Get amazing AI audio voiceovers made for long-form content such as podcasts, presentations and social media. (Get started for free)
7 Hidden Features in Audacity for Professional Voice Recording That Producers Often Overlook
7 Hidden Features in Audacity for Professional Voice Recording That Producers Often Overlook - Voice Separation Tool Through Advanced Spectrum Analysis
Audacity's capacity for voice separation leverages the power of spectrum analysis, pushing the boundaries of what's possible with audio editing. The ability to visualize the frequency makeup of audio using tools like the Spectrogram and Plot Spectrum provides a detailed understanding of the various sound components within a recording. This deep dive into the sound's frequency structure unlocks the potential for advanced audio manipulation.
The inclusion of tools like OpenVINO Music Separation, which utilizes specialized algorithms, empowers users to meticulously isolate vocal tracks from instrumental elements. This feature shines when crafting audiobooks or podcasts, where clean and isolated vocal tracks are crucial for achieving a professional-sounding product. Beyond simple separation, the 'Spectral Edit Multi Tool' allows for fine-grained control, enabling the removal of specific frequencies that detract from the overall quality of the sound. Through a powerful union of spectral analysis and intelligent separation techniques, Audacity empowers producers to meticulously shape the audio landscape and elevate the production value of their work.
Audacity's ability to delve into the frequency makeup of sound through spectrum analysis is fascinating. By visualizing the different frequencies present in a recording, we gain a clearer understanding of how individual voices interact, particularly in complex mixes where voices may overlap or blend. Each person's voice possesses unique sonic characteristics, a kind of 'fingerprint' that spectrum analysis can help us identify. This is crucial when attempting to extract a specific voice, even when it's embedded in a noisy environment.
Many of the newer separation algorithms rely on the power of machine learning. By sifting through mountains of audio data, these algorithms learn to distinguish and separate voices with increasing precision over time. Techniques like phase cancellation become incredibly important here. By selectively attenuating specific frequencies associated with a particular voice while boosting others, we can achieve a cleaner isolation of individual tracks. The quality of the original recording is paramount, as the resolution of the audio directly impacts the effectiveness of these tools. Higher sample rates capture more detailed frequency information, making spectral analysis sharper and yielding better separation results.
The applications of this technology are becoming increasingly prevalent. In audiobook productions, isolating and manipulating various voice actors becomes seamless, enabling creative adjustments without impacting overall audio quality. Podcasters, similarly, can leverage these tools to mitigate the effects of overlapping speech, ensuring clarity and improving the listening experience. Beyond mere isolation, certain advanced methods can even reconstruct lost or corrupted parts of the audio signal, making it possible to salvage otherwise unusable recordings. We're even seeing advancements in real-time separation, which could open up exciting new avenues for live performances and recording. However, we should acknowledge that the effectiveness of voice separation tools can vary significantly. Things like vocal pitch, tone, and other vocal qualities can heavily influence the results, making the ability to isolate individual voices with perfect clarity a challenging goal in many situations.
7 Hidden Features in Audacity for Professional Voice Recording That Producers Often Overlook - Audio Streaming Capability With Built In DAW Integration
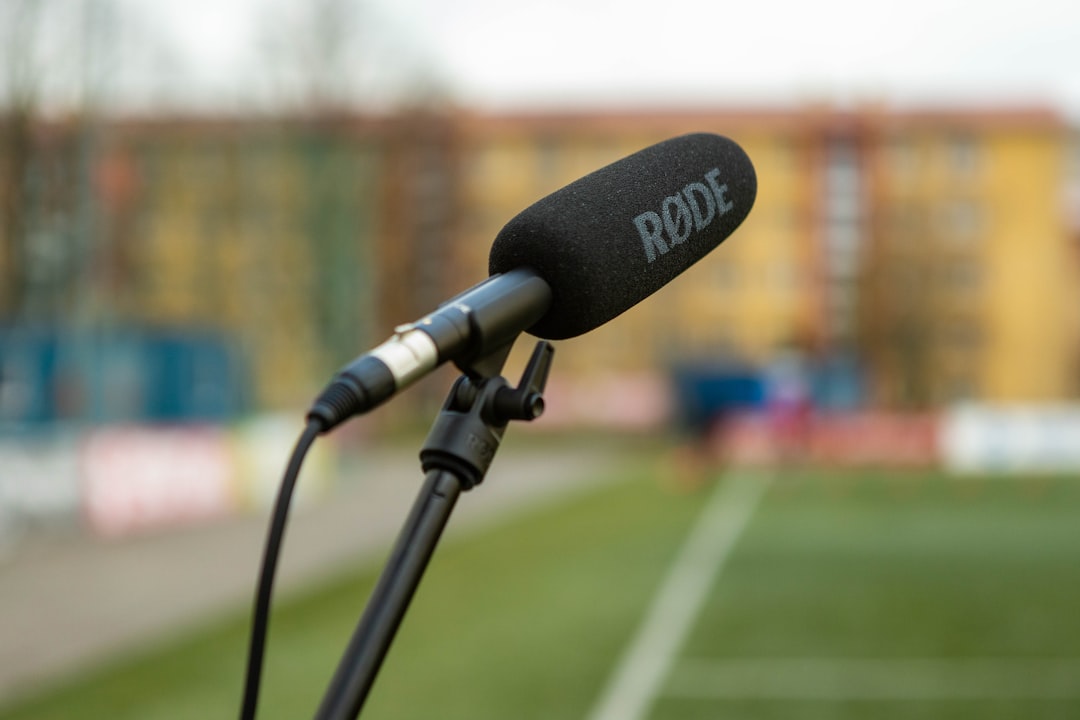
Audacity's newly integrated audio streaming capability, coupled with its DAW functionality, offers a noteworthy upgrade for producers engaged in voice recording, audiobook production, and podcasting. This feature enables smoother workflows for live or pre-recorded sessions by permitting the connection of microphones and other audio equipment through audio interfaces. The integration, while seemingly a boon for productivity, might not fully measure up to more robust digital audio workstations in terms of advanced features. Despite this caveat, Audacity's ability to function alongside professional DAWs fosters promising collaborative potential, expanding creative horizons for audio producers. Furthermore, with plugins like LISTENTO, audio streaming becomes more readily available, encouraging exploration of novel creative avenues within the sound production realm. While this offers promise, one should remain mindful of the potential limitations compared to dedicated professional audio software.
Audacity, while a versatile tool, hasn't fully embraced the dynamic world of real-time audio streaming in the way some dedicated Digital Audio Workstations (DAWs) have. While there are plugins like LISTENTO that facilitate streaming, it's not inherently integrated, which can create workflow hurdles for certain production scenarios. Imagine a scenario where you're recording a voice-over for an audiobook, and you want to stream it live to collaborators for feedback – the current Audacity setup doesn't offer a seamless solution for that.
The concept of integrated DAWs with streaming capabilities is intriguing. This would potentially allow for real-time mixing and adjustments during a voice cloning session, offering immediate feedback and ensuring a smoother creative process. Consider the example of a voice actor attempting to replicate a specific voice – real-time feedback through streaming would allow them to fine-tune their performance more effectively. Furthermore, the capacity for multi-track streaming within Audacity would be beneficial for collaborative voice acting projects, especially when working on audiobooks with multiple narrators or character voices.
However, there are challenges to consider. Achieving low-latency streaming with Audacity's architecture might be problematic. Latency can be a significant issue in interactive audio scenarios, where delays in the audio stream can disrupt the creative flow or cause feedback. For tasks like live voice cloning or interactive podcast recording, minimizing latency is crucial for a good user experience. Additionally, while adaptive bitrate streaming can enhance the user experience by adjusting to varying network conditions, implementing this feature within Audacity's framework might prove complex.
While Audacity currently lacks a fully integrated streaming solution, the potential for future development is compelling. Perhaps a deeper integration with existing streaming protocols or the creation of custom solutions might address these limitations. If Audacity were to adopt these advanced features, it could provide a compelling and more accessible platform for audio professionals across diverse fields, including voice cloning and audiobook production, without needing specialized or potentially more expensive DAWs. The ability to stream directly to platforms like YouTube or Spotify from within Audacity could streamline the workflow significantly, offering a complete production-to-distribution pipeline. This would make Audacity an even more formidable option for individuals who want a powerful and free audio editing tool. However, the road to a seamless streaming experience in Audacity is still under development, and it's unclear if or when it will become a core functionality.
7 Hidden Features in Audacity for Professional Voice Recording That Producers Often Overlook - Custom Voice Print Recording With Spectral Frequency Mapping
Audacity's spectral frequency mapping tools offer a unique way to work with audio, especially for voice-related tasks like cloning and sound design. By using the Spectrogram and Plot Spectrum, producers can get a visual representation of the different frequencies within a recording. This visual approach helps in understanding how vocal qualities are formed and allows for precise control over specific frequencies within a selected time range. This level of control is beneficial for cleaning up recordings, enhancing certain aspects of a voice, and tailoring a unique vocal imprint. This becomes incredibly valuable in scenarios such as audiobook production, podcasting, and voice training. While this offers exciting possibilities, especially for creating a custom voice print, it's important to acknowledge the learning curve associated with these features. Furthermore, the initial audio recording quality plays a crucial role in achieving the desired results. If the recording is poor, then the final output will likely be limited. The quality of the spectral analysis depends on the clarity of the original recording.
Each person's voice carries a unique spectral fingerprint, shaped by their vocal tract, resonance, and individual harmonics. This unique frequency map is becoming increasingly important in voice cloning technologies, as it allows for more precise replication. By capturing and mapping these frequencies, producers can create synthetic voices that sound remarkably realistic and recognizable, enhancing the potential of voice cloning.
Employing spectral frequency mapping to create custom voice prints can greatly enhance the accuracy of voice cloning. By carefully mapping the specific frequencies associated with a given voice, producers gain a precise representation that guides the cloning process. This meticulous approach ensures a more authentic and consistent sonic output, which is especially relevant in the production of audiobooks and podcasts, where maintaining consistent voice character is critical.
Techniques like phase cancellation, which leverage the nature of sound waves, become valuable for isolating specific frequency ranges. This allows us to filter out unwanted noises and enhance the clarity of the desired voice, a vital skill when editing complex audio recordings.
The field of spectral analysis is experiencing a resurgence thanks to the rise of machine learning. Algorithms are trained on massive audio datasets, enabling them to discern subtle differences in voice patterns with ever-increasing precision. This refinement in voice separation and cloning is crucial, particularly when dealing with recordings where multiple voices are present, often overlapping and intertwined.
Recent advances in spectral analysis and visualization have led to tools capable of real-time voice manipulation. This is a huge development that has exciting implications for live performance and recording. Imagine being able to adjust a singer's vocal tone or add effects in real-time during a performance, previously an impossibility.
The detailed spectral map produced via spectral analysis doesn't just reveal the fundamental frequency of a voice but also shows the associated harmonics and overtones. This provides crucial insights into the timbre of a voice. Understanding the relationship between these components enables producers to optimize audio settings for specific voices and mixed audio environments, thereby improving vocal clarity.
One of the most fascinating aspects of spectral analysis lies in its ability to reconstruct lost or damaged audio segments. By analyzing the remaining frequency information, it's possible to estimate the missing parts of the signal, potentially saving a recording deemed unusable. This capability has immense implications for salvaging recordings that might otherwise be discarded.
The accuracy and detail of spectral mapping are significantly affected by the sampling rate used during recording. A higher sampling rate captures a more comprehensive frequency profile, leading to sharper, more defined spectral maps. This is crucial for advanced voice manipulation techniques like cloning and alteration.
Spectral analysis' principles have applications beyond voice cloning. These tools are now also being utilized in the fields of music production, sound design, and noise reduction. By applying the same principles and methodologies, producers can identify and address specific sound issues across a wider range of audio formats and genres.
Despite all the improvements, achieving flawless voice isolation remains challenging. Factors like overlapping vocal frequencies and background noise often create limitations. Understanding the limitations and the complexities that influence the outcome is crucial for producers to manage expectations and continue pushing innovation in this field. The future of voice manipulation and cloning relies on continued advancement in spectral mapping and analysis techniques to continually refine these capabilities.
7 Hidden Features in Audacity for Professional Voice Recording That Producers Often Overlook - Smart Background Noise Reduction With Voice Recognition
Audacity's "Smart Background Noise Reduction With Voice Recognition" offers a significant advantage for audio producers, especially those working on projects like audiobooks and podcasts where pristine vocal recordings are paramount. This feature represents a more sophisticated approach to noise reduction, enabling Audacity to differentiate between the desired voice and undesirable background sounds more effectively. It essentially enhances the overall audio clarity by intelligently filtering out unwanted noises. Producers benefit by gaining greater control over background noise removal, which leads to more polished and professional recordings.
The process still involves selecting a representative noise profile, a crucial step for the software to understand what sounds to minimize. However, the "smart" aspect suggests a more refined analysis of the audio, allowing for better identification and reduction of different types of background noise. While this feature is very helpful, producers need to realize it's not a magic bullet. Fine-tuning the noise reduction settings often requires experimentation and a degree of artistry to achieve the perfect balance between clean audio and preserving the nuances of the voice recording. Essentially, the feature helps improve audio quality and allows for more precise manipulation of sound elements during voice production. This further underlines the importance of a skilled audio engineer who understands the subtle elements of sound for achieving top-tier voice recordings.
Audacity's built-in Noise Reduction effect provides a valuable tool for cleaning up audio recordings by targeting and minimizing unwanted background noise. The process begins with isolating a section of the recording that represents only the background noise and using the "Get Noise Profile" function. This allows Audacity to analyze and create a unique profile of the noise characteristics present in the recording. Once this noise profile is generated, users can apply the Noise Reduction effect to the entire recording, effectively removing the identified background noise.
The beauty of Audacity's Noise Reduction feature is its flexibility. Users can fine-tune the effect using a series of adjustable sliders, allowing for iterative adjustments and experimentation. The "Preview" button serves as a critical tool during this fine-tuning, providing a quick listen to the changes made by the adjustments. This iterative process is, in a way, more art than science, requiring some patience and experimentation to achieve the desired level of noise reduction and audio clarity.
While Audacity excels at reducing consistently present background sounds like hums, hisses, and steady whistles, it's crucial to acknowledge that the effectiveness of this tool is closely tied to the quality of the initial noise sample. Precisely choosing and isolating a representative sample of the background noise is essential for the Noise Reduction effect to accurately recognize and eliminate it from the recording. The algorithm leverages this noise profile to separate the unwanted sounds from the desired audio content.
The use of the "Effect > Noise Reduction" pathway is how users access and activate this crucial tool within Audacity. This feature enhances the quality of the final audio output, particularly for voice recordings used in productions like audiobooks, voiceovers, and podcasts. These recordings often require a clean audio environment, and Audacity’s ability to eliminate consistent background noises serves as a significant advantage for professional audio production.
The implementation of noise reduction within Audacity is largely based on frequency analysis. The algorithm examines the audio waveform and isolates specific frequencies corresponding to background noise. This frequency domain approach can be highly effective, especially when dealing with constant background sounds.
However, we should also acknowledge the limitations. While noise reduction features have advanced significantly, they struggle when dealing with complex and dynamic noise profiles—such as fluctuating background sounds or those overlapping with the intended audio—or when faced with recordings of low quality to begin with. It might also require a careful combination of noise reduction and other techniques, such as equalization, for more complex audio manipulation tasks. Nonetheless, the ability to achieve a cleaner sound through relatively straightforward methods like Audacity's Noise Reduction effect is extremely helpful and continues to be one of the overlooked tools for many audio engineers, perhaps due to the need for experimentation. It empowers producers to refine audio quality and achieve a higher standard of professional output for their creations.
7 Hidden Features in Audacity for Professional Voice Recording That Producers Often Overlook - Real Time Voice Pitch Correction Without Latency
The ability to correct vocal pitch in real-time without introducing noticeable delays is a significant advancement for sound production. This capability, now accessible through Audacity and its plugins, eliminates the frustrating lag often associated with pitch correction tools. This is especially helpful for situations where immediate feedback is vital, such as live voice-overs, interactive podcasting, or voice cloning.
Tools like MAutoPitch or Waves Tune RealTime offer impressive real-time pitch correction while minimizing latency. This allows for natural sounding adjustments without compromising the responsiveness of the recording process. The impact on voice cloning is particularly interesting, enabling voice actors to mimic or create a specific voice with immediate feedback. This interactive aspect fosters a smoother and more creative recording process compared to traditional post-production techniques.
While pitch correction is a powerful tool, it's not without its potential pitfalls. Improper use can easily introduce artifacts or make a voice sound unnatural or robotic. A degree of finesse and understanding of how pitch correction works is needed to avoid these issues and achieve truly seamless vocal refinement. It's crucial that producers understand and control the extent of these adjustments to preserve the naturalness of the voice, making for an enhanced and engaging final product, whether it's an audiobook, podcast, or a cloned voice.
Real-time voice pitch correction without latency is an intriguing pursuit in the world of audio production, especially for voice cloning and audiobook creation. However, achieving truly latency-free correction in practical applications can be a challenge due to the processing demands of the algorithms involved. While various algorithms like those found in Autotune and Melodyne aim to correct pitch while retaining the natural sound, the complexity of human speech can be a hurdle.
Human vocalizations are multifaceted, influenced by factors such as formants – the resonant frequencies of the vocal tract – that add layers of complexity to the pitch correction process. Maintaining a natural vocal quality during correction while avoiding unwanted artifacts is a tricky balancing act. Furthermore, relying heavily on pitch correction tools in extended recording sessions could potentially lead to vocal fatigue for performers, negatively affecting audio quality and requiring more correction work later.
The prospect of real-time pitch correction in live settings introduces feedback loop considerations. The ability to monitor corrections immediately is crucial, but the act of feeding the corrected audio back into the system can cause distortions or glitches, potentially disrupting a performer's flow and impacting the acoustic feedback they receive. It's a complex interplay of engineering and human perception.
As we manipulate pitch in real-time, the Doppler effect, which describes how sound changes as a source moves, can also come into play. Rapid shifts in pitch can lead to alterations in the perceived loudness and clarity of the sound, especially in mobile environments where the sound source is in motion. This impact on sound perception becomes vital when considering the context of audio production and consumption.
Interestingly, advancements in audio interfaces incorporating dedicated DSP chips are helping mitigate latency. These specialized processors allow for more efficient handling of pitch correction, leading to significantly lower delays. This improvement benefits producers who rely on instant feedback during live recordings or performances, particularly in scenarios like voice cloning where precise, immediate responses are essential.
The growing role of machine learning in audio processing also presents exciting opportunities. Machine learning algorithms can be trained to adapt and refine real-time pitch correction based on user feedback and preferences. These systems hold the potential to improve the accuracy and naturalness of pitch correction over time. However, these sophisticated systems require extensive training data and powerful computational resources to function optimally.
One interesting feature of real-time pitch correction is the ability to modify a vocal range. Beyond simply fixing off-key notes, producers can alter the overall range of a singer's voice, expanding their musical possibilities. While this is a valuable tool, it's important to note that excessive manipulation might cause vocal strain, emphasizing the need for careful use.
Ultimately, when we attempt to correct pitch while keeping the natural harmonic characteristics of a voice intact, we encounter a balancing act. There's a risk that excessive correction can strip the voice of its emotional depth and warmth, leaving it sounding sterile and artificial. Finding that sweet spot between accurate correction and preserving a natural vocal timbre is paramount for producing captivating vocal performances. This constant balancing act is a testament to the complexity and subtlety of both the human voice and the fascinating world of audio technology.
7 Hidden Features in Audacity for Professional Voice Recording That Producers Often Overlook - Voice Duplication Through Advanced Phase Alignment
"Voice Duplication Through Advanced Phase Alignment" taps into the increasing need for creating high-fidelity voice clones within audio production. The process leverages phase alignment techniques to achieve more accurate voice replication, contributing to a cleaner and more authentic sound. This involves a technique using a figure-8 microphone and subsequently inverting the phase on a copied audio track. This method can be particularly useful when layering voices for podcasts, audiobooks, or other projects where a richer vocal experience is desired. While manual alignment of audio clips using tools within Audacity is possible, understanding phase relationships is paramount. Failing to do so can lead to unwanted frequency cancellations and reduce the quality of the stacked voices. As voice cloning technologies continue to evolve, understanding these subtle, yet important, aspects of audio production will become increasingly crucial for anyone seeking to achieve high-quality voice replication.
Audacity's ability to manipulate audio through advanced phase alignment offers a fascinating approach to voice duplication, particularly valuable in audiobook production and voice cloning. The core principle relies on precisely aligning the phases of sound waves to create a more coherent and clear audio signal. This is critical for voice cloning because it enables the replication of a voice's unique spectral fingerprint, encompassing not just the fundamental pitch but also the subtle tonal qualities and emotional nuances that give a voice its character.
The success of voice cloning hinges on this ability to precisely control phase. Even minor misalignments can lead to unwanted artifacts that detract from the overall quality. Mastering this technique is essential for creating realistic and believable character voices in audiobooks, where authenticity plays a crucial role in the listener’s experience. Furthermore, by analyzing the interactions between different frequencies, engineers can utilize these techniques to extract specific voices from complex mixes with overlapping audio elements. This skill becomes vital in podcast production or audiobooks where background noise or overlapping voices can hinder clarity.
These advanced phase alignment features also benefit dynamic real-time manipulation of vocal characteristics. Producers can adjust elements of a voice during a recording, potentially enhancing the experience of voice actors or other performers working on audio projects. However, the challenge remains in implementing dynamic adjustments without introducing noticeable lag or artifacts. The ever-evolving field of machine learning continues to improve the algorithms used for voice separation and duplication. By processing massive amounts of audio data, machine learning algorithms are learning to more accurately isolate and clone voices, even in complex acoustic environments. This holds great promise for future developments in voice cloning technologies.
However, phase alignment encounters challenges when confronted with closely spaced vocal frequencies or complex overlapping speech. This often results in inaccuracies or confusion during voice separation or cloning, highlighting a persistent obstacle for audio producers in generating high-quality audio. The accuracy of voice duplication is also closely related to the quality of the source audio. Higher sampling rates capture a more detailed frequency profile, improving the resolution of the spectral fingerprint, resulting in a more precise and accurate duplication of vocal characteristics. In essence, the sampling rate dictates the level of detail available for manipulating and replicating the voice.
Beyond just voice cloning, these phase manipulation techniques find applications in the reconstruction of lost audio segments. By analyzing remaining frequency data, the algorithms can estimate missing parts of a recording, a valuable capability in rescuing potentially damaged audio files. Phase alignment also provides tools for characterizing voices through the analysis of formant interactions. This allows for a more nuanced control over a voice's features, helping in creating distinctive vocal identities for characters in various multimedia productions. Interestingly, these same techniques also find applications within the music world, enhancing harmonization and shaping the overall sonic landscape in various music styles.
The world of voice cloning and audio manipulation is constantly evolving. While advancements in phase alignment provide increasingly precise control over sound, there are inherent limitations. Understanding these limitations and the complexities involved in audio manipulation is key for audio producers and engineers to manage expectations and continue refining the technologies that contribute to shaping the future of sound.
Get amazing AI audio voiceovers made for long-form content such as podcasts, presentations and social media. (Get started for free)
More Posts from clonemyvoice.io: