alarms, creating a complex web of regulatory and ethical concerns for content producers and platforms.
AI-powered real-time audio translation is another area where ethical dilemmas arise. Without appropriate oversight, crucial aspects of tone and context can be inadvertently lost, potentially distorting the intended message and diminishing cultural nuances inherent in the original content.
Contrary to the assumption that perfect synthesized voices represent a desirable achievement, studies indicate that audiences often prefer voices with subtle imperfections, such as breath sounds and hesitations. This complicates the ethics of pursuing 'perfection' in voice cloning, prompting conversations around the inherent authenticity of audio.
Modern AI models are increasingly adept at replicating regional accents and dialects, raising a new ethical concern: how to cultivate cultural sensitivity while harnessing the nuanced vocal characteristics in podcasting. This involves avoiding potential reinforcement of stereotypes.
The expanding capabilities of voice manipulation naturally raise concerns regarding consent, particularly when cloning the voices of prominent figures or individuals in close relationships. This highlights the ethical responsibility for transparency in the application of such technologies.
AI-driven emotional synthesis within voice technology presents a fascinating and complex ethical issue. While this technology can enrich storytelling, it also presents risks of manipulative practices, where creators might leverage emotional triggers without revealing the synthetic nature of their content.
Finally, it's clear that integrating synthesized voices into multimedia storytelling significantly influences audience engagement. However, this positive impact demands that we develop and adhere to ethical guidelines that ensure emotional responses are fostered responsibly and not exploited for manipulative purposes.
Get amazing AI audio voiceovers made for long-form content such as podcasts, presentations and social media. (Get started for free)
More Posts from clonemyvoice.io: