7 Ways Text-to-Speech Latency Affects Voice Quality A Deep Dive into Real-Time Speech Synthesis
7 Ways Text-to-Speech Latency Affects Voice Quality A Deep Dive into Real-Time Speech Synthesis - Buffer Size Impact on Real-Time Voice Processing Speed
When it comes to voice processing, particularly in real-time applications like voice cloning or audiobook production, the buffer size plays a significant role in how quickly and smoothly the audio is processed. A larger buffer helps prevent glitches and distortions in the audio output, but it also increases the delay, or latency, in the system. This delay can become a problem, making the generated voice sound unnatural or even causing frustration for the listener.
On the other hand, reducing the buffer size can make the system more responsive, leading to a more natural-sounding voice. However, if the system's hardware can't handle the increased workload, you may end up with artifacts or distortions in the output. The challenge is to find the sweet spot—a buffer size that's large enough to ensure smooth, high-quality audio but small enough to maintain a low latency that feels natural to the listener.
This balance is critical for achieving a positive user experience, especially in applications where quick, natural-sounding voice output is essential. For instance, if a voice clone is used for an interactive podcast format or in creating audiobooks, excessive delays in processing can disrupt the listener's engagement. As the capabilities of processing hardware continue to improve, utilizing specialized chips designed for audio processing could allow us to use smaller buffer sizes without sacrificing audio quality, potentially pushing the boundaries of how realistic and responsive synthesized voices can become.
The size of the buffer used in real-time voice processing directly influences the delay, or latency, we experience between when a sound is input and when it's output. Larger buffers introduce more delay, which in voice interactions, like a conversation, can manifest as unnatural pauses or echoing effects that interrupt the flow. This can be particularly disruptive in live scenarios like voice cloning or interactive voice response systems.
On the flip side, shrinking the buffer size improves responsiveness. Voice data is handled with minimal delay, allowing applications to react quickly. However, this increased speed puts more strain on the processing power of the system, particularly noticeable on less capable hardware. It becomes more likely that audio glitches or interruptions can pop up if the system can't keep up with the processing demands.
The CPU workload in voice processing is heavily affected by the chosen buffer size. Smaller buffers mean more frequent handling of small data chunks, leading to a heavier load on system resources. This becomes especially important when dealing with systems that are already under pressure, like some older mobile devices or embedded systems designed for low-power environments.
In the world of audiobooks and similar applications, the buffer size plays a critical role in achieving quality. While the goal is to keep the delay low to make the listening experience more immersive, we also need to avoid overburdening the system with unnecessary processing. Excessive audio processing can degrade the quality of the audiobook, ultimately affecting the clarity and intelligibility that is essential for a good experience.
Voice cloning presents a fascinating application where buffer size plays a vital role in achieving a natural-sounding clone. Carefully chosen buffer sizes tailored to the nuances of the specific voice can contribute to a more convincing clone. With this precise control over timing and tonal shifts, you can further push the boundary of how expressive or convincing a synthesized voice sounds.
Podcast creation isn't immune to the impact of buffer size. For podcasters with multiple guests, careful management of the buffer becomes critical in handling the audio in real-time. The challenge here is the need for perfect synchronization between each voice to prevent any awkward or noticeable gaps in the recording. Imagine a poorly synchronized multi-person conversation– jarring!
The optimal buffer size is not a one-size-fits-all solution. Different applications and tasks will have different requirements. For example, real-time conversational platforms benefit from small buffer sizes because immediate feedback is crucial for a good user experience. Conversely, applications focused on large audio editing tasks might lean toward larger buffers to ensure efficiency during complex processing.
In the realm of real-time speech synthesis, if the buffer size is too large, it can cause a range of issues that affect the audio quality. Distortion or clipping of the audio can happen, impacting the natural and clear reproduction of the voice. Careful monitoring is crucial to ensure the sound remains clear and distortion-free.
Buffer size optimization extends to audio streaming. Well-tuned buffer settings enhance the streaming experience by reducing disruptions in audio transmission caused by dropped data packets. This, in turn, impacts both the quality for the listener and the performance requirements of the server sending the stream.
Finally, if buffer sizes are not carefully considered, the consequence can be something I’ve dubbed the "Lippy Effect". This describes the phenomenon where, due to the noticeable latency, users start feeling detached or frustrated during conversations with speech-based interactive systems, potentially diminishing their engagement. It emphasizes the critical role of optimal buffer management in crafting responsive, engaging speech technology.
7 Ways Text-to-Speech Latency Affects Voice Quality A Deep Dive into Real-Time Speech Synthesis - Network Streaming Delays in Cloud Based Voice Generation
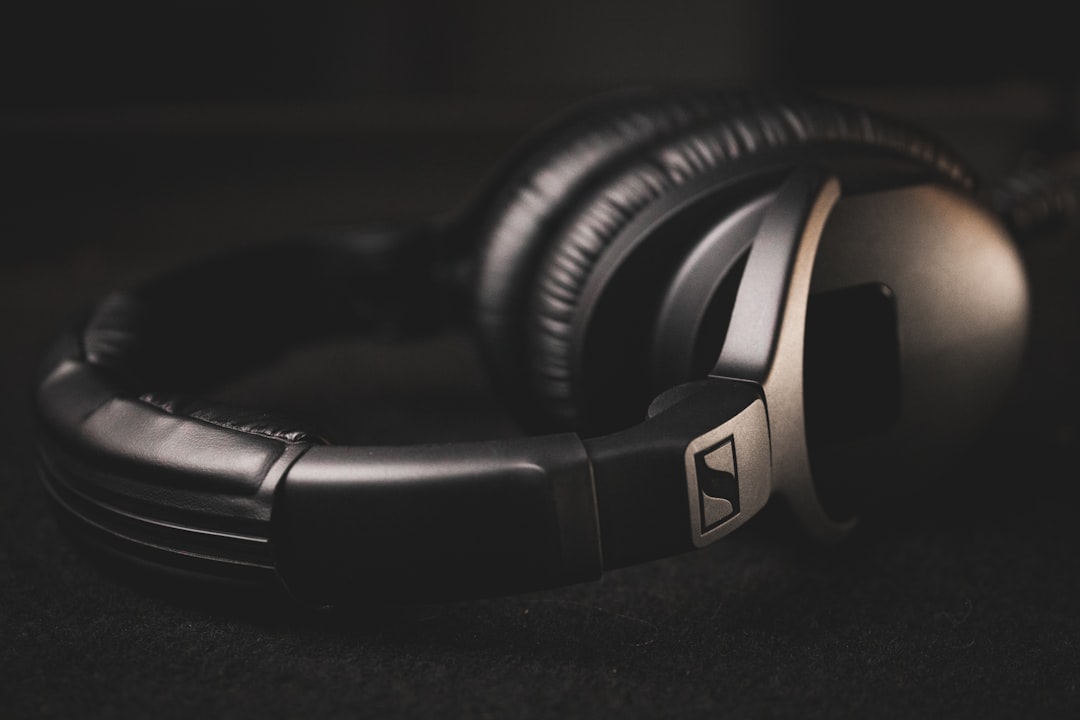
When generating voice using cloud-based systems, network delays can significantly impact the quality of the final product, especially for applications demanding real-time audio, like voice cloning or audiobook production. The process of speech synthesis relies on fast communication between the device requesting the audio (the client) and the server that handles the generation. Any lag in this communication can lead to unnatural pauses, breaks, or distortions in the generated voice, disrupting the flow and impacting the listener's experience. This is especially noticeable in dynamic applications, such as interactive podcasts or virtual conversations where immediate and seamless audio delivery is critical.
These delays can be particularly bothersome in applications where instant feedback is necessary. Imagine a voice clone participating in a lively conversation or a complex audiobook production with intricate narrative and sound design. Even small network delays can create disruptions that make the experience less enjoyable and break immersion.
To improve the quality of voice generated in such scenarios, optimizing the network environment is essential. Developers and users alike should focus on reducing network latency through efficient routing, higher bandwidth connections, or utilizing specific asynchronous techniques that can minimize delays. Implementing such solutions can ensure a smoother experience for listeners and prevent frustration stemming from interruptions or distortions.
In the realm of voice generation, it's evident that network delays can be a real hurdle to creating truly natural-sounding voices. Understanding the impact these delays have on the final audio is vital for creating applications that deliver an enjoyable and immersive audio experience. By working to minimize the effects of network latency, we can refine and improve the quality of cloud-based voice generation, bringing us closer to achieving truly seamless and lifelike audio.
Network delays are a major hurdle in cloud-based voice generation, particularly when striving for real-time interactions. While specialized hardware like GPUs and TPUs can accelerate speech synthesis, the unpredictable nature of internet connections can introduce unexpected delays, especially in cloud-based systems. It seems the human ear is quite sensitive to latency, with delays beyond about 200 milliseconds potentially leading to a perception of a less natural or robotic-sounding voice. Of course, the exact threshold varies between individuals and the specific context of the conversation.
This inherent variability in network conditions poses challenges for real-time applications, particularly in systems designed for interactive purposes, like voice-powered customer service. Imagine a virtual assistant that stutters or hesitates because of a brief network hiccup. It can negatively impact user perception and engagement.
Furthermore, the interaction of microphones and speakers in close proximity can be amplified by network delays, potentially creating unwanted echo effects. This is something frequently experienced in video conferencing, further degrading the user's experience and making it harder to follow the conversation.
The design of advanced voice systems incorporates adaptive buffering, which dynamically adjusts the buffer size based on the network conditions. This can smooth out the listening experience during periods of fluctuating bandwidth. However, it makes the system more complex to build.
We also have to think about the interplay between human and machine interaction times. When a person has to wait for synthesized speech, their patience begins to run thin after about half a second, making user experience a crucial part of design considerations for developers.
Advanced applications that incorporate multiple voice tracks need to delicately manage the latency for each track. If not carefully coordinated, it can lead to unpleasant phase issues, muddying up audio clarity, particularly a concern when producing podcasts. It highlights the intricacies of managing audio streams in complex audio projects.
Additionally, cloud-based voice generation can inadvertently mute the subtleties in tone that enrich human speech. The drive for efficient processing sometimes requires sacrifices in expressive capacity, which is quite a drawback for uses like audiobook production.
The network is never perfect, leading to data packet loss, which compounds the problem of latency. Fortunately, there are innovative techniques that can rebuild lost audio in real time. However, this introduces yet another processing step, potentially adding to overall delays.
In the context of complex language models used in voice generation, it becomes more challenging to produce truly natural-sounding speech. Those models often take extra processing time for complicated sentence structures or when interpreting natural language input. The more unpredictable the interaction, as is often the case in systems like virtual assistants, the greater the impact of these processing delays.
Finally, synchronizing different audio sources becomes more complex when latency isn't controlled. These discrepancies can lead to noticeable synchronization errors that hinder the quality of a podcast or live broadcast. It emphasizes the crucial role of managing latency to maintain an enjoyable, coherent auditory experience in complex audio systems.
7 Ways Text-to-Speech Latency Affects Voice Quality A Deep Dive into Real-Time Speech Synthesis - CPU Load Effects During Continuous Speech Synthesis
When generating speech continuously, the CPU's workload becomes a key factor in how well the audio sounds and responds. More complex speech models, for instance, often put a heavier burden on the CPU, leading to longer delays and occasional glitches in the audio. This can make the synthesized voice sound robotic or less natural, which isn't ideal for situations like voice cloning where authenticity matters or creating audiobooks where the listener's immersion is important.
As we improve the methods and designs used for speech synthesis, we are finding better ways to balance the CPU demands with a fast and responsive output. The focus is not only on making speech creation faster but also on maintaining a high-quality audio result. Developers are working to reduce the negative effects of heavy CPU loads by optimizing models and refining the way the audio is processed. The goal is to create a smoother and better overall user experience in real-time speech applications.
Generating speech continuously can put a strain on a computer's processor, or CPU, and how much it impacts the CPU depends on the intricacy of the voice model being used. For instance, crafting complex language or lengthy sentences demands more computational muscle, potentially leading to noticeable delays and a drop in audio quality if the CPU gets bogged down.
When tasks like real-time voice cloning push synthesis to its limits, the CPU can experience a surge in workload that surpasses its regular capacity. If the demands become excessive, the system might be forced to rely on slower processing methods, introducing unwanted disruptions like choppy or glitchy audio, which can seriously detract from the listener's enjoyment.
One strategy to alleviate these burdens is utilizing multiple processing threads. This allows tasks to be split across the CPU's cores more efficiently, not only boosting responsiveness in speech synthesis but also improving audio smoothness, especially when managing multiple audio streams simultaneously, like those needed for different voice tracks in podcast creation.
The fluctuations in the CPU's workload can also influence the subtle nuances in the generated voice. For example, factors like pitch control and overall tone quality may suffer during high CPU usage. Consequently, the voice might lose its natural quality, potentially sounding more synthetic and less like a human. This is particularly important for applications like recording audiobooks or creating voice assistants that strive for human-like interactions.
Sustained periods of high CPU load can lead to a phenomenon called thermal throttling. To avoid overheating, the processor automatically reduces its speed, impacting the fluidity of real-time speech generation and introducing delays. Maintaining adequate cooling for systems that frequently engage in intensive speech synthesis is critical.
Techniques for manipulating buffer sizes can help manage the CPU's workload. Smaller buffers can promote faster response times but at the cost of potentially overloading the CPU, while larger buffers help to manage the workload but introduce more delay. Achieving the ideal balance between responsiveness and clarity is key to a positive experience.
Some advanced speech synthesis systems have incorporated methods that dynamically adapt the processing load based on the CPU's current performance. This on-the-fly adjustment helps maintain consistent voice quality by automatically scaling the amount of processing based on the available resources.
In applications like voice assistants, the delay associated with CPU load can have a significant impact on how satisfied users feel. People generally expect instantaneous responses from such systems. Even minor delays can easily lead to frustration, underscoring the importance of thoughtful CPU management in the design of interactive voice-based interfaces.
Integrating convolutional neural networks (CNNs) into speech synthesis can offer increased naturalness in voice outputs but also leads to a corresponding increase in CPU load. Balancing the gains in voice quality with the impact on CPU performance is a challenge for developers.
And finally, after the core speech synthesis is done, there are often additional tasks, like noise reduction or audio enhancement, which can contribute to the CPU's workload. In audiobook production, where sound quality is paramount, carefully managing these post-processing stages is crucial to prevent lengthy delays that could detract from the listener's immersion.
In conclusion, understanding and mitigating the effects of CPU load during continuous speech synthesis are essential for building high-quality and user-friendly applications. Continuous improvements in processor technologies and innovative software methods will continue to push the boundaries of what's possible in speech generation, enabling ever more natural and interactive voice experiences.
7 Ways Text-to-Speech Latency Affects Voice Quality A Deep Dive into Real-Time Speech Synthesis - Memory Management Challenges in High Volume Voice Production
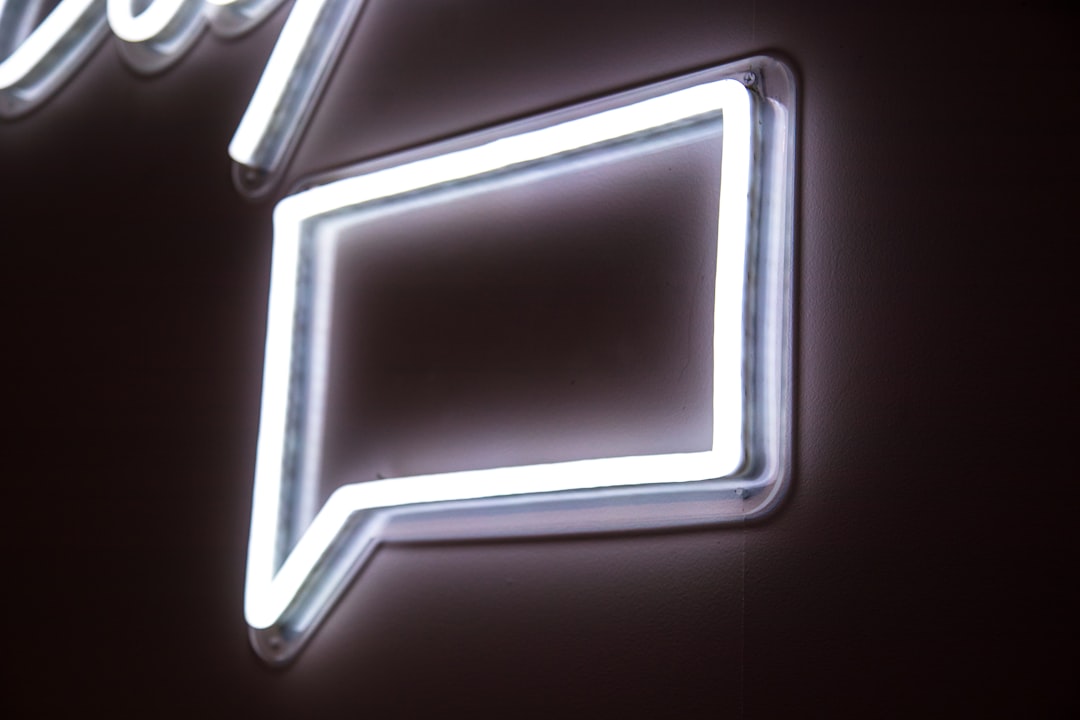
When producing large volumes of synthetic voice, particularly for applications like voice cloning or audiobook creation that demand realistic, real-time audio, managing memory effectively becomes a significant challenge. The intricate algorithms powering text-to-speech (TTS) systems demand considerable computational resources to achieve smooth, natural-sounding speech. If memory isn't allocated and utilized efficiently, it can lead to performance bottlenecks. These bottlenecks manifest as disruptions in the audio output, like unnatural pauses or glitches that detract from the overall listening experience. This can be a significant problem in applications where the goal is to seamlessly replicate human speech, such as voice cloning. As voice generation technologies continue to evolve in complexity, the need for robust memory management solutions will become even more critical. Optimizing memory strategies is increasingly important for fulfilling the requirements of producing high-quality synthetic speech. Failing to effectively manage memory can limit the capacity of synthesized voices to convey nuanced expressions and emotions. Developers need to focus on improving memory management strategies to keep up with the rising demand for advanced and lifelike audio output.
In the realm of high-volume voice production, like crafting audiobooks, voice cloning, or even intricate podcast productions, the way memory is managed becomes a surprisingly significant factor in overall quality and performance. This is especially true in real-time applications where voice characteristics—pitch, tone, accents—need continuous and rapid processing to create convincing audio.
One key challenge lies in how we handle the constantly shifting demands of voice parameters. We need to dynamically allocate and release memory, which can be resource-intensive. This dynamic memory management is essential for ensuring smooth audio output, but it adds complexity to the system.
Another issue comes from the way audio is frequently processed in chunks. While this approach helps manage memory efficiently, it can introduce a trade-off. Smaller chunks reduce memory pressure, but lead to more frequent memory access and ultimately more latency. This latency can lead to audio that is less clear and responsive, potentially harming the realism of a voice clone or making an audiobook less enjoyable.
It's also fascinating how much reliance we place on CPU caches. They can greatly speed up memory access, but if the system's memory access patterns don't align well with the cache design, it can hamper performance. This mismatch can lead to audio that is noticeably less clean, impacting the overall listening experience.
Then there's the challenge of languages with built-in garbage collection. These automatic clean-up processes are usually a good thing, but in a high-volume voice production environment, they can be disruptive. If garbage collection happens during audio generation, it can introduce unpredictable delays, causing glitches or stutters that detract from the seamless audio experience.
And if that wasn't enough, we also contend with memory fragmentation. It's like a jigsaw puzzle where we have plenty of pieces but can't form a complete image because the pieces are scattered. This problem arises because voice processing constantly creates and deletes memory blocks of various sizes. Ultimately, the system's ability to respond quickly is impacted because it can't readily use the available memory.
Some sophisticated systems attempt to manage memory in real-time by adjusting it as needed. However, these adaptive approaches add a layer of complexity that demands extra computing power. The resulting increase in CPU load can complicate the process of generating audio efficiently.
It’s also worth noting that modern deep learning methods used for voice generation are memory hogs. Neural networks, particularly recurrent neural networks (RNNs), require a large amount of memory not only to store their internal structure but also to process voice data efficiently. Managing this memory usage while maintaining real-time performance is a difficult juggling act.
We've also found that the memory's performance itself can be sensitive to environmental conditions. High CPU loads and other factors can lead to increases in temperature, which can impact the reliability of memory. Any hiccup in memory access in these crucial areas can lead to noticeable audio distortions.
Even the choice of sample rate for audio affects memory requirements. Higher sample rates produce better quality but increase the amount of data the system needs to process each second. We need clever memory management to avoid performance issues and the resultant audio quality degradation.
Finally, the system needs to be prepared for interruptions or errors during processing. The goal is to gracefully recover from these situations without major disruptions. If audio stops and starts or becomes distorted in audiobooks or podcasts, it can break the listener's immersion and become annoying quickly.
The challenges of memory management in high-volume voice production highlight how intertwined the different parts of the system are. Each part—from the deep learning models to the audio buffers to the physical memory chips—affects the others. Finding the optimal solutions will continue to drive the evolution of voice technology as we strive to achieve ever more natural-sounding voices.
7 Ways Text-to-Speech Latency Affects Voice Quality A Deep Dive into Real-Time Speech Synthesis - Audio Driver Configuration Role in Output Quality
Audio driver configuration is vital for achieving high-quality output, particularly in scenarios like voice cloning or podcast production where sound clarity and responsiveness are crucial. Properly configuring these drivers helps address potential issues, especially when it comes to minimizing DPC latency. DPC latency, a type of delay, can create noticeable disruptions in the audio output, negatively affecting the naturalness of a synthetic voice.
Furthermore, driver configuration can influence how the system manages resources, such as CPU core allocation, which can directly impact audio dropouts during complex speech processing. Utilizing asynchronous audio methods during the speech synthesis process can help reduce latency, improving the overall smoothness and responsiveness of voice interactions.
The bitrate used in the audio output also directly impacts how natural and detailed the voice sounds. Higher bitrates usually mean higher audio fidelity, resulting in a more refined and lifelike sound. Using background audio or other sound effects can improve the engagement, but it is important that those components are mixed well to not mask or negatively affect the quality of the voice output.
Ultimately, ensuring that all audio drivers are kept up to date and configured correctly is essential for avoiding audio glitches and achieving the best possible audio quality. This is critical when creating voice clones, producing audiobooks, or building a podcast in situations where even minor disruptions can be noticeable and detract from the experience.
The way we configure audio drivers plays a surprisingly important role in the quality of the sound we hear, particularly when we're working with things like text-to-speech (TTS) systems, voice cloning, or audiobook production where minimizing delays (latency) is key. For example, the bit depth setting in the driver can affect the overall dynamic range of the sound. Higher bit depths, like 24-bit, can capture a wider range of audio, from very soft to very loud, without distortion. This can be crucial for creating a realistic and engaging listening experience in projects where there's a wide range of audio levels, such as audiobooks or intricate podcast productions.
However, it's not always a simple trade-off. While we might want to push for the highest quality settings, features like the audio sample rate, which controls how often samples are taken in the audio, can influence latency. While increasing the sample rate can improve clarity, it also adds to the load on the CPU, making it more likely that we run into problems with audio glitches or dropouts, particularly in real-time situations like voice cloning. This can be an unfortunate consequence for users who are trying to achieve more natural-sounding voices.
The configuration of audio interfaces, which connect audio hardware to a computer, can make a big difference as well. If they're set up poorly, it can increase the risk of buffer underruns which lead to choppy or broken audio. This is particularly important for real-time audio applications.
Even the operating system you're using can influence how audio drivers behave and contribute to latency. Some operating systems, like Windows, might introduce a bit more latency into the process compared to other options like macOS or Linux, making it important for developers to be mindful of this and optimize their systems and software accordingly.
In addition, it's wise to regularly update your audio drivers, since manufacturers release updates that include optimizations for a variety of audio processing tasks. Ignoring these updates could lead to compatibility issues and gradual degradation in audio quality over time.
The world of audio drivers also includes features like ASIO drivers which are explicitly designed for low-latency audio performance. For applications like podcasts or interactive content where timing is incredibly important, ASIO drivers can be a real benefit in improving overall sound quality and reducing latency.
Another important aspect is the buffer size in the driver's configuration. This buffer helps to store audio data temporarily before it's processed or sent to the output devices. A poorly configured buffer size can have a significant impact on how well the system handles latency in real-time applications. This is particularly important for applications like text-to-speech systems or voice cloning where maintaining a smooth and responsive audio experience is a top priority.
Something to consider, as well, is the idea of jitter, which can occur when packets of audio data arrive irregularly during transmission over a network. Jitter can significantly influence the sound quality in applications that rely on voice over the internet or in cloud-based voice production. Luckily, the driver can help control jitter, contributing to smoother audio playback and higher clarity in live sessions or during synthetic voice generation.
The way audio is handled through the different APIs—such as DirectSound and WaveOut—can also impact the performance and quality of audio. DirectSound typically has lower latency than WaveOut, making it preferable for real-time applications like voice response systems.
Finally, there's the concept of sample rate conversion, where audio needs to be changed from one sample rate to another as it's transmitted between different parts of a system. Each conversion step has the potential to introduce a little bit of audio quality loss. So, ensuring that the audio drivers maintain a consistent sample rate throughout the whole processing pipeline is crucial for keeping audio integrity, especially in applications where audio quality is vital like in audiobook creation or complex podcasts.
In conclusion, proper audio driver configuration is about much more than just getting the audio to work. It's intricately linked to the quality of sound, latency, and responsiveness of the system, particularly for projects that focus on real-time voice generation, audiobooks, voice cloning, and podcasting. As we continue to explore advanced applications in this space, understanding these nuanced relationships will only become more important.
7 Ways Text-to-Speech Latency Affects Voice Quality A Deep Dive into Real-Time Speech Synthesis - Speech Model Size versus Processing Time Tradeoffs
When it comes to generating speech using text-to-speech (TTS) systems, the size of the speech model used has a direct effect on how long it takes to process the text and produce audio. Larger speech models, which are essentially complex sets of instructions that define how the voice should sound, usually generate higher quality audio. They can better replicate human-like qualities in a voice like natural intonation or subtle variations in speech patterns. However, this improvement often leads to a longer processing time, which is a problem when the speech needs to be generated in real-time, such as during voice cloning, creating audiobooks, or recording podcasts.
This means there's a constant tension between getting the best audio quality and keeping the delays to a minimum. While a more complex voice might be incredibly engaging for listeners, if there are long pauses between words or sentences due to processing time, the experience can feel disjointed or unnatural. It's a bit like trying to have a conversation with someone who is constantly lagging behind – it can be very frustrating.
As we continue to develop more sophisticated speech synthesis technology, a key challenge will be finding ways to improve processing speed without losing the benefits that come from more complex speech models. The goal is to create a situation where users have a truly natural and engaging audio experience, whether it's a captivating audiobook or an expressive voice clone.
The size of a speech model significantly impacts its processing time, with larger, more complex models demanding greater computational resources and leading to longer processing times. This can translate into several seconds of latency, potentially hindering the naturalness of the synthesized voice, especially in applications like voice cloning that rely on real-time audio. For instance, if we're trying to create a convincingly realistic voice clone, too much latency will make it sound artificial and disrupt the illusion.
Finding the right balance between responsiveness and audio quality often involves adjusting buffer size. Decreasing the buffer size can enhance responsiveness, but this puts more stress on the system's resources, leading to increased chances of audio glitches or artifacts, especially when we're using highly complex speech models. This trade-off can be problematic for creating things like audiobooks because we want high quality, clear audio without distortions. If we try to squeeze out too much performance from the system, we compromise that quality.
Furthermore, real-time speech synthesis, in conjunction with CPU load and latency, can exacerbate problems caused by interference between nearby microphones and speakers. This is particularly problematic when we're trying to create interactive experiences, as it can lead to echo effects that can be incredibly distracting and potentially ruin the experience. It's important to consider those interactions when setting up any system.
Mobile devices often have limited CPU and memory capabilities, making it crucial to use speech models that are optimized for low processing times. If we fail to do this, we risk running into problems with dropped audio frames or interruptions during the production process, especially with features that rely on real-time audio, like dynamic podcasting where it can be crucial for smooth transitions and transitions.
Caching strategies play a surprisingly large role in the quality of audio produced. If the speech synthesis algorithm doesn't efficiently utilize the available CPU cache, it can lead to increased latency and degrade the quality of the generated audio, potentially harming the fidelity of a voice clone or audiobook.
When dealing with automatic memory management, such as garbage collection, in speech synthesis environments, we can encounter latency challenges. If garbage collection runs during crucial audio generation phases, it can produce random audio glitches, especially in large-scale projects like audiobook production.
Environmental factors such as temperature, particularly changes due to high CPU usage, can negatively affect memory reliability, leading to audio interruptions in real-time voice applications. This means it is important to consider thermal management as part of the speech synthesis process.
The audio sample rate that we use affects the overall quality of the audio output, but using a higher sample rate to achieve more detail and clarity requires more processing power. This means that if we choose a sample rate that is too high for the resources available, it will introduce noticeable delays in synthesized speech, damaging the user experience.
Cloud-based speech generation can be affected by jitter, or irregular transmission of audio data packets, which can degrade the quality and clarity of the output. Luckily, properly configured audio drivers can help reduce the effects of jitter, allowing for a smoother audio experience in applications that need real-time voice interactions.
Some complex speech synthesis systems utilize dynamic buffering, which allows the system to adjust buffer sizes on the fly in response to real-time CPU load changes. This approach can help create smoother audio output, but it also adds complexity to the system and can sometimes impact the ability to control quality or responsiveness.
It's clear that latency in speech generation is an intricate problem with significant implications for a range of applications. Developing robust, yet efficient models and systems will continue to drive progress and push the boundaries of how lifelike and realistic synthetic voices can be.
7 Ways Text-to-Speech Latency Affects Voice Quality A Deep Dive into Real-Time Speech Synthesis - Compression Algorithms Effect on Voice Clarity
Compression algorithms are essential for maintaining a balance between reducing the size of audio files and preserving the clarity of synthesized speech. This is especially important in applications like creating voice clones, producing audiobooks, or building podcasts where sound quality is a primary concern. When used well, they can strip away unnecessary data while retaining the naturalness of a voice. This allows for the efficient storage and transmission of audio, which is valuable for many uses.
However, excessive compression can lead to a loss of subtle audio nuances. This can cause the synthesized voice to sound robotic, lacking the natural variations in tone and inflection that make human speech engaging. It's a delicate balance, as using too aggressive of compression techniques can strip away essential parts of a voice, hindering the goal of producing natural-sounding audio, especially in real-time settings where a quick response is needed.
Deep learning methods have been applied to compression, showing potential for greatly improving audio quality and reducing file sizes. However, it is crucial to understand that the use of more complex techniques, while often improving the final audio, also introduces complexities into the processing pipeline, which can lead to delays and negatively impact the quality of the output. It can be a tradeoff that has to be carefully considered when choosing compression techniques. As voice technologies continue to improve and become more sophisticated, there will be an increased demand for compression methods that can balance audio clarity and efficiency in audio production environments.
Comprehending how compression algorithms affect voice clarity is crucial, particularly within applications like voice cloning, audiobook production, and podcasting where preserving the naturalness of the human voice is paramount. Lossy compression methods, like MP3 or AAC, while effective for reducing file sizes, can sacrifice sonic details in the pursuit of efficiency. They can trim away parts of the audio spectrum deemed less significant, often including higher frequencies essential for the clarity of speech, such as those 's' and 'sh' sounds that can become muffled. In audiobook production, this could easily muddle the narrative and reduce the listening experience.
A continuous trade-off arises between file size reduction and audio quality. When aiming for high-fidelity audio, which is frequently the goal in voice cloning or audiobook projects, increasing the bitrate can improve the sound quality during the compression/decompression processes. However, there's a consequence. Higher bitrate settings often result in increased latency, which is problematic for real-time voice interaction applications. Imagine the frustrating experience of a voice clone engaged in a conversation that sounds slightly delayed. It breaks the sense of natural back-and-forth.
The manipulation of audio dynamics via techniques like dynamic range compression also introduces tradeoffs. This approach can level out loudness variations in a voice, but excessive compression can 'flatten' the sound, eroding the subtle tonal nuances that give human voices their expressive nature. Losing these features can diminish the emotional impact of a well-read audiobook or make a voice clone seem overly processed and lifeless.
Thankfully, more advanced speech coding techniques like Linear Predictive Coding (LPC) help to navigate this balance better. This technique focuses on replicating the human vocal tract's movements, leading to a greater understanding of the sound produced. The advantage is improved voice clarity when bandwidth is limited. This has made them helpful for voice interactions over the internet through services like VoIP or streaming platforms.
Error resilience, a key feature built into many compression algorithms, becomes particularly important when dealing with cloud-based streaming of audio. These techniques mitigate the issues that occur with dropped data packets. The end result is less interruption and greater clarity for the listener during interactive conversational scenarios, such as a podcast where maintaining a consistent flow is very important.
Interestingly, how an audio codec handles temporal resolution affects the nuances of a generated voice. Poor temporal resolution in a codec can lead to synthesized speech sounding slow and robotic. This negatively impacts any scenario where expressive audio is important, such as voice assistants or dynamic storytellers.
Perceptual coding is yet another method of compression that attempts to take advantage of how humans perceive sound. It uses clever tricks to eliminate the less perceptible components of sound to compress the file without sacrificing the overall sound quality. However, if applied poorly, it could erase subtle features of the voice, which is a drawback in scenarios like audiobooks or complex narratives where the speaker's articulation is important.
It's also important to realize that there's a fundamental delay built into each compression algorithm. These delays, or latency, can vary widely between codecs. There are low-latency options like Opus, which are suitable for real-time communication and interaction. But others might introduce noticeable delays that disrupt conversations in applications like voice cloning.
The field of artificial intelligence has also begun to impact compression, with AI-driven codecs that adapt dynamically to audio. These algorithms offer the possibility of near real-time adjustment of compression levels based on the characteristics of the voice. This proves beneficial when audio quality conditions vary rapidly, such as during a live audio feed or collaborative podcast creation.
It's worth remembering that voices each have unique characteristics like pitch, tone, and timbre, which in turn interact with compression algorithms in different ways. Consequently, understanding those interactions is important for audio engineers who want to retain the integrity of a voice in any application, particularly where professionalism is essential, such as in voiceovers. The nuances of the voice need to be maintained, or it becomes detrimental to the desired outcome.
In the continually evolving landscape of voice technologies, understanding the strengths and limitations of compression algorithms is critical for producing high-quality audio for applications such as audiobooks, voice cloning, and podcasts. As researchers, it's clear that future work in this area will need to continue refining methods and approaches to improve compression and minimize the impact on voice clarity in real-time audio applications.
More Posts from clonemyvoice.io: